A Classification Catalog of Periodic Variable Stars for LAMOST DR9 Based on Machine Learning
ASTROPHYSICAL JOURNAL SUPPLEMENT SERIES(2024)
摘要
Identifying and classifying variable stars is essential to time-domain astronomy. The Large Area Multi-Object Fiber Optic Spectroscopic Telescope (LAMOST) acquired a large amount of spectral data. However, there is no corresponding variable source-related information in the data, constraining LAMOST data utilization for scientific research. In this study, we systematically investigated variable source classification methods for LAMOST data. We constructed a 10-class classification model using three mainstream machine-learning methods. Through performance comparison, we chose the LightGBM and XGBoost models. We further identified variable source candidates in the r band in LAMOST DR9 and obtained 281,514 variable source candidates with probabilities greater than 95%. Subsequently, we filtered out the sources of periodic variable sources using the generalized Lomb-Scargle periodogram and classified these periodic variable sources using the classification model. Finally, we propose a reliable periodic variable star catalog containing 176,337 stars with specific types.
更多查看译文
关键词
Catalogs,Variable stars,Cross-validation,Light curves
AI 理解论文
溯源树
样例
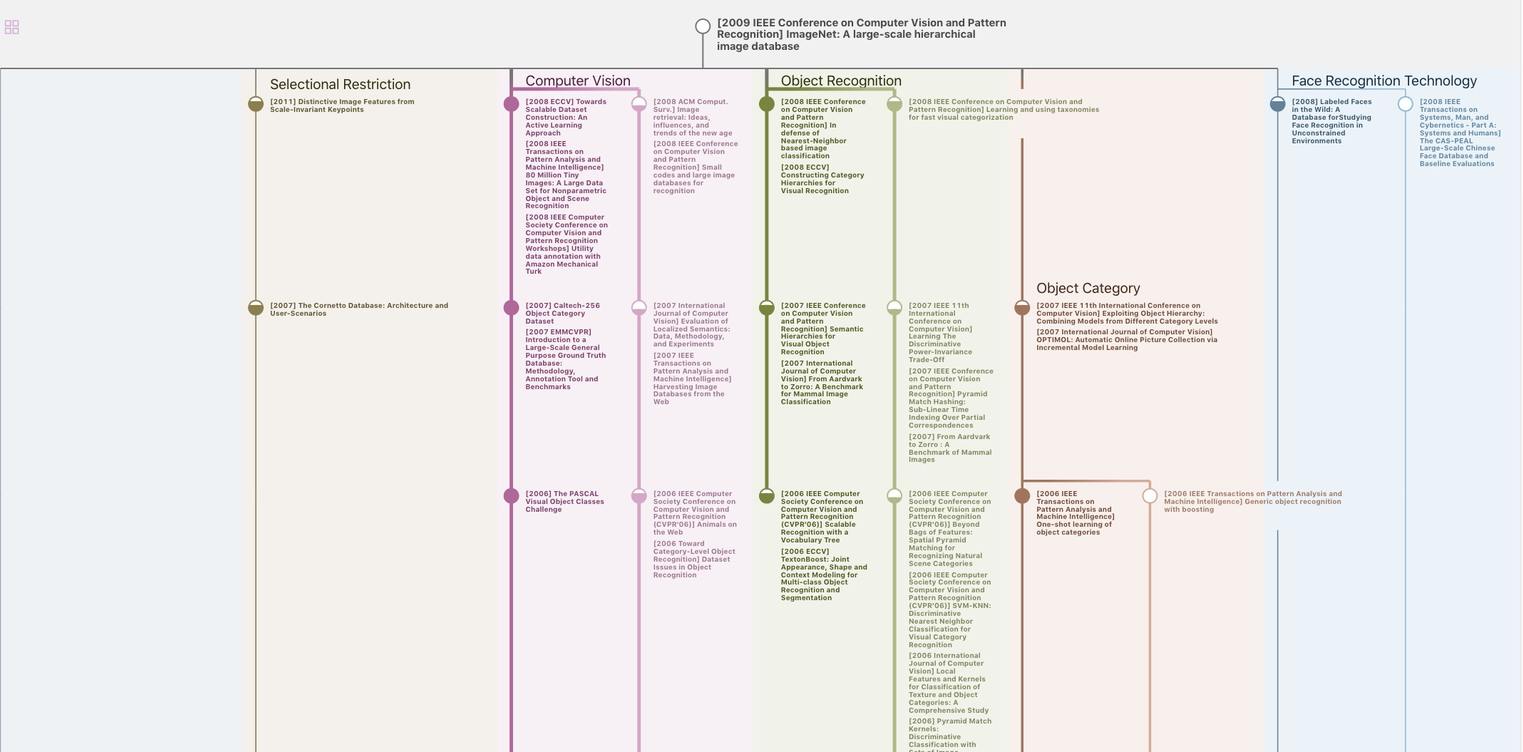
生成溯源树,研究论文发展脉络
Chat Paper
正在生成论文摘要