Knowledge-Distillation-Based Label Smoothing for Fine-Grained Open-Set Vehicle Recognition.
IEEE/CVF Winter Conference on Applications of Computer Vision(2024)
摘要
Fine-grained vehicle classification describes the task of estimating the make and the model of a vehicle based on an image. It provides a useful tool for security authorities to find suspects in surveillance cameras. However, most research about fine-grained vehicle classification is only focused on a closed-set scenario which considers all possible classes to be included in the training. This is not realistic for real-world surveillance applications where the images fed into the classifier can be of arbitrary vehicle models and the large number of commercially available vehicle models renders learning all models impossible. Thus, we investigate fine-grained vehicle classification in an open-set recognition scenario which includes unknown vehicle models in the test set and expects these samples to be rejected. Our experiments highlight the importance of label smoothing for open-set recognition performance. Nonetheless, it lacks recognizing the different semantic distances between vehicle models which result in largely different confusion probabilities. Thus, we propose a knowledge-distillation-based label smoothing approach which considers these different semantic similarities and thus, improves the closed-set classification as well as the open-set recognition performance.
更多查看译文
关键词
Semantic Similarity,Surveillance Cameras,Semantic Distance,Training Set,Learning Rate,Classification Performance,F1 Score,Image Classification,Deep Learning Models,Confusion Matrix,Data Augmentation,Task Difficulty,Classifier Training,Softmax Function,Targeting Vector,Semantic Differential,Energy Score,Target Label,One-hot Encoding,Student Network,Distance-based Approach,Classification Confusion,Feature Space,Subset Of Samples,Deep Learning,Hyperparameters
AI 理解论文
溯源树
样例
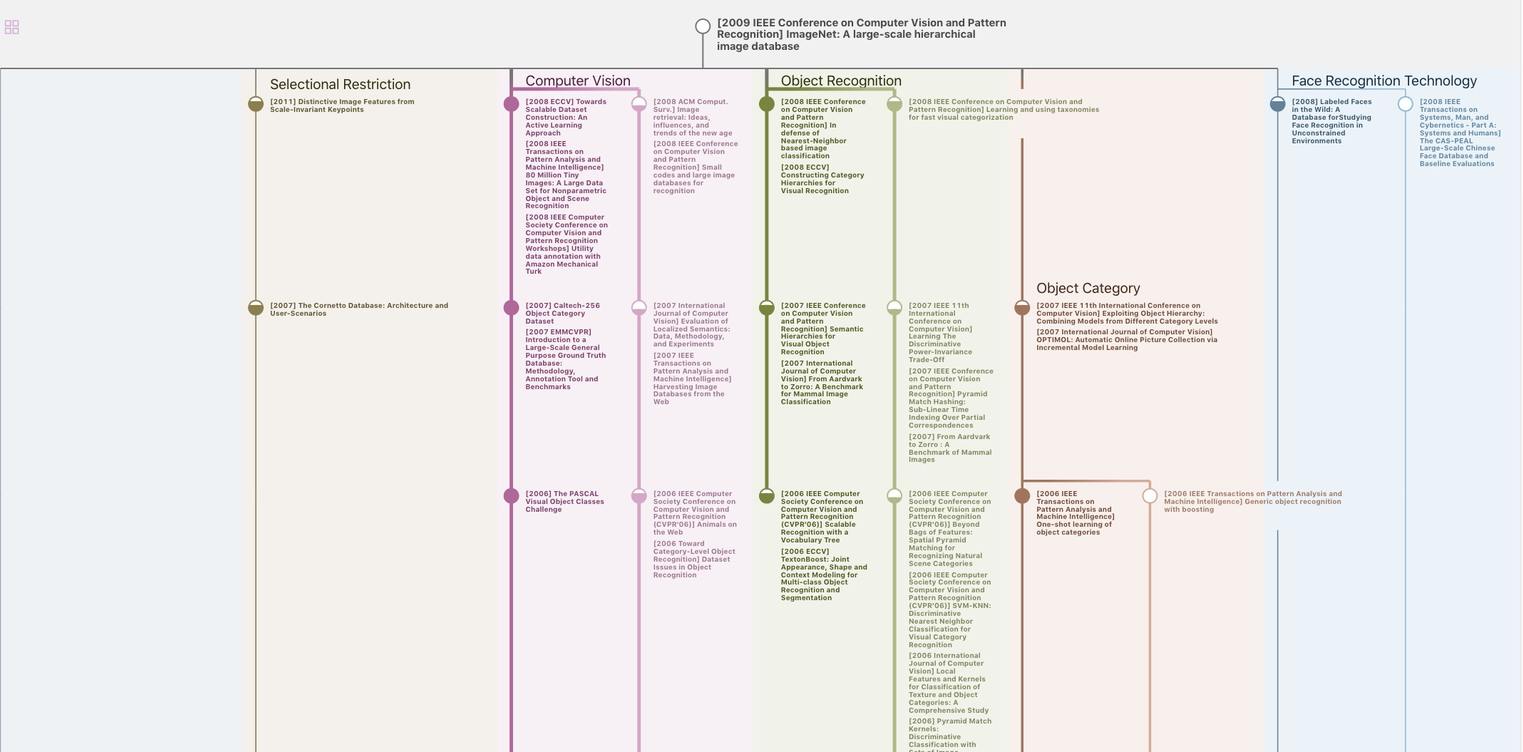
生成溯源树,研究论文发展脉络
Chat Paper
正在生成论文摘要