Predicting Thermal Transport Properties in Phononic Crystals Via Machine Learning
Applied physics letters(2024)
摘要
Although anisotropic phononic crystals (PnCs) could be utilized to control the phonon dispersions and thermal transports, rapidly discovering their properties presents a significant challenge due to the enormous consumption of traditional computational methods. In this study, we have developed machine learning techniques to forecast the thermal conductance of anisotropic PnCs (GPnC and GPnC/Gmem) based on the elastic constants, taking conventional inorganic and halide perovskites as examples for their thermoelectric applications. Our findings suggest that predicting GPnC/Gmem is more challenging than predicting GPnC attribute to the complex influence factors and spatial distribution patterns of the former. The GPnC and GPnC/Gmem of the weakest thermal anisotropic materials—all hexagonals are invariants in the (0 0 1) plane, because the velocities in this plane are direction-independent. The GPnC and GPnC/Gmem of the strongest thermal anisotropic material FAPbI3 reaches the minimum and maximum values in [1 1 0] and [1 0 0] directions, respectively. Ultimately, our machine learning models can map the hidden complex nonlinear relationships between target thermal properties and mechanical features to provide valuable insight for accurate and efficient prediction and analysis of the thermal behaviors of PnCs at a mesoscopic level under low temperatures.
更多查看译文
AI 理解论文
溯源树
样例
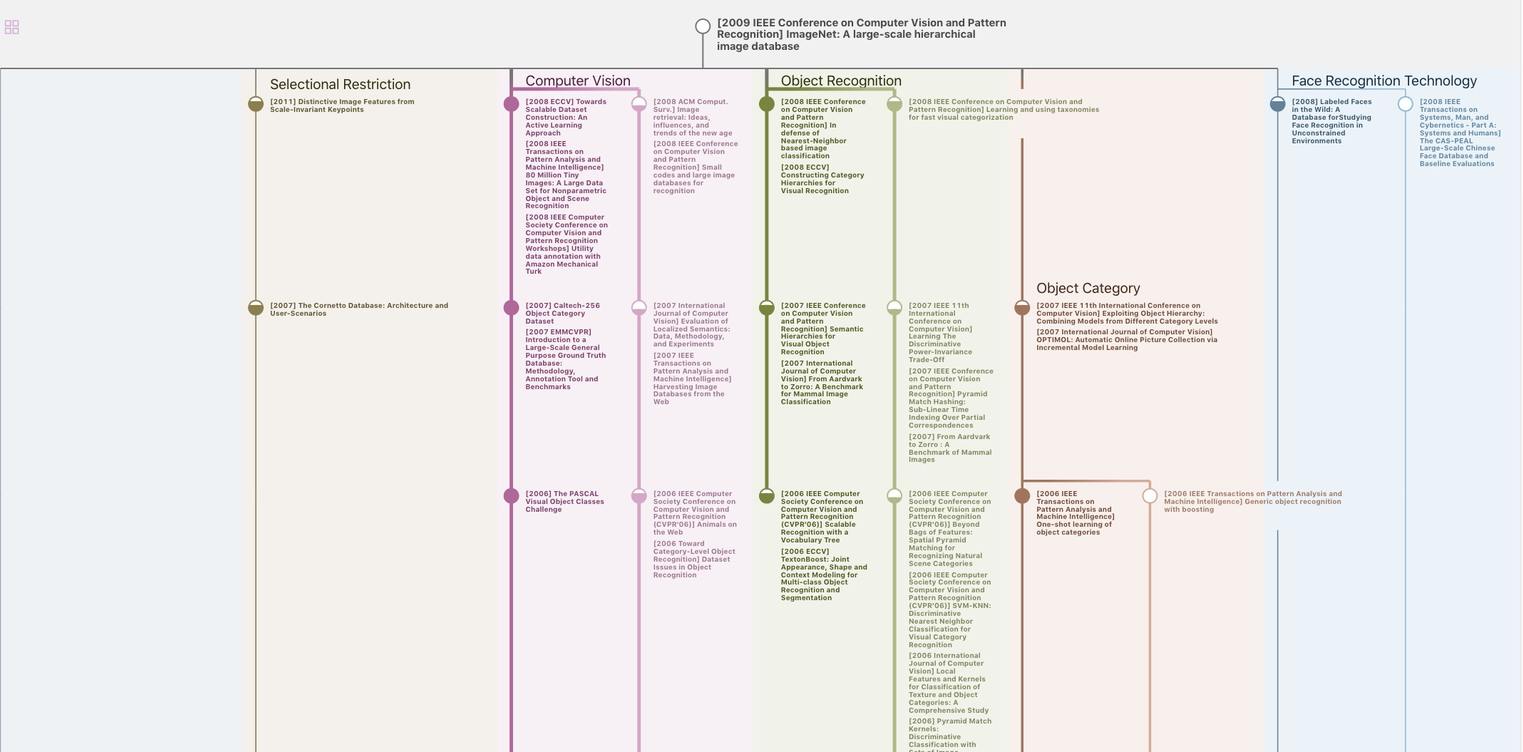
生成溯源树,研究论文发展脉络
Chat Paper
正在生成论文摘要