A spatio-temporal model to detect potential outliers in disease mapping
arxiv(2024)
摘要
Spatio-temporal disease mapping models are commonly used to estimate the
relative risk of a disease over time and across areas. For each area and time
point, the disease count is modelled with a Poisson distribution whose mean is
the product of an offset and the disease relative risk. This relative risk is
commonly decomposed in the log scale as the sum of fixed and latent effects.
The Rushworth model allows for spatio-temporal autocorrelation of the random
effects. We build on the Rushworth model to accommodate and identify
potentially outlying areas with respect to their disease relative risk
evolution, after taking into account the fixed effects. An area may display
outlying behaviour at some points in time but not all. At each time point, we
assume the latent effects to be spatially structured and include scaling
parameters in the precision matrix, to allow for heavy-tails. Two prior
specifications are considered for the scaling parameters: one where they are
independent across space and one with spatial autocorrelation. We investigate
the performance of the different prior specifications of the proposed model
through simulation studies and analyse the weekly evolution of the number of
COVID-19 cases across the 33 boroughs of Montreal and the 96 French departments
during the second wave. In Montreal, 6 boroughs are found to be potentially
outlying. In France, the model with spatially structured scaling parameters
identified 21 departments as potential outliers. We find that these departments
tend to be close to each other and within common French regions.
更多查看译文
AI 理解论文
溯源树
样例
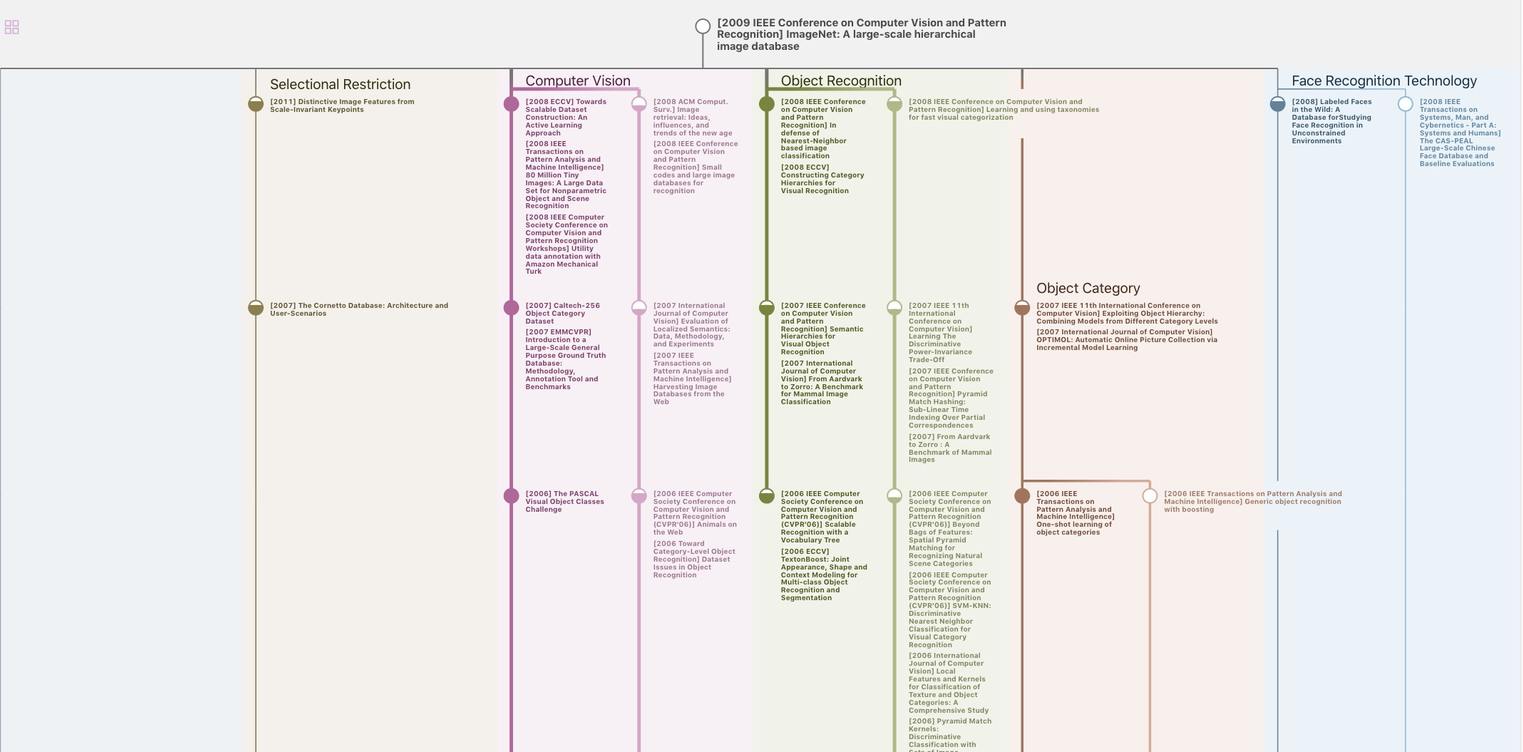
生成溯源树,研究论文发展脉络
Chat Paper
正在生成论文摘要