DCIM-GCN: Digital Computing-in-Memory Accelerator for Graph Convolutional Network
IEEE TRANSACTIONS ON CIRCUITS AND SYSTEMS I-REGULAR PAPERS(2024)
摘要
Graph convolutional network (GCN) has gained great success in a diverse range of intelligent tasks. However, the hardware performance of GCNs is often bounded by random and non-continuous memory accesses due to the sparse graph data, which incur high latency and high power consumption. The emerging computing-in-memory (CIM) architecture significantly reduces the overhead of data movements, which is suitable for memory-intensive GCN acceleration. Existing analog-based CIM solutions require a large amount of analog-to-digital (AD) and digital-to-analog (DA) conversions, which dominate the overall area and power consumption. Furthermore, the analog non-ideality can degrade accuracy and reliability of CIM. To address these challenges, this work proposes a digital CIM accelerator based on SRAM, called DCIM-GCN, to accelerate GCN algorithm. DCIM-GCN introduces innovations on three levels: circuit, architecture, and algorithm. At the circuit level, digital CIM is proposed with SRAM sub-arrays to eliminate the power and area expensive AD/DA converters. Furthermore, we have incorporated the multi-address feature into the digital CIM, thereby leveraging its ability to efficiently process sparse matrix multiplication. At the architecture level, the sparsity-aware computation engine takes advantage of sparsity in GCNs and leverages CIM to minimize memory accesses and data movements. Finally, at the algorithm level, the balance mapping algorithm tackles workload imbalance issues, while the vertex reorder algorithm reduces idle states for aggregation engines, resulting in increased hardware utilization. Our DCIM-GCN achieves 1.89 $\times$ and 2.42 $\times$ speedup and 4.58 $\times$ and 9.46 $\times$ energy efficiency improvement on average over other CIM-based graph accelerators, e.g., PASGCN and PIM-GCN, respectively.
更多查看译文
关键词
Graph neural networks,computing-in-memory,domain-specific accelerator
AI 理解论文
溯源树
样例
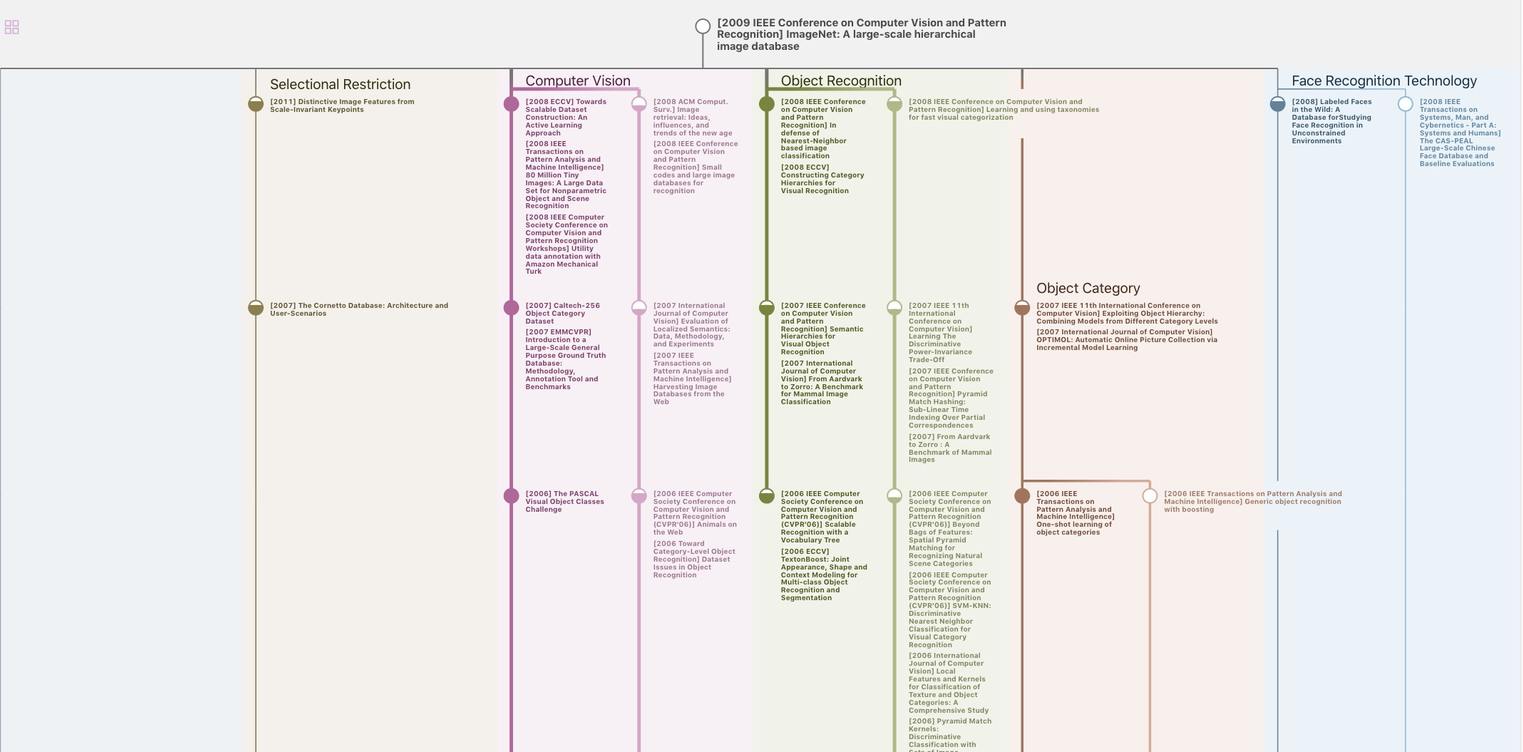
生成溯源树,研究论文发展脉络
Chat Paper
正在生成论文摘要