A Data-Driven Approach for Power IGBT Operation State Prediction Based on Domain Adapted BiLSTM Networks
2023 International Conference on Sensing, Measurement & Data Analytics in the era of Artificial Intelligence (ICSMD)(2023)
摘要
Insulated gate bipolar transistor (IGBT), as a power semiconductor element used to convert direct and alternating currents, has become a key component in the fields of new energy vehicles. IGBT is subject to a variety of faults and aging failures when operating at high loads. This work proposes a data-driven approach to predicting the RUL of IGBTs. The approach utilizes a deep learning (DL) framework based on bidirectional long short-term memory (BiLSTM) networks. This framework allows for quick processing of timing aging data and provides accurate prediction results. Domain-adversarial neural network is also applied to the LSTM network framework to reduce the distribution differences between the source domain and the target domain, and solve the problem of predicting the operation state of IGBT under unknown modules. To evaluate the performance of our proposed approach, we utilized the NASA Ames Laboratory Prognostics Center of Excellence IGBTs accelerated aging database. Experimental results demonstrate that our method can provide correct predictions during the early stages of IGBT degradation, even under unknown operating conditions. Furthermore, the method updates the prediction results throughout the aging process.
更多查看译文
关键词
IGBT power modules,Operation state prediction,Channel self-attention mechanism,Domain adaptation,Long short-term memory networks
AI 理解论文
溯源树
样例
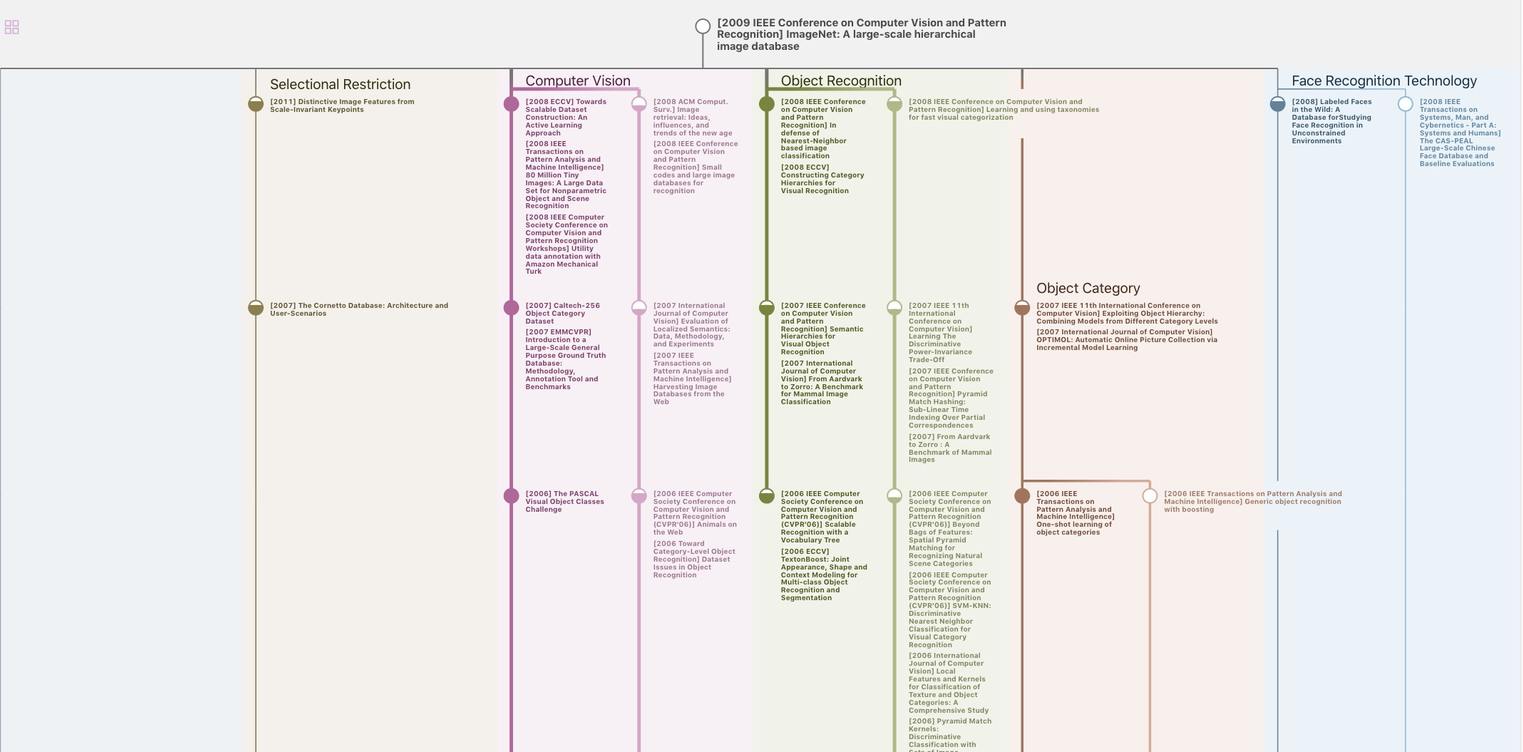
生成溯源树,研究论文发展脉络
Chat Paper
正在生成论文摘要