Is a direct numerical simulation (DNS) of Navier-Stokes equations with small enough grid spacing and time-step definitely reliable/correct?
arxiv(2024)
摘要
Traditionally, results given by the direct numerical simulation (DNS) of
Navier-Stokes equations are widely regarded as reliable benchmark solutions of
turbulence, as long as grid spacing is fine enough (i.e. less than the minimum
Kolmogorov scale) and time-step is small enough, say, satisfying the
Courant-Friedrichs-Lewy condition. Is this really true? In this paper a
two-dimensional sustained turbulent Kolmogorov flow is investigated numerically
by the two numerical methods with detailed comparisons: one is the traditional
`direct numerical simulation' (DNS), the other is the `clean numerical
simulation' (CNS). The results given by DNS are a kind of mixture of the false
numerical noise and the true physical solution, which however are mostly at the
same order of magnitude due to the butterfly-effect of chaos. On the contrary,
the false numerical noise of the results given by CNS is much smaller than the
true physical solution of turbulence in a long enough interval of time so that
a CNS result is very close to the true physical solution and thus can be used
as a benchmark solution. It is found that numerical noise as a kind of
artificial tiny disturbances can lead to huge deviations at large scale on the
two-dimensional Kolmogorov turbulence, not only quantitatively (even in
statistics) but also qualitatively (such as symmetry of flow). Thus, fine
enough spatial grid spacing with small enough time-step alone cannot guarantee
the validity of the DNS: it is only a necessary condition but not sufficient.
This finding might challenge some assumptions in investigation of turbulence.
So, DNS results of a few sustained turbulent flows might have huge deviations
on both of small and large scales from the true solution of Navier-Stokes
equations even in statistics. Hopefully, CNS as a new tool to investigate
turbulent flows more accurately than DNS could bring us some new discoveries.
更多查看译文
AI 理解论文
溯源树
样例
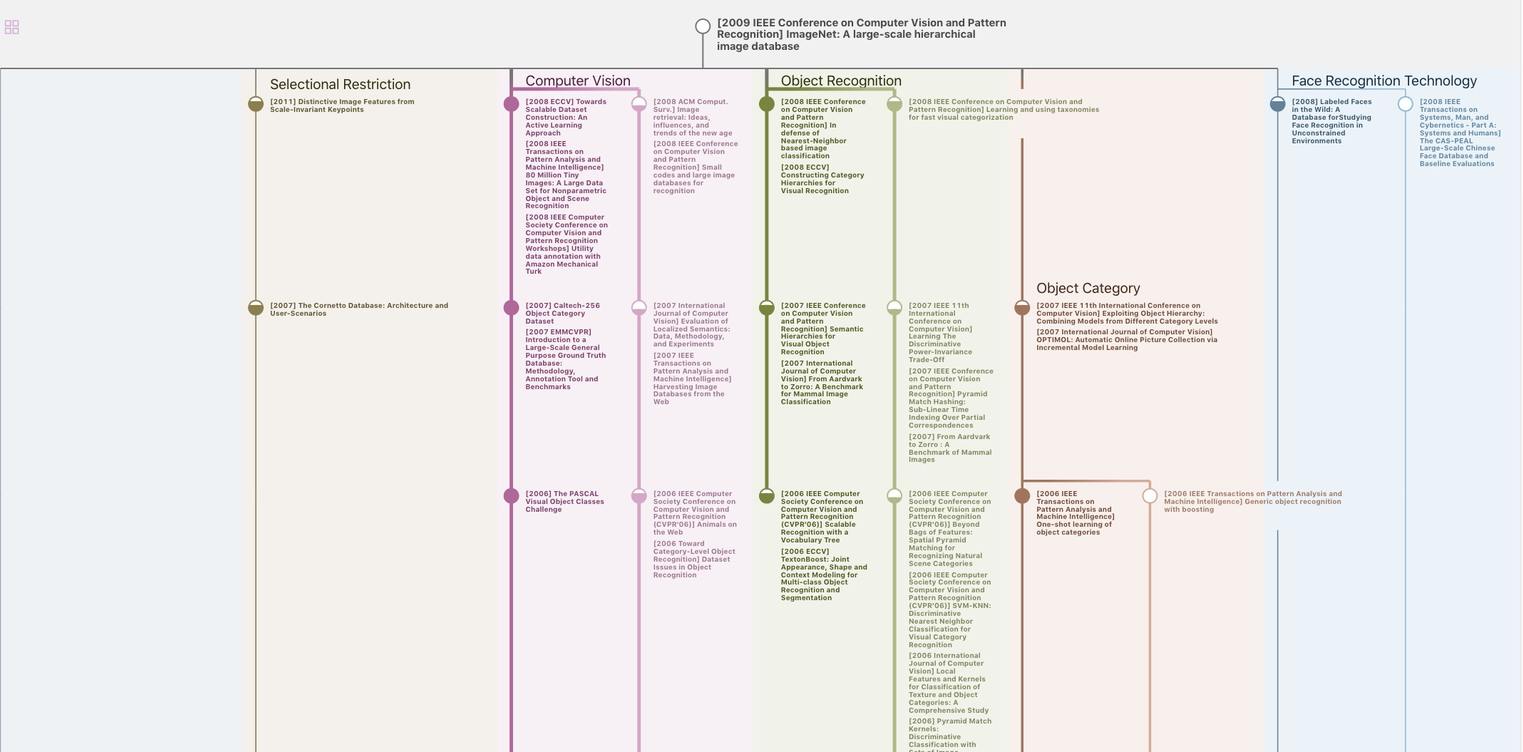
生成溯源树,研究论文发展脉络
Chat Paper
正在生成论文摘要