A Multi-sensor Approach Integrating Cyclostationary Analysis and Evidence Theory for Explainable Bearing Fault Diagnosis
IEEE Sensors Journal(2024)
摘要
Rolling element bearing diagnostics has long been a crucial and challenging problem in industrial applications due to the presence of heavy and intricate noise. Demodulation techniques excel in revealing faulty modulation characteristics in rolling element bearings, offering a strongly explainable result compared with other intelligent algorithms. However, the diagnostic process heavily relies on manual judgment, often leading to potential misdiagnoses and false alarms. Besides, single-sensor diagnostics may fail to achieve high accuracy due to the uncertainty of information. Thus, this paper proposes a multi-sensor fault diagnosis method based on cyclostationary analysis and evidence theory for the explainable diagnosis of rolling element bearings. First, the feature vector containing bearing fault characteristics is constructed by cyclostationary analysis. Second, the Mahalanobis distance between training data and test data is utilized to generate the basic probability assignment (BPA) of Dempster-Shafer (D-S) evidence theory. Third, to deal with the conflicting information, weight factors are assigned to the original BPAs based on the Mahalanobis distance as well. Last, the diagnosis result is obtained by fusing the BPAs of multiple sensors via Dempster’s combination rule (DCR). Through the validation by experiments, the superiority of the proposed method is demonstrated in comparison with other state-of-the-art methods based on evidence theory. The proposed method can identify the fault type and fault severity of the bearing with 97.84% average accuracy. Furthermore, it exhibits excellent generalization ability, high accuracy with small sample sizes, and strong noise resistance.
更多查看译文
关键词
Cyclostationary analysis,evidence theory,fault diagnosis,information fusion,rolling element bearing
AI 理解论文
溯源树
样例
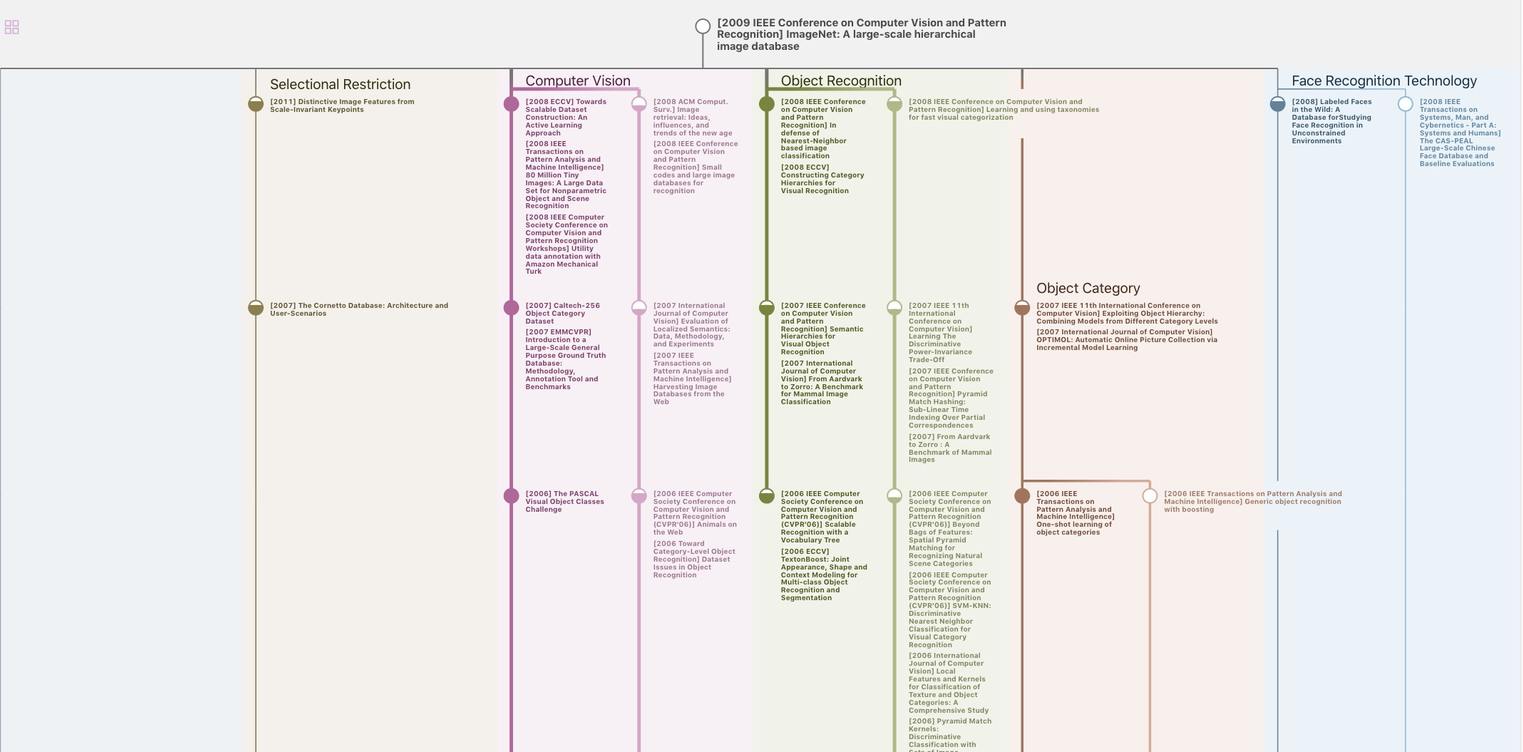
生成溯源树,研究论文发展脉络
Chat Paper
正在生成论文摘要