OSNet & MNetO: Two Types of General Reconstruction Architectures to Transform DBP Images for Linear Computed Tomography in Multi-Scenarios
IEEE Transactions on Instrumentation and Measurement(2024)
摘要
Recently, in some special situations, linear computed tomography (LCT) systems have provided better imaging solutions for internal structures compared with conventional CT instruments. To image the region of interest (ROI) and weaken projection truncation in the LCT reconstruction, the backprojection filtration (BPF) algorithm is an effective solution. However, in BPF for LCT, it is difficult to achieve stable interior reconstruction, and for differentiated backprojection (DBP) images of LCT. To satisfy multiple reconstruction scenarios for LCT, including interior ROI, complete object, and exterior region, we propose two types of reconstruction architectures. The first overlays multiple DBP images to obtain a complete DBP image, then uses a network to learn the overlying or composite Hilbert filtering function, referred to as the Overlay-Single Network (OSNet). The second uses multiple networks to train different directional Hilbert filtering models for DBP images of multiple linear scanning trajectories, respectively, and then overlays the reconstructed results, i.e., Multiple Networks Overlaying (MNetO). In two architectures, we introduce a Swin Transformer (ST) block to the generator of pix2pixGAN to extract both local and global features from DBP images at the same time. We investigate the proposed architectures from different networks, FOV sizes, pixel sizes, number of projections, geometric magnification, and computation time. The two architectures are proven to recover images. OSNet outperforms BPF in multiple scenarios. In OSNet, ST-pix2pixGAN is superior to pix2pixGAN and CycleGAN for the different networks. The average peak signal-to-noise ratio (PSNR) value reaches 41.1949 dB, which improves by 31% compared to BPF. The average structural similarity index measure (SSIM) and root-mean-square error (RMSE) are improved at 0.9996 and 0.0093, respectively. In MNetO, one model in a certain direction can increase the PSNR by about 18.4% compared to BPF, enabling high-quality imaging of the exterior edge. Our architectures can provide great solutions or references for LCT high-resolution imaging and non-destructive measuring.
更多查看译文
关键词
Linear computed tomography,differentiated backprojection,finite inversion of Hilbert transform,deep learning,swin transformer
AI 理解论文
溯源树
样例
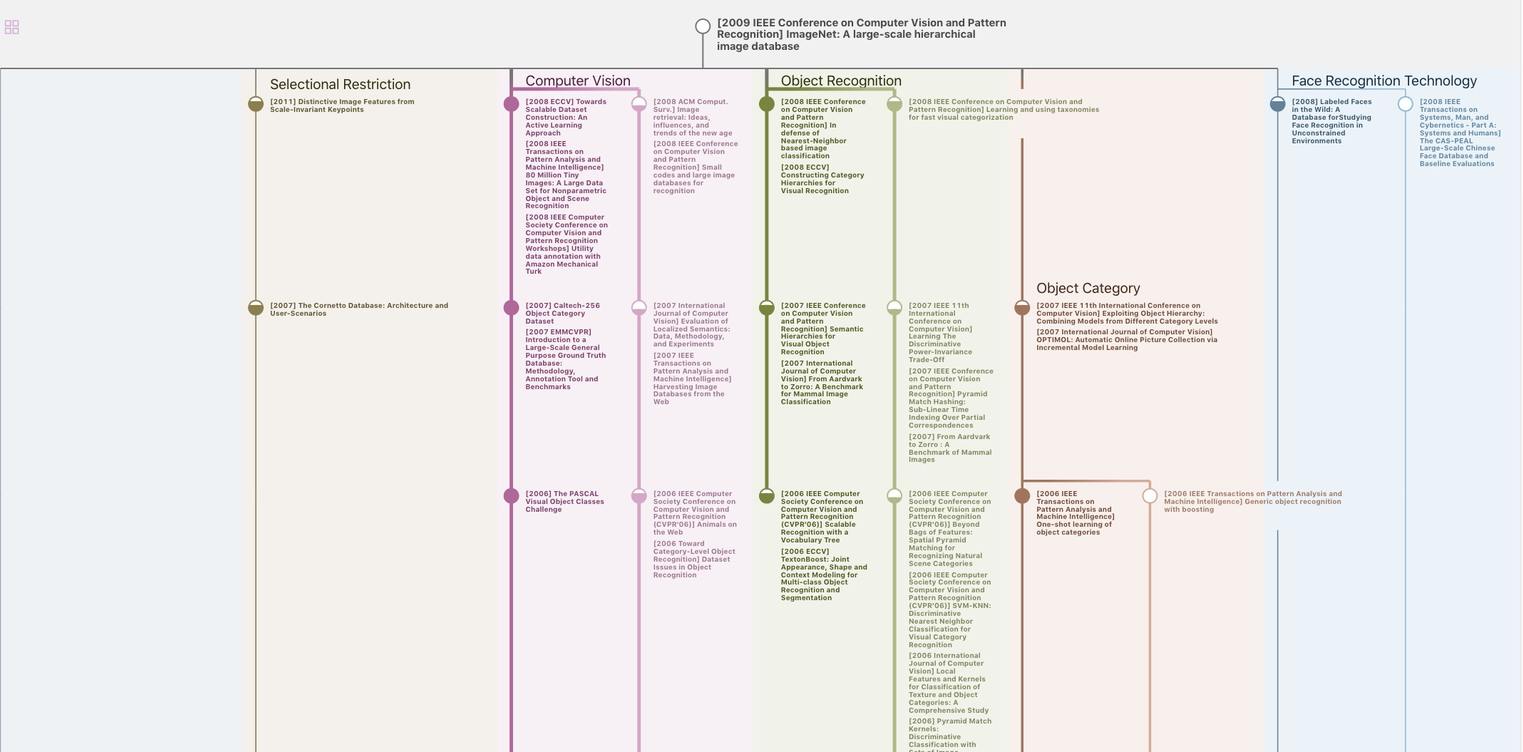
生成溯源树,研究论文发展脉络
Chat Paper
正在生成论文摘要