On the Optimal Regret of Locally Private Linear Contextual Bandit
arxiv(2024)
摘要
Contextual bandit with linear reward functions is among one of the most
extensively studied models in bandit and online learning research. Recently,
there has been increasing interest in designing locally private linear
contextual bandit algorithms, where sensitive information contained in contexts
and rewards is protected against leakage to the general public. While the
classical linear contextual bandit algorithm admits cumulative regret upper
bounds of Õ(√(T)) via multiple alternative methods, it has
remained open whether such regret bounds are attainable in the presence of
local privacy constraints, with the state-of-the-art result being Õ(T^3/4). In this paper, we show that it is indeed possible to achieve an
Õ(√(T)) regret upper bound for locally private linear contextual
bandit. Our solution relies on several new algorithmic and analytical ideas,
such as the analysis of mean absolute deviation errors and layered principal
component regression in order to achieve small mean absolute deviation errors.
更多查看译文
AI 理解论文
溯源树
样例
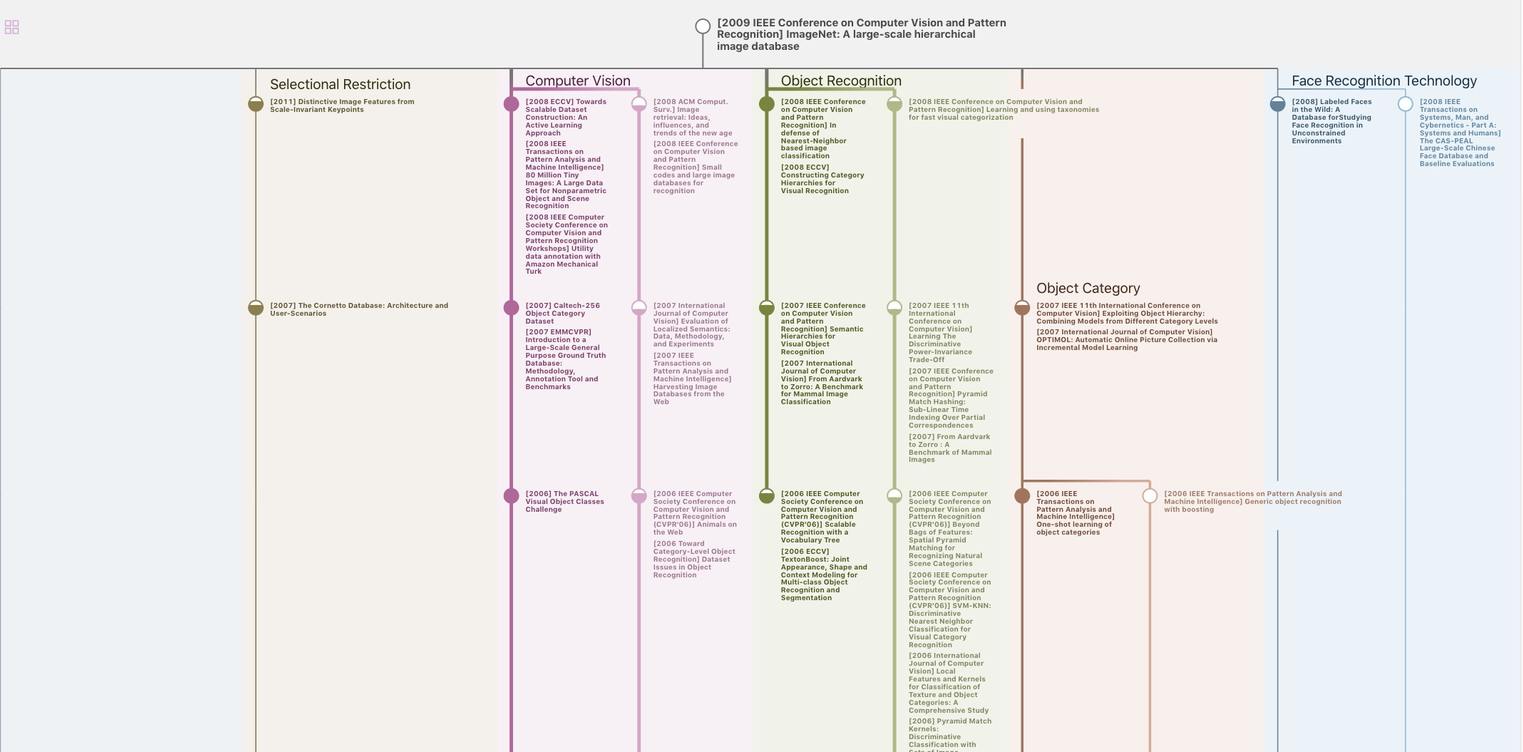
生成溯源树,研究论文发展脉络
Chat Paper
正在生成论文摘要