A multi-scale feature weighted transfer network for unlabeled rotating machinery fault diagnosis
IEEE Sensors Journal(2024)
摘要
Currently, bearings and gears play crucial roles as components in mechanical transmission systems, which fault diagnosis is of great significance for ensuring the safe operation of equipment. In practical industrial scenarios, the fault samples are often unlabeled, obtaining the complete training datasets required for training models is extremely difficult and expensive, and variable working conditions lead to differences in sample data distribution, which will reduce the diagnostic performance of fault recognition models. In response to these challenges, this paper proposes an intelligent fault diagnosis method for unlabeled rotating machinery based on the multi-scale feature weighted transfer network (MFWTN), which adopts a multi-scale network structure to extract multi-scale features of samples. The proposed method mitigates distribution differences between source and target domains by constraining features across multiple dimensions, reduces the negative transfer rate of samples, improves the transferability of sample features and the adaptability of the model, and achieves the goal of improving the accuracy of cross domain fault diagnosis of bearings and gears. MFWTN demonstrates superior performance and recognition accuracy compared to other comparative methods by verifying the performance of the proposed model on two public datasets.
更多查看译文
关键词
Multi-scale features,weighted transfer network,unlabeled,rotating machinery,fault diagnosis
AI 理解论文
溯源树
样例
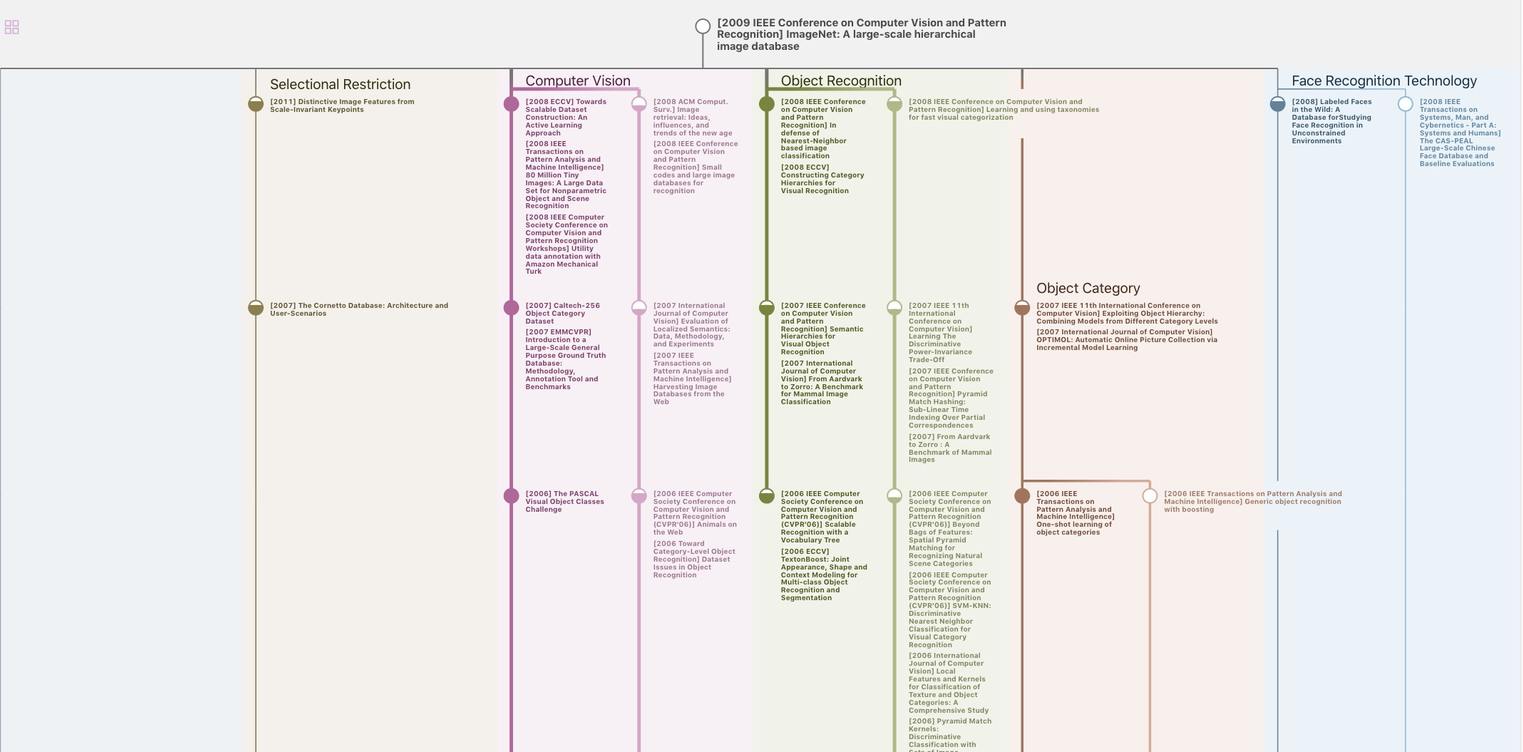
生成溯源树,研究论文发展脉络
Chat Paper
正在生成论文摘要