Navigating Uncertainty: Semantic-Powered Image Enhancement and Fusion
IEEE signal processing letters(2024)
摘要
The fusion of infrared and visible imagery plays a crucial role in environmental monitoring. Existing approaches aim to achieve high-quality perceptual results for human observers and robust outcomes for machine-based high-level tasks by adopting a joint design of fusion and segmentation. However, design constraints imposed by predetermined fusion rules limit the precision of high-level tasks, and drawbacks in the image domains to be fused are often overlooked. This letter presents a novel semantic-powered infrared and visible image fusion framework to address these issues. The key feature of our approach is the utilization of trainable gains and weights in enhancement and fusion processes, influenced solely by segmentation and serving as uncertainty parameters. We propose a two-stage training strategy: initially, training a combined enhancement and fusion network with random uncertainty parameters, followed by the estimation of semantic-driven uncertainty parameters. The enhancement and fusion process is optimized within the Laplacian pyramid framework to ensure efficient computation. Experimental results highlight the significance of modeling the fusion process with uncertainty for achieving satisfactory fusion and segmentation outcomes.
更多查看译文
关键词
Uncertainty,Task analysis,Training,Laplace equations,Visualization,Image segmentation,Semantics,Infrared and visible images fusion,Laplacian pyramid,task-oriented fusion
AI 理解论文
溯源树
样例
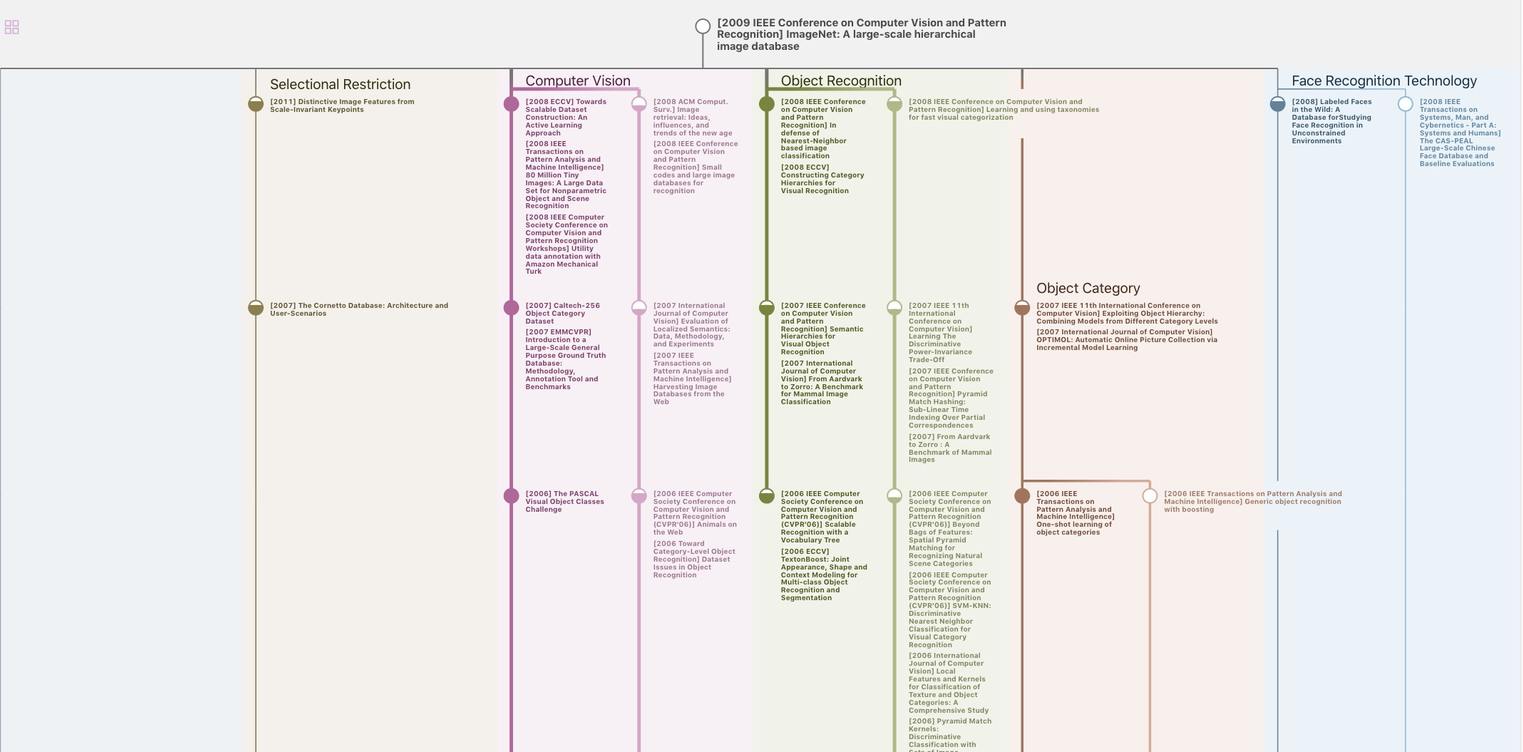
生成溯源树,研究论文发展脉络
Chat Paper
正在生成论文摘要