Self-supervised learning for medical image analysis: a comprehensive review
Evolving Systems(2024)
摘要
Deep learning and advancements in computer vision offer significant potential for analyzing medical images resulting in better healthcare and improved patient outcomes. Currently, the dominant approaches in the field of machine learning are supervised learning and transfer learning. These methods are not only prevalent in medicine and healthcare but also across various other industries. They rely on large datasets that have been manually annotated to train increasingly sophisticated models. However, the manual labeling process results in a wealth of untapped, unlabeled data that is accessible in both public and private data repositories. Self-supervised learning (SSL), an emerging field within machine learning, provides a solution by leveraging this untapped, unlabeled data. Unlike traditional machine learning paradigms, SSL algorithms pre-train models using artificial supervisory signals generated from the unlabeled data. This comprehensive review article explores the fundamental concepts, approaches, and advancements in self-supervised learning, with a particular emphasis on medical image datasets and their sources. By summarizing and highlighting the main contributions and findings from the article, this analysis and synthesis aim to shed light on the current state of research in self-supervised learning. Through these rigorous efforts, the existing body of knowledge is synthesized, and implementation recommendations are provided for future researchers interested in harnessing self-supervised learning to develop classification models for medical imaging.
更多查看译文
关键词
Contrastive learning,CT images,Medical imaging,Pretext task,Self -supervised learning
AI 理解论文
溯源树
样例
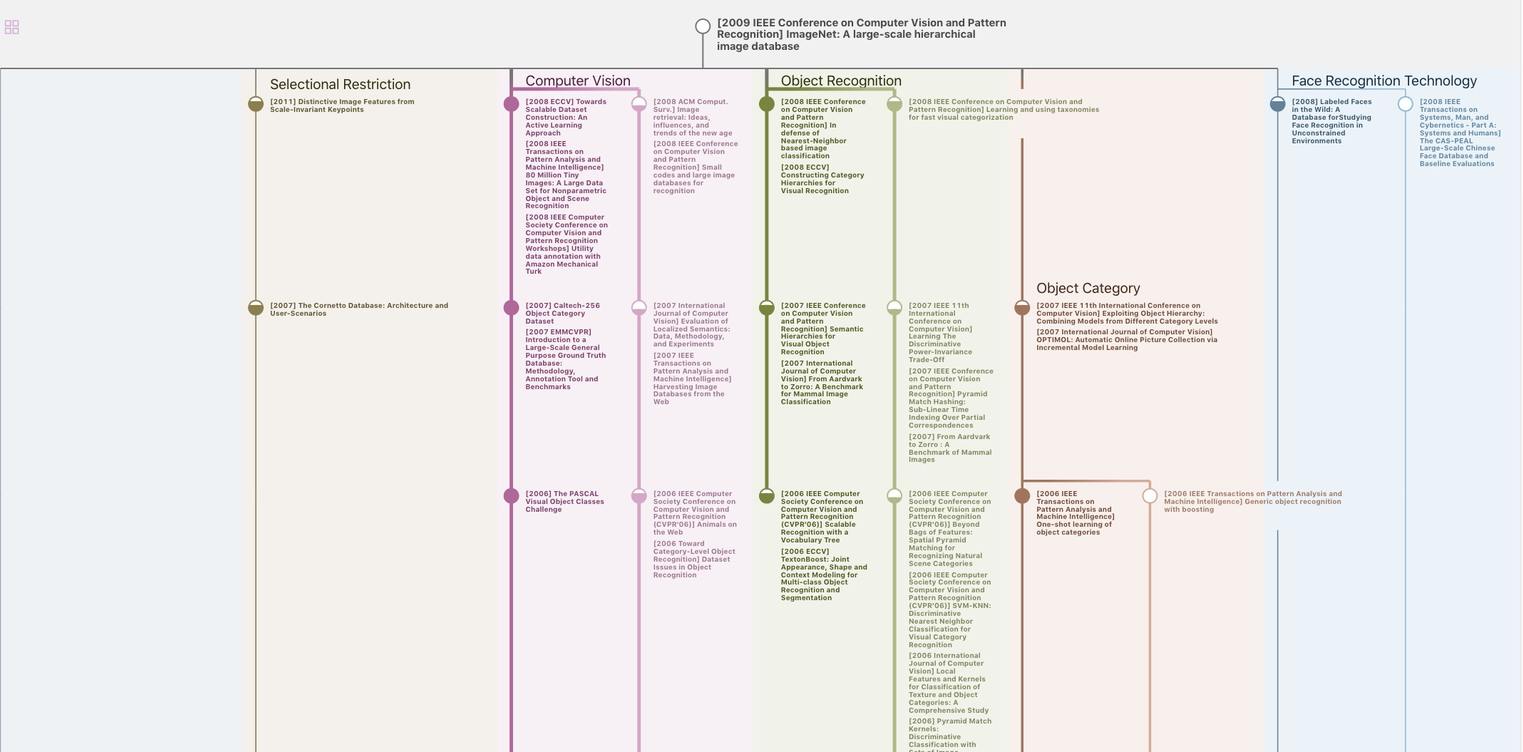
生成溯源树,研究论文发展脉络
Chat Paper
正在生成论文摘要