Neural Network-Based Approach for Failure and Life Prediction of Electronic Components under Accelerated Life Stress
ELECTRONICS(2024)
摘要
Researchers worldwide have been focusing on accurately predicting the remaining useful life of electronic devices to ensure reliability in various industries. This has been made possible by advancements in artificial intelligence (AI), machine learning, and Internet of Things (IoT) technologies. However, accurately forecasting device life with minimal data sets, especially in industrial applications, remains a challenge. This paper aims to address this challenge by utilizing machine learning algorithms, specifically BP, XGBOOST, and KNN, to predict device reliability with limited data. The remaining life dataset of electronic components is obtained through simulation for training and testing the algorithms, and the experimental results show that the algorithms achieve a certain level of accuracy, with the error rates being as follows: BP algorithm, 0.01–0.02%; XGBOOST algorithm, 0.01–0.02%; and KNN algorithm, 0–0.07%. By benchmarking these algorithms, the study demonstrates the feasibility of deploying machine learning models for device life prediction with acceptable accuracy loss, and highlights the potential of AI algorithms in predicting the reliability of electronic devices.
更多查看译文
关键词
neural networks,machine learning,BP algorithm,XGBOOST algorithm,KNN algorithm,remaining life prediction
AI 理解论文
溯源树
样例
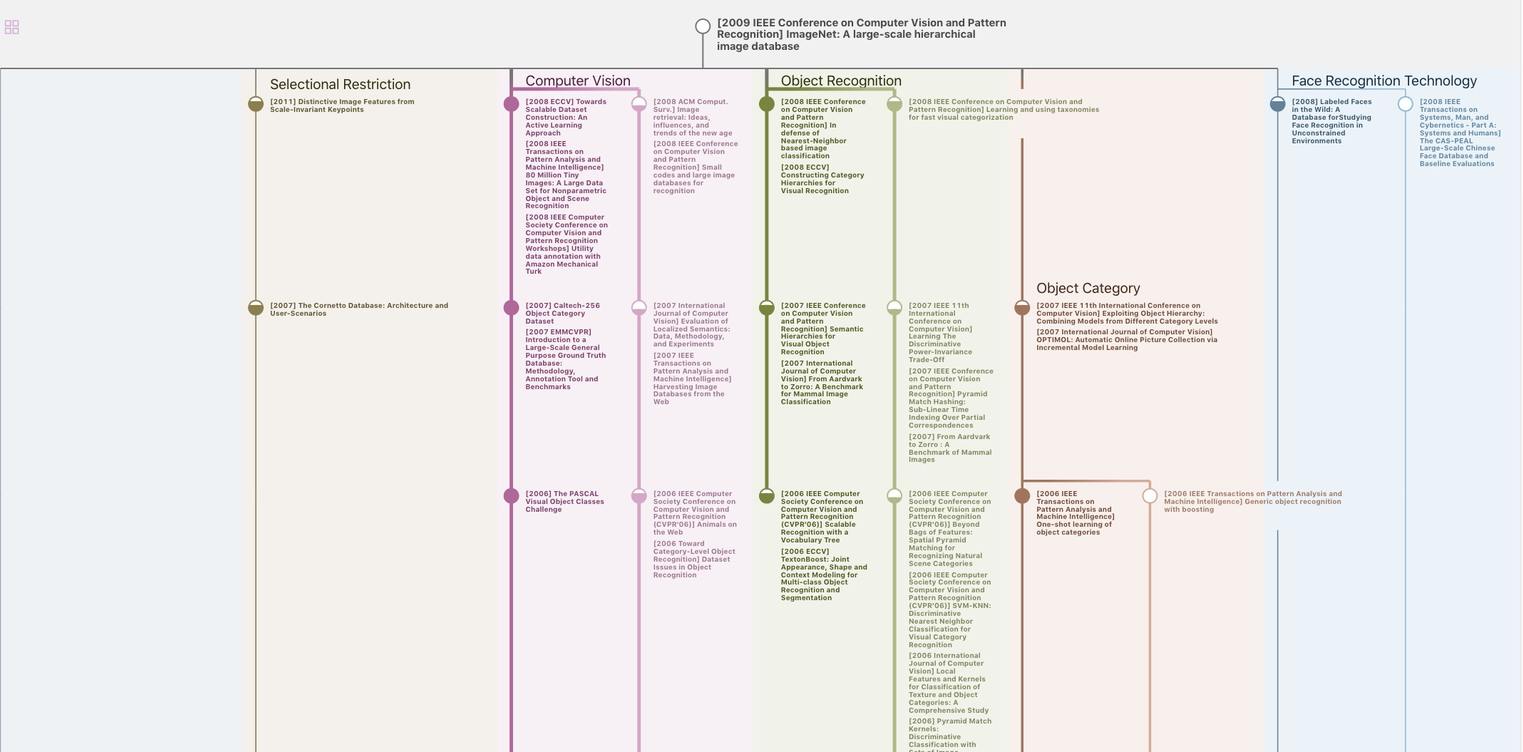
生成溯源树,研究论文发展脉络
Chat Paper
正在生成论文摘要