Time trends and regional variation in utilization of antidiabetic medicines in China, 2015-2022
DIABETES OBESITY & METABOLISM(2024)
摘要
Aim To assess the use of non-insulin antidiabetic medicines in China. Materials and Methods We analysed the national procurement data for 29 non-insulin antidiabetic medicines from nine subgroups in China from 2015 to 2022. We estimated the number of defined daily doses (DDDs) procured per year in seven regions of China for nine subgroups and adjusted the data by the number of patients with diabetes. For each subgroup, the regional ratio was calculated by comparing the procurement per patient in each region with the procurement nationwide. The regional disparity was the difference between the highest and lowest regional ratios. We compared the medication patterns across regions. Results Nationally, between 2015 and 2022, the number of DDDs per patient increased from 14.45 to 47.37. The two most commonly used categories were sulphonylurea and biguanides, which increased from 7.04 to 15.39 (119%) and 3.28 to 11.11 (239%) DDDs per patient, respectively. The procurement of new drugs (dipeptidyl peptidase-4 inhibitors, sodium-glucose cotransporter type 2 inhibitors and glucagon-like peptide-1 receptor agonists) increased quickly and had >5000% relative changes. Particularly for sodium-glucose cotransporter type 2 inhibitors, it increased from 0.08 to 5.03 DDDs (6662%). The southwest region had the highest relative change (319%), while the southern region had the lowest (118%). Biguanide and thiazolidinediones had the lowest (1.19) and highest level (2.21) of regional disparity in 2022, respectively. Conclusion The procurement of non-insulin antidiabetic medicines in China has increased a lot from 2015 to 2022. In terms of DDDs per patient, sulphonylurea ranked first, followed by metformin. The procurement of new drugs increased greatly. A large regional disparity existed in medicine usage and patterns.
更多查看译文
关键词
antidiabetic medicines,China,prescribing pattern,regional variation,time trends
AI 理解论文
溯源树
样例
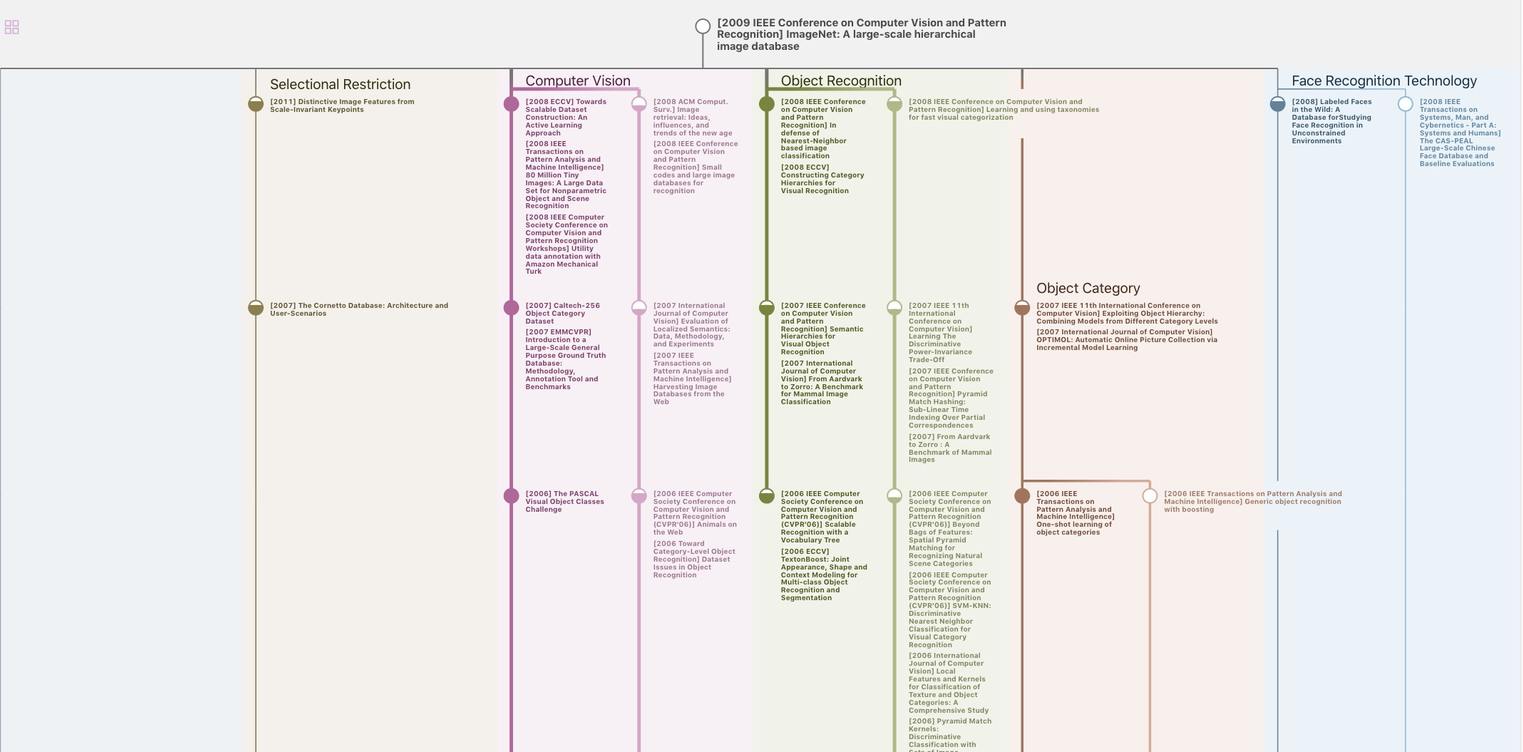
生成溯源树,研究论文发展脉络
Chat Paper
正在生成论文摘要