Functionality and Data Stealing by Pseudo-Client Attack and Target Defenses in Split Learning
IEEE Transactions on Dependable and Secure Computing(2024)
Abstract
Split learning (SL) aims to protect a client's data by splitting up a neural network among the client and the server. Previous efforts have shown that a semi-honest server can conduct a model inversion attack. However, those attacks require the knowledge of the client network structure, and the performance deteriorates dramatically as the client network gets deeper (
$\geq 2$
layers). In this work, we explore the attack in a more general and challenging situation where the client model is unknown and more complex. We unveil the inherent privacy leakage through a series of intermediate server models during SL, and propose a new attack on SL:
P
seudo-
C
lient
AT
tack (PCAT). To the best of our knowledge, this is the first attack for a semi-honest server to steal clients' functionality, reconstruct private inputs and labels without any knowledge about the clients' network structure. Moreover, the attack is transparent to clients. Extensive experiments demonstrate that our attack outperforms previous works in scenarios involving more complex models and learning tasks, even in non-i.i.d. settings and confronted with conventional defensive measures. We further explore novel defense mechanisms to mitigate PCAT and improve our attack to counteract the potential defenses.
MoreTranslated text
Key words
privacy attacks,privacy defenses,split learning
AI Read Science
Must-Reading Tree
Example
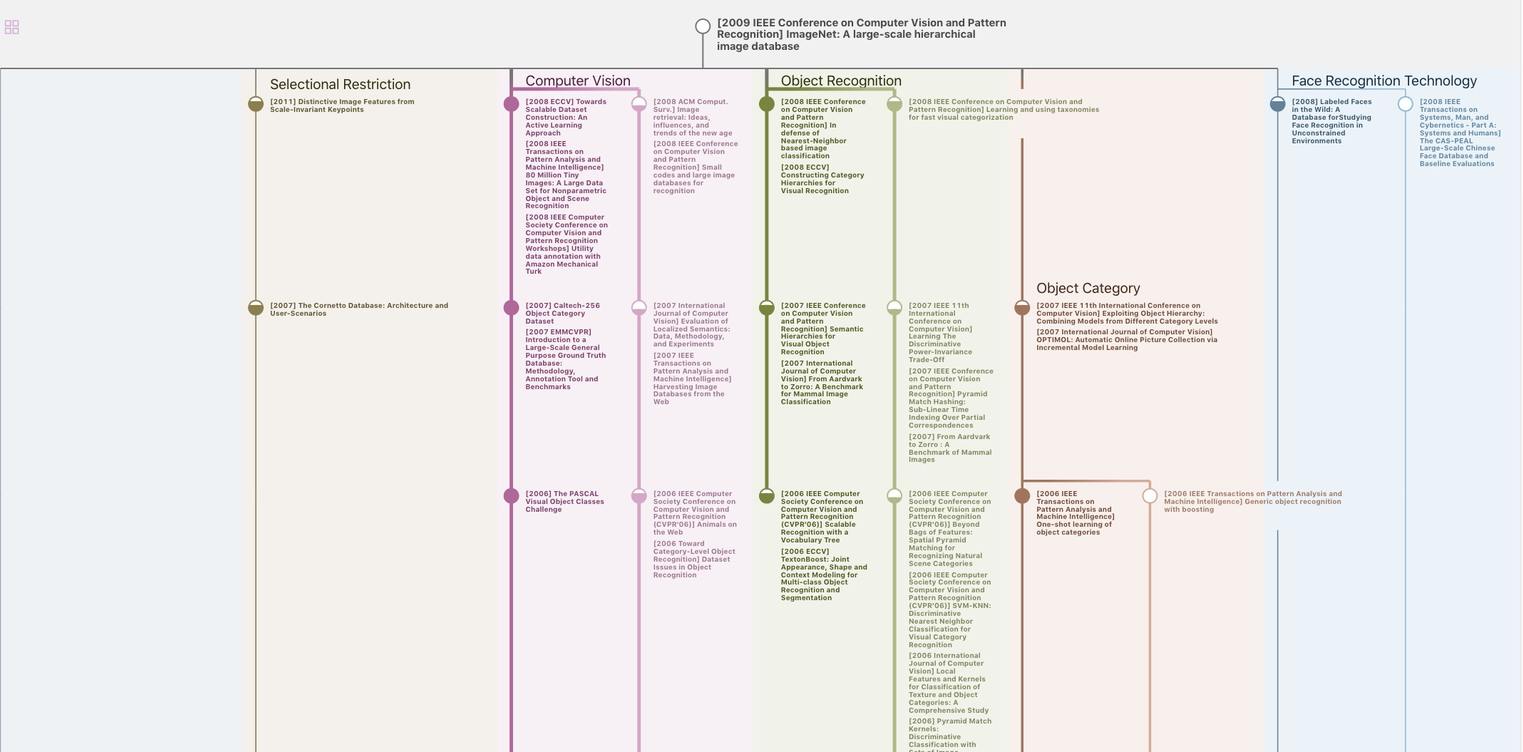
Generate MRT to find the research sequence of this paper
Chat Paper
Summary is being generated by the instructions you defined