Remaining Useful Life Prediction Based on Sequential Bayesian Approach for Railway Balise
2023 Global Reliability and Prognostics and Health Management Conference (PHM-Hangzhou)(2023)
摘要
Balise plays an important role in Chinese train control system. Based on the principle of coupling circuit, it realizes the point-to-point information transmission between train and ground, and is used to determine the train running direction. However, during the operation of the balise, its circuit components will inevitably degrade and eventually lead to system failure. Since the degradation process must consider the effects of natural degradation and sudden shocks, and according to different conditions, the degradation curve conforms to a variety of different nonlinear distributions, it is not easy to predict the system failure time or remaining useful life (RUL) of the balise. This paper introduces a method combining interactive multi-model particle filter (IMMPF) and Metropolis-Hastings (MH) sampling algorithm, namely IMMPF-MH. It is used to update the online parameters of the dependent competing failure process (DCFP) model and predict the RUL of the balise based on the first arrival time concept. IMMPF algorithm works well in dealing with nonlinear and non-Gaussian systems, while MH is easy to deal with the diversity of time series distribution characteristics. The experimental results show the effectiveness of the model. Compared with other methods, the error is smaller, the parameter estimation is more accurate, and the RUL of the balise can be predicted more accurately with less sampling data.
更多查看译文
关键词
Balise,remaining useful life,interactive multi-model particle filter,Metropolis-Hastings,dependent competing failure process
AI 理解论文
溯源树
样例
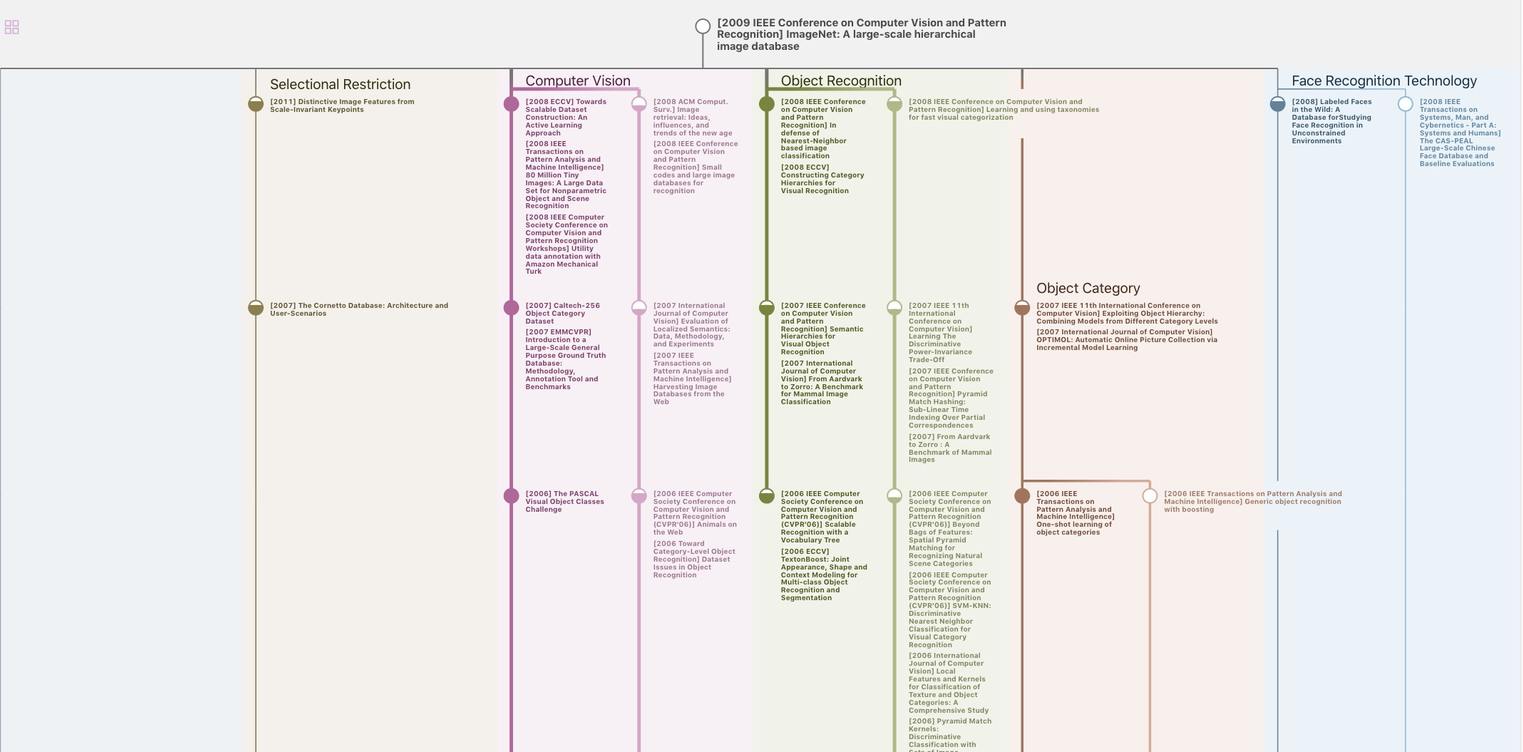
生成溯源树,研究论文发展脉络
Chat Paper
正在生成论文摘要