Hyperspectral Anomaly Detection via MERA Decomposition and Enhanced Total Variation Regularization.
IEEE Trans. Geosci. Remote. Sens.(2024)
摘要
In recent years, tensor representation (TR) based hyperspectral anomaly detection approaches have attracted more and more attention. However, two urgent issues still need to be addressed: 1) existing tensor decomposition approaches for hyperspectral anomaly detection (HAD) cannot make full use of the spectral-spatial correlation of background components in hyperspectral images (HSIs); 2) most approaches based on TR overlook the piecewise-smooth of background components that exist simultaneously in the spectral and spatial domains. To this end, with the aid of an advanced multi-scale entanglement renormalization ansatz (MERA) tensor network, this paper proposes an algorithm based on MERA decomposition and enhanced total variation regularization (MERAETV) for HAD. Specifically, MERA decomposes the background tensor by contracting a top-level factor with the remaining semi-orthogonal and orthogonal factors. Due to the intricate interplay between semi-orthogonal (low-rank) and orthogonal factors, low-rank MERA approximation exhibits a robust representational capacity that effectively captures the spectral-spatial correlation of the background component. Meanwhile, an enhanced total variation (ETV) regularization is devised to capture the inherent piecewise-smooth of the background component in both spectral and spatial domains. Furthermore, our algorithm incorporates group sparsity constraint and Gaussian noise term to enhance the discrimination between anomalies and background. Finally, a highly efficient update scheme based on the alternating direction method of multipliers (ADMM) is designed. A large number of experiments on one synthetic and seven real HSIs demonstrate the superiority of our proposed approach.
更多查看译文
关键词
Hyperspectral images (HSIs),anomaly detection,MERA decomposition,low-rank MERA approximation,enhanced total variation (ETV)
AI 理解论文
溯源树
样例
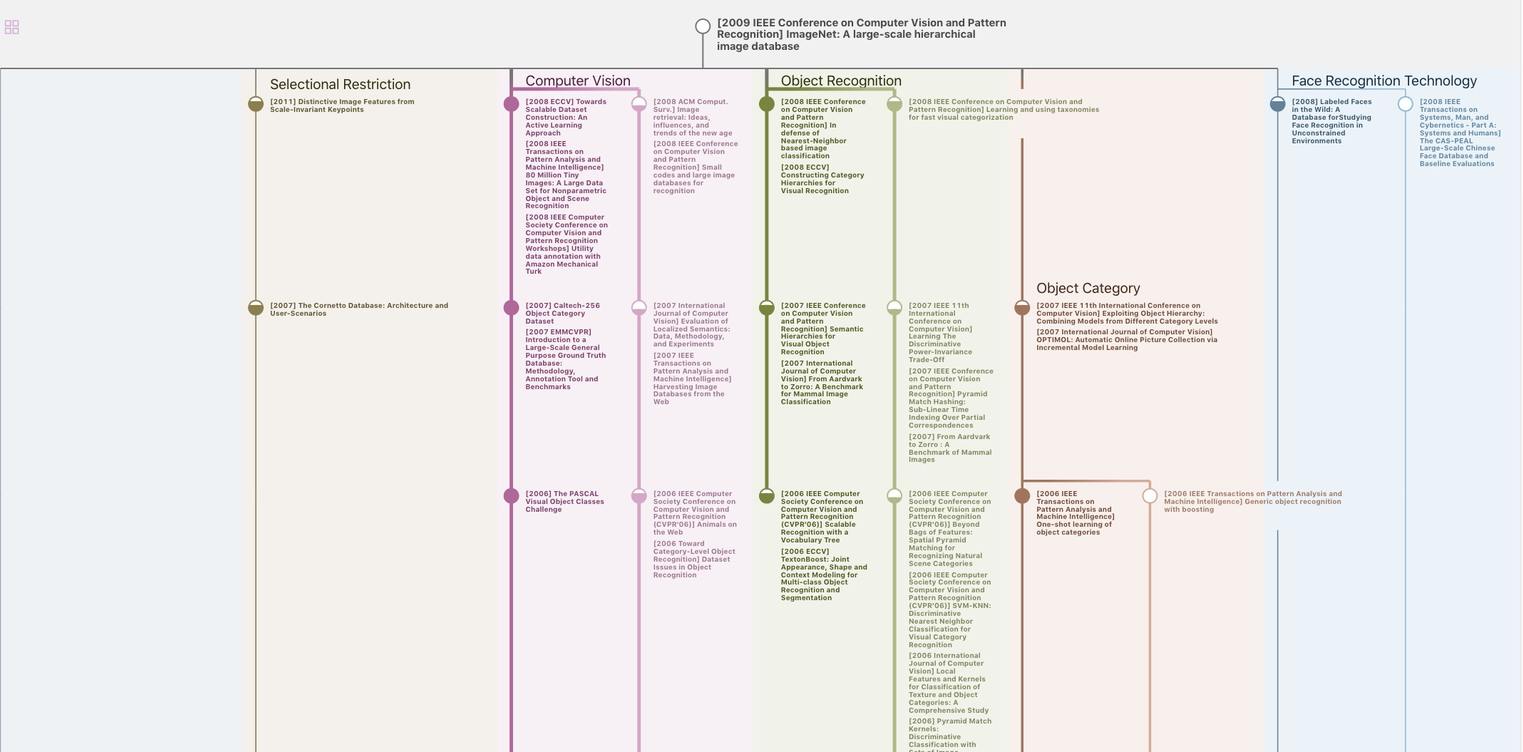
生成溯源树,研究论文发展脉络
Chat Paper
正在生成论文摘要