Region-based compressive distributed storage in mobile crowdSensing
Future Generation Computer Systems(2024)
摘要
Compressive Sensing-based distributed data storage for Mobile CrowdSensing (MCS) primarily addresses the problem that data needs to be stored temporarily on mobile devices when the central server can not work as expected. In the crowdsensing-aware platform, the emerging distributed storage scheme mainly recovers the data fields of the entire area. However, when only local area information is required, it is still necessary to recover the entire area, creating unnecessary waste. Based on this observation, this paper proposes a Region-based Distributed Data Storage with global Denoising (R-DDS-D) algorithm. Region-based distributed data storage strategy is individually coded in regional blocks, allowing users to reconstruct the regional information they need selectively. Simultaneously, global denoising can be achieved through the correlation between small regions, which can further improve the accuracy of reconstructed data. In addition, not all local region information is equally significant, an extended algorithm for sampling allocation is proposed considering the importance of different regions to further improve accuracy. Theoretical analysis demonstrates that R-DDS-D can recover the signal with high probability, and the sampling allocation method can more reasonably allocate sampling resources. Experimentally, the R-DDS-D scheme offers greater flexibility, recruiting cost savings, and smoother reconstruction performance for recovering local regions, and the sampling allocation method further enhances the reconstruction quality in the case of uneven data distribution.
更多查看译文
关键词
Compressive sensing,Distributed data storage,Global denoising,Mobile crowdsensing,Sampling allocation
AI 理解论文
溯源树
样例
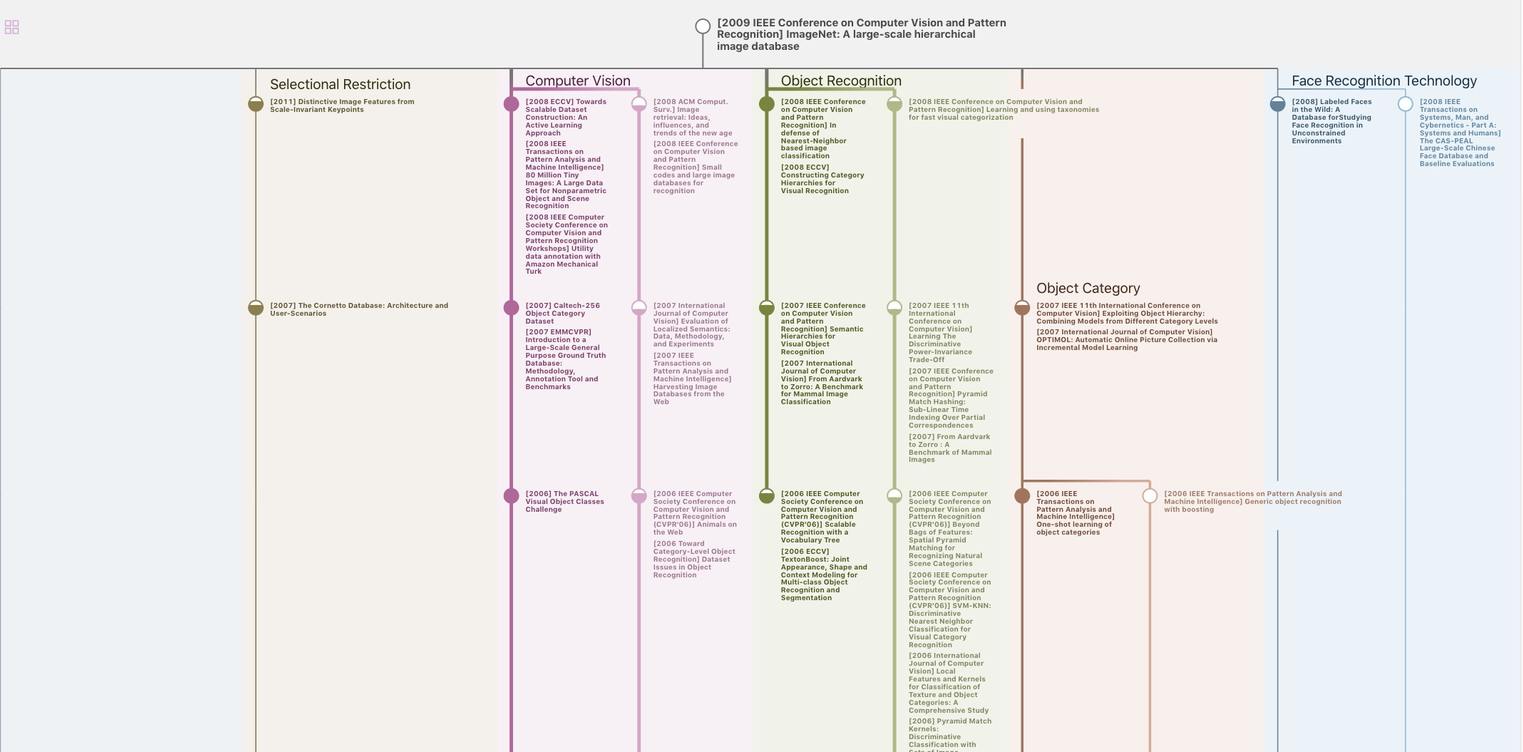
生成溯源树,研究论文发展脉络
Chat Paper
正在生成论文摘要