TFUT: Task fusion upward transformer model for multi-task learning on dense prediction
Computer Vision and Image Understanding(2024)
摘要
Transformer-based advancements have shown great promise in solving multi-task learning on dense prediction tasks. Well-designed task interaction modules of these methods further improve the performances by effectively transferring contextual information between tasks. However, many of these methods do not leverage the target task to guide contextual information from the source task. We propose the Task Fusion Upward Transformer (TFUT) model for multi-task learning on dense prediction. To facilitate task interaction, we introduce the Asymmetric Cross Task Interaction module, which utilizes asymmetric transmission in attention. During similarity calculations, the model leverages the target task to guide the expression of contextual information from the source task, ensuring effective transmission of the context information. In order to avoid the loss of detail and the discontinuity of gradient in upsampling, the Upward Transformer Decoder is designed to extract and align multi-scale features using multi-level convolution. The effectiveness of the proposed model has been demonstrated through experiments on the NYUD-v2 dataset and the PASCAL Context dataset. The experimental results show that this model has achieved optimal performance in various single task and multi-task scenarios.
更多查看译文
关键词
Vision transformer,Multi-task learning,Dense prediction
AI 理解论文
溯源树
样例
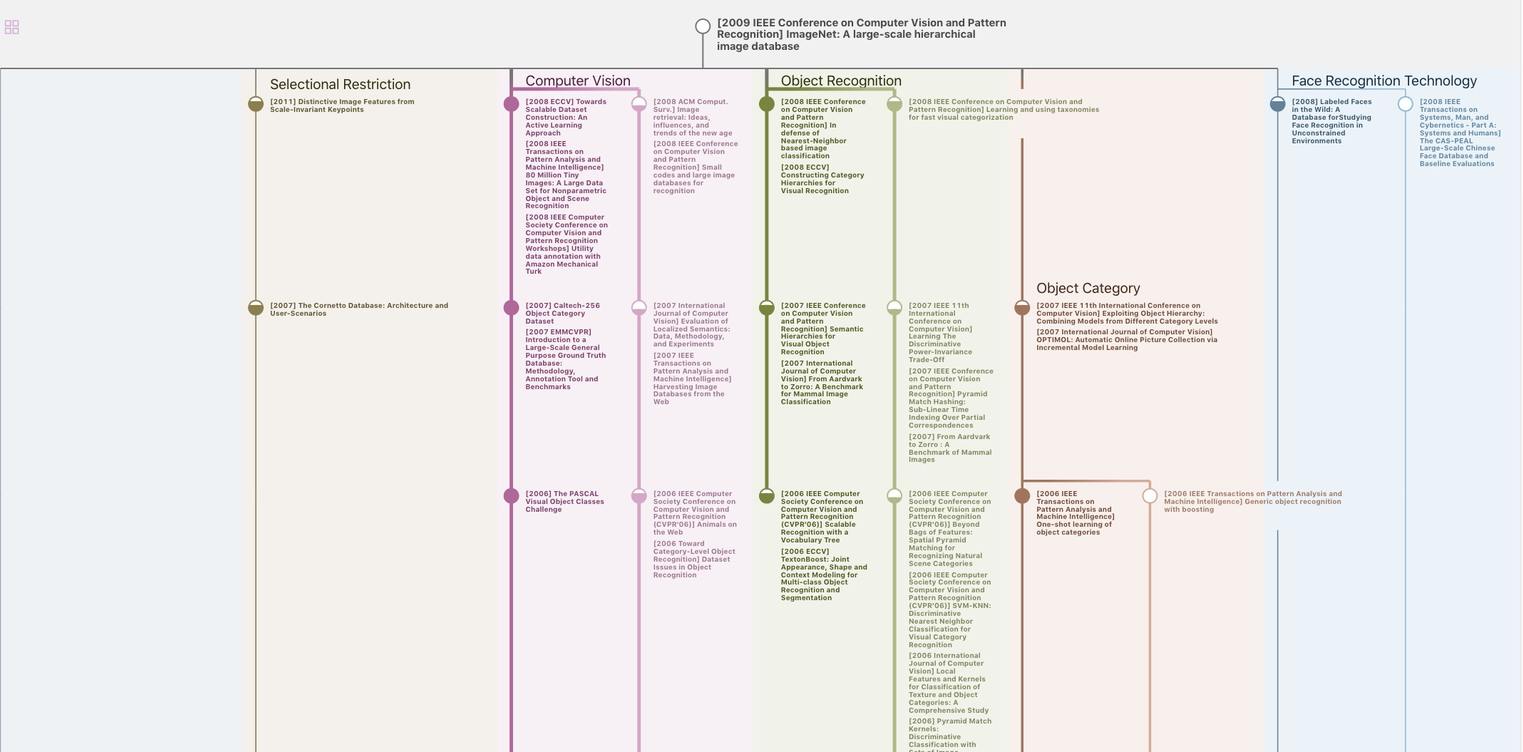
生成溯源树,研究论文发展脉络
Chat Paper
正在生成论文摘要