Tangram: High-resolution Video Analytics on Serverless Platform with SLO-aware Batching
2024 IEEE 44th International Conference on Distributed Computing Systems (ICDCS)(2024)
摘要
Cloud-edge collaborative computing paradigm is a promising solution tohigh-resolution video analytics systems. The key lies in reducing redundantdata and managing fluctuating inference workloads effectively. Previous workhas focused on extracting regions of interest (RoIs) from videos andtransmitting them to the cloud for processing. However, a naive Infrastructureas a Service (IaaS) resource configuration falls short in handling highlyfluctuating workloads, leading to violations of Service Level Objectives (SLOs)and inefficient resource utilization. Besides, these methods neglect thepotential benefits of RoIs batching to leverage parallel processing. In thiswork, we introduce Tangram, an efficient serverless cloud-edge video analyticssystem fully optimized for both communication and computation. Tangramadaptively aligns the RoIs into patches and transmits them to the scheduler inthe cloud. The system employs a unique “stitching” method to batch thepatches with various sizes from the edge cameras. Additionally, we develop anonline SLO-aware batching algorithm that judiciously determines the optimalinvoking time of the serverless function. Experiments on our prototype revealthat Tangram can reduce bandwidth consumption and computation cost up to74.30% and 66.35%, respectively, while maintaining SLO violations within 5%and the accuracy loss negligible.
更多查看译文
关键词
video analytics,batching inference,serverless computing
AI 理解论文
溯源树
样例
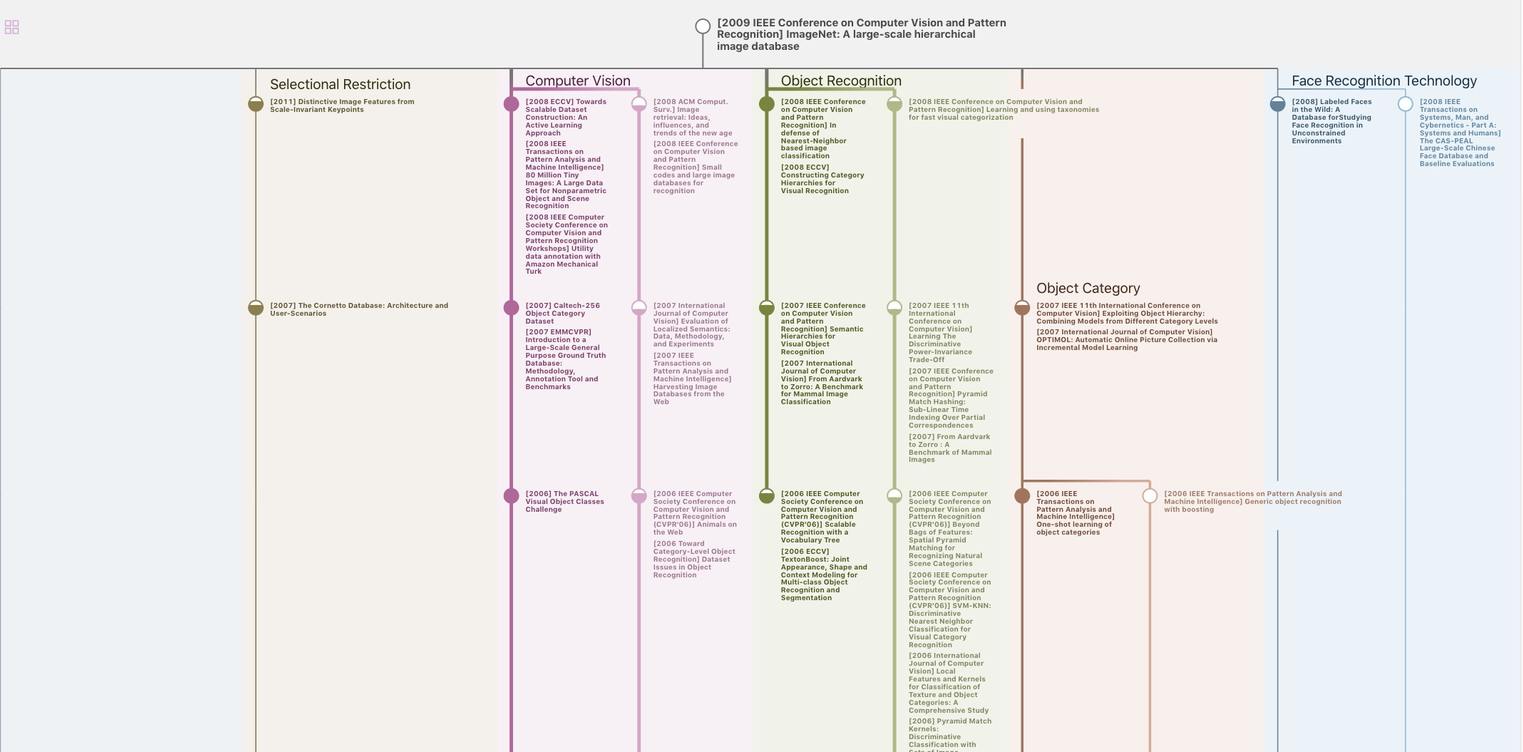
生成溯源树,研究论文发展脉络
Chat Paper
正在生成论文摘要