Machine Learning-Accelerated First-Principles Study of Atomic Configuration and Ionic Diffusion in Li10GeP2S12 Solid Electrolyte
Materials(2024)
摘要
The solid electrolyte Li10GeP2S12 (LGPS) plays a crucial role in the development of all-solid-state batteries and has been widely studied both experimentally and theoretically. The properties of solid electrolytes, such as thermodynamic stability, conductivity, band gap, and more, are closely related to their ground-state structures. However, the presence of site-disordered co-occupancy of Ge/P and defective fractional occupancy of lithium ions results in an exceptionally large number of possible atomic configurations (structures). Currently, the electrostatic energy criterion is widely used to screen favorable candidates and reduce computational costs in first-principles calculations. In this study, we employ the machine learning- and active-learning-based LAsou method, in combination with first-principles calculations, to efficiently predict the most stable configuration of LGPS as reported in the literature. Then, we investigate the diffusion properties of Li ions within the temperature range of 500–900 K using ab initio molecular dynamics. The results demonstrate that the atomic configurations with different skeletons and Li ion distributions significantly affect the Li ions’ diffusion. Moreover, the results also suggest that the LAsou method is valuable for refining experimental crystal structures, accelerating theoretical calculations, and facilitating the design of new solid electrolyte materials in the future.
更多查看译文
关键词
Li<sub>10</sub>GeP<sub>2</sub>S<sub>12</sub> solid electrolyte,first-principles calculation,Ewald-summation-based electrostatic energy,machine learning- and active-learning-based LAsou method,ab initio molecular dynamics
AI 理解论文
溯源树
样例
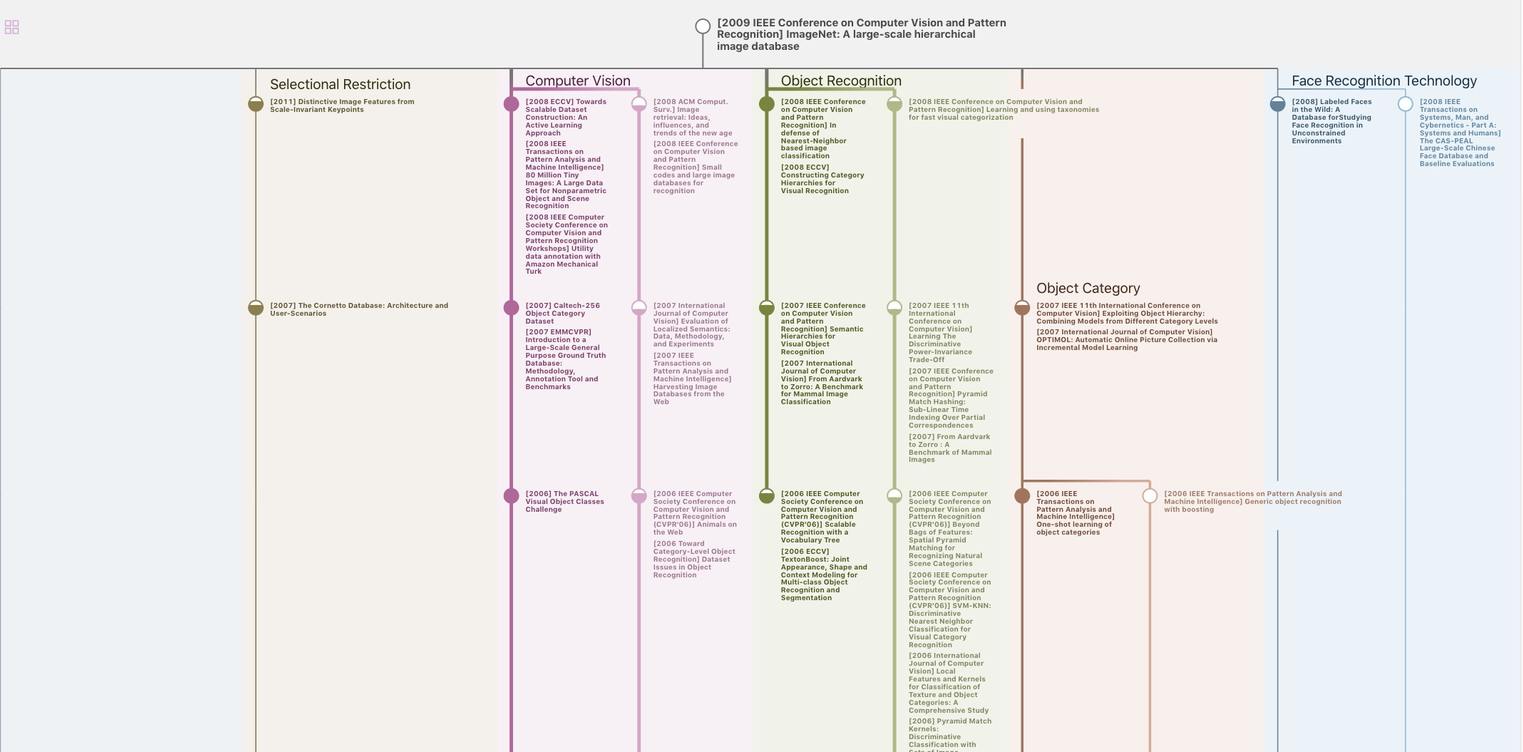
生成溯源树,研究论文发展脉络
Chat Paper
正在生成论文摘要