DeepDecon accurately estimates cancer cell fractions in bulk RNA-seq data
Patterns(2024)
摘要
Understanding the cellular composition of a disease-related tissue is important in disease diagnosis, prognosis, and downstream treatment. Recent advances in single-cell RNA-sequencing (scRNA-seq) technique have allowed the measurement of gene expression profiles for individual cells. However, scRNA-seq is still too expensive to be used for large-scale population studies, and bulk RNA-seq is still widely used in such situations. An essential challenge is to deconvolve cellular composition for bulk RNA-seq data based on scRNA-seq data. Here, we present DeepDecon, a deep neural network model that leverages single-cell gene expression information to accurately predict the fraction of cancer cells in bulk tissues. It provides a refining strategy in which the cancer cell fraction is iteratively estimated by a set of trained models. When applied to simulated and real cancer data, DeepDecon exhibits superior performance compared to existing decomposition methods in terms of accuracy.
更多查看译文
关键词
deconvolution,scRNA-seq,deep neural network,cancer tissue
AI 理解论文
溯源树
样例
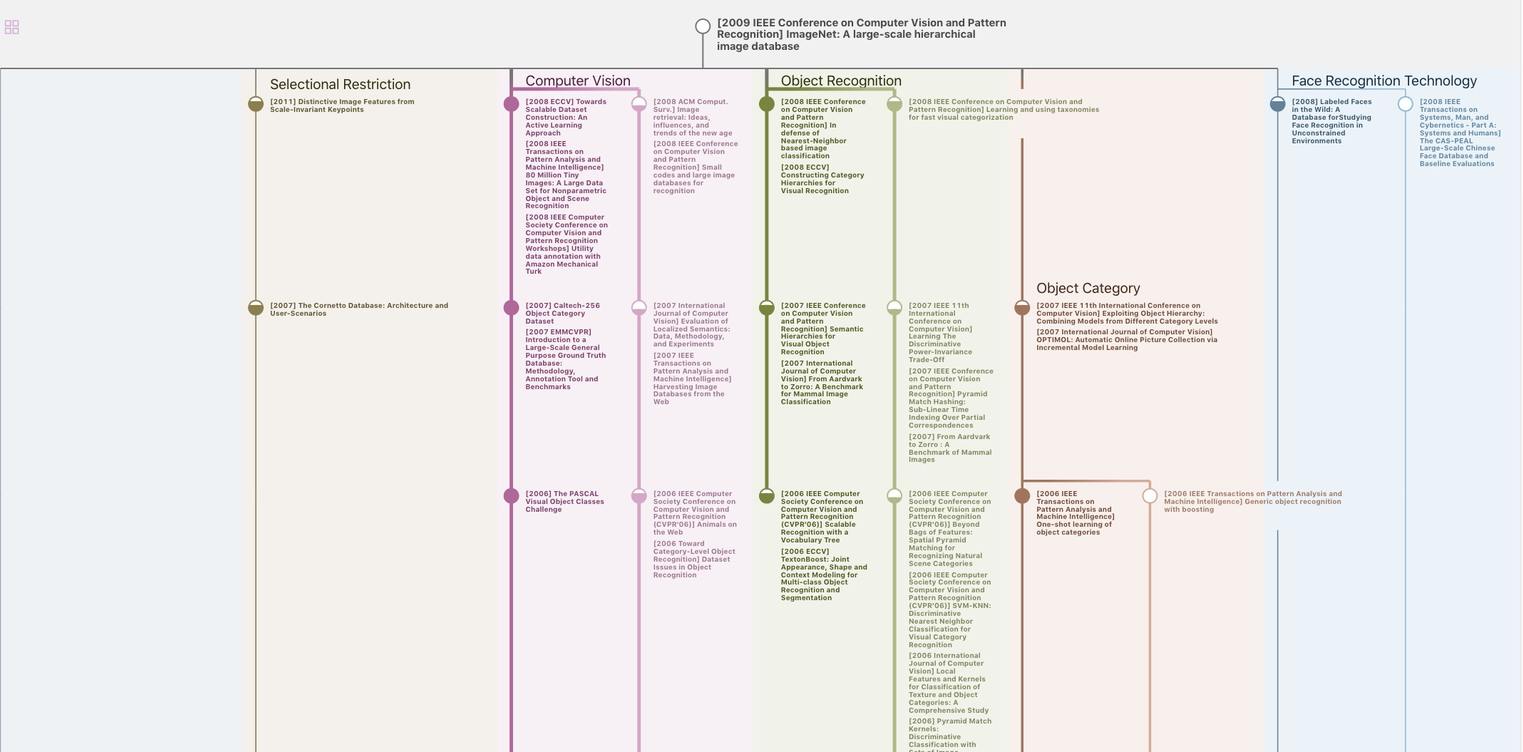
生成溯源树,研究论文发展脉络
Chat Paper
正在生成论文摘要