Fast Fishing: Approximating BAIT for Efficient and Scalable Deep Active Image Classification
Lecture Notes in Computer Science Machine Learning and Knowledge Discovery in Databases Research Track(2024)
摘要
Deep active learning (AL) seeks to minimize the annotation costs for training deep neural networks. BAIT, a recently proposed AL strategy based on the Fisher Information, has demonstrated impressive performance across various datasets. However, BAIT's high computational and memory requirements hinder its applicability on large-scale classification tasks, resulting in current research neglecting BAIT in their evaluation. This paper introduces two methods to enhance BAIT's computational efficiency and scalability. Notably, we significantly reduce its time complexity by approximating the Fisher Information. In particular, we adapt the original formulation by i) taking the expectation over the most probable classes, and ii) constructing a binary classification task, leading to an alternative likelihood for gradient computations. Consequently, this allows the efficient use of BAIT on large-scale datasets, including ImageNet. Our unified and comprehensive evaluation across a variety of datasets demonstrates that our approximations achieve strong performance with considerably reduced time complexity. Furthermore, we provide an extensive open-source toolbox that implements recent state-of-the-art AL strategies, available at https://github.com/dhuseljic/dal-toolbox.
更多查看译文
关键词
Active Learning,Robust Learning,Deep Learning,Representation Learning,Hyperparameter Optimization
AI 理解论文
溯源树
样例
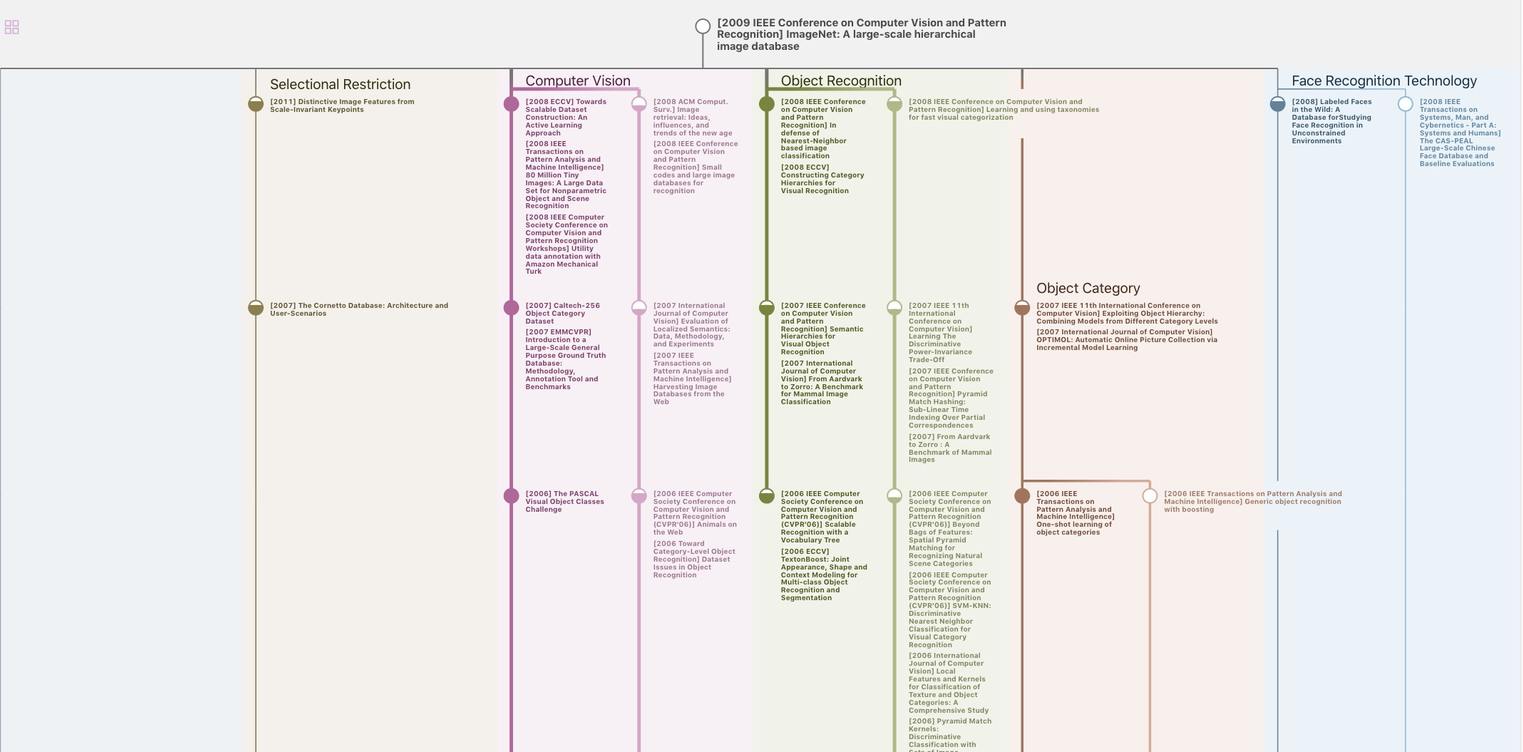
生成溯源树,研究论文发展脉络
Chat Paper
正在生成论文摘要