Dual-level feature assessment for unsupervised multi-view feature selection with latent space learning
Information Sciences(2024)
摘要
Recently, numerous unsupervised multi-view feature selection methods have been presented. However, these methods assess the significance of data features in each view individually or jointly evaluate the significance of data features across multiple views by concatenating data features from all data views. So, they disregard either inter-view or intra-view feature connections of data. Moreover, they ignore the complementary information from multiple insufficient views, each of which only captures partial information of multi-view data and is insufficient to characterize data distribution. To address these problems, this paper proposes a model named Dual-level Feature Assessment for Unsupervised Multi-view Feature Selection with Latent Space Learning (DFA-LSL). It first investigates the underlying complementary information from multiple views in a latent space while simultaneously learning latent representations for data. Compared with the original data representations, the latent representations contain the entire information of multi-view data. Then, it explores connections and distinguishing ability of features at two levels, namely the inter-view and intra-view levels, by jointly assessing the significance of features in the latent space and individually assessing the significance of features in each view concurrently. Following that, an effective optimization algorithm is presented. Extensive experiments demonstrate that the proposed work surpasses several state-of-the-art models.
更多查看译文
关键词
Multi-view learning,Unsupervised feature selection,Dual-level feature assessment,Latent space learning,Local structure learning
AI 理解论文
溯源树
样例
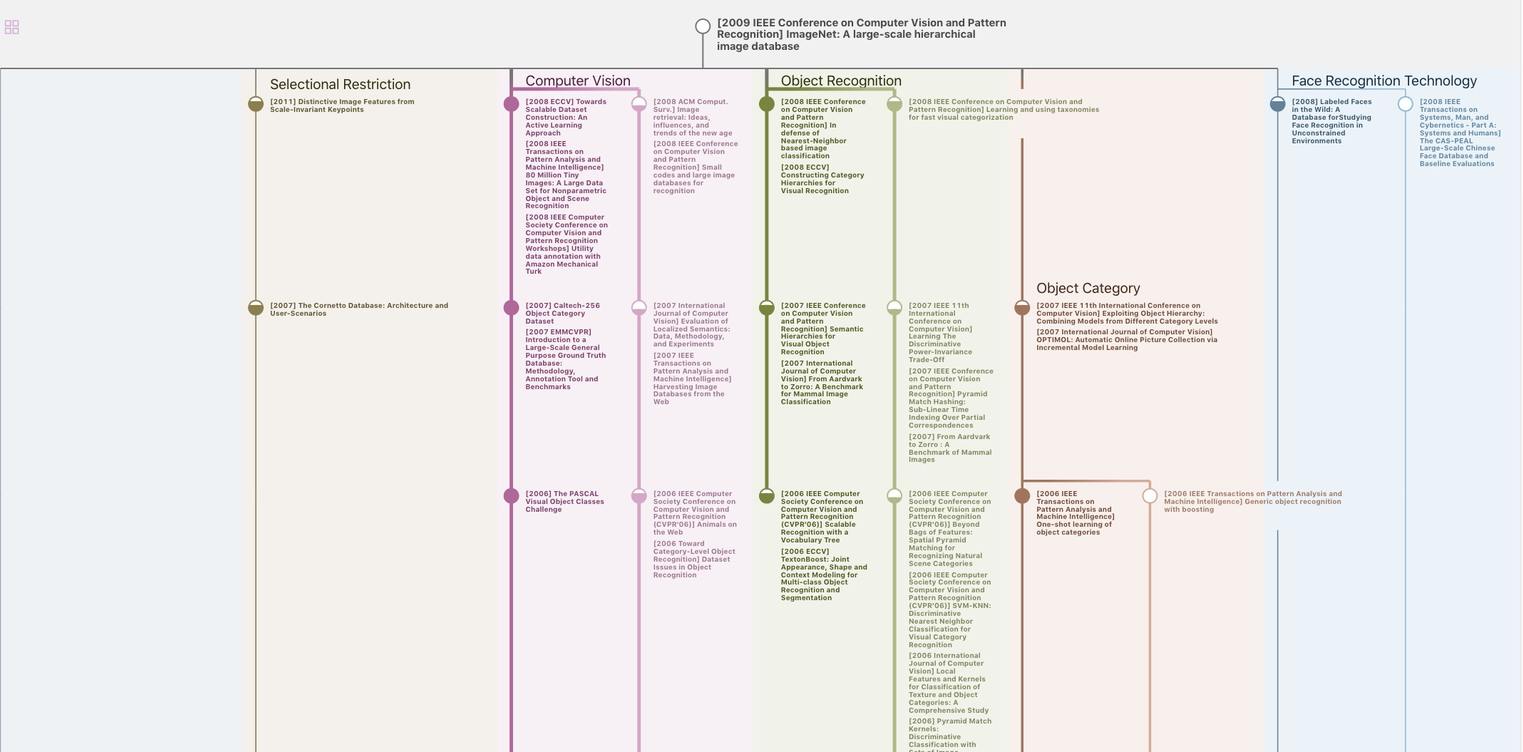
生成溯源树,研究论文发展脉络
Chat Paper
正在生成论文摘要