Deep Learning‐based Grain‐size Decomposition Model: A Feasible Solution for Dealing with Methodological Uncertainty
Sedimentology(2024)
摘要
ABSTRACTTerrigenous clastic sediments cover a large area of the Earth's surface and provide valuable insights into the Earth's evolution and environmental change. Sediment grain‐size decomposition has been widely used as an effective approach to inferring changes in sediment sources, transport processes and depositional environments. Several algorithms, such as single sample unmixing, end‐member modelling analysis and the universal decomposition model, have been developed for grain‐size decomposition. The performance of these algorithms is highly dependent on parameter selections, introducing subjective uncertainty. This uncertainty could undermine the reliability of decomposition results, limit the application of grain‐size decomposition techniques and reduce comparability across different studies. To mitigate the methodological uncertainty, a novel deep learning‐based framework for grain‐size decomposition of terrigenous clastic sediments is proposed. First, an improved universal decomposition model is used to analyse the collected grain‐size data, in order to provide training sets for the end‐to‐end decomposers. To meet the data size requirements of supervised learning, generative adversarial networks are also trained for data augmentation. The performance of the new framework is then evaluated using a small‐scale dataset (73 393 samples from 18 sites) of three sedimentary types (loess, fluvial and lake delta deposits). The decomposed grain‐size results demonstrate high feasibility and great potential of the framework in constructing a robust grain‐size decomposition model. Finally, it is proposed that future grain‐size research should aim to establish guidelines for grain‐size data sharing and produce a big grain‐size database for deep learning.
更多查看译文
关键词
Convolutional neural network,data augmentation,deep learning,generative adversarial network,grain-size decomposition
AI 理解论文
溯源树
样例
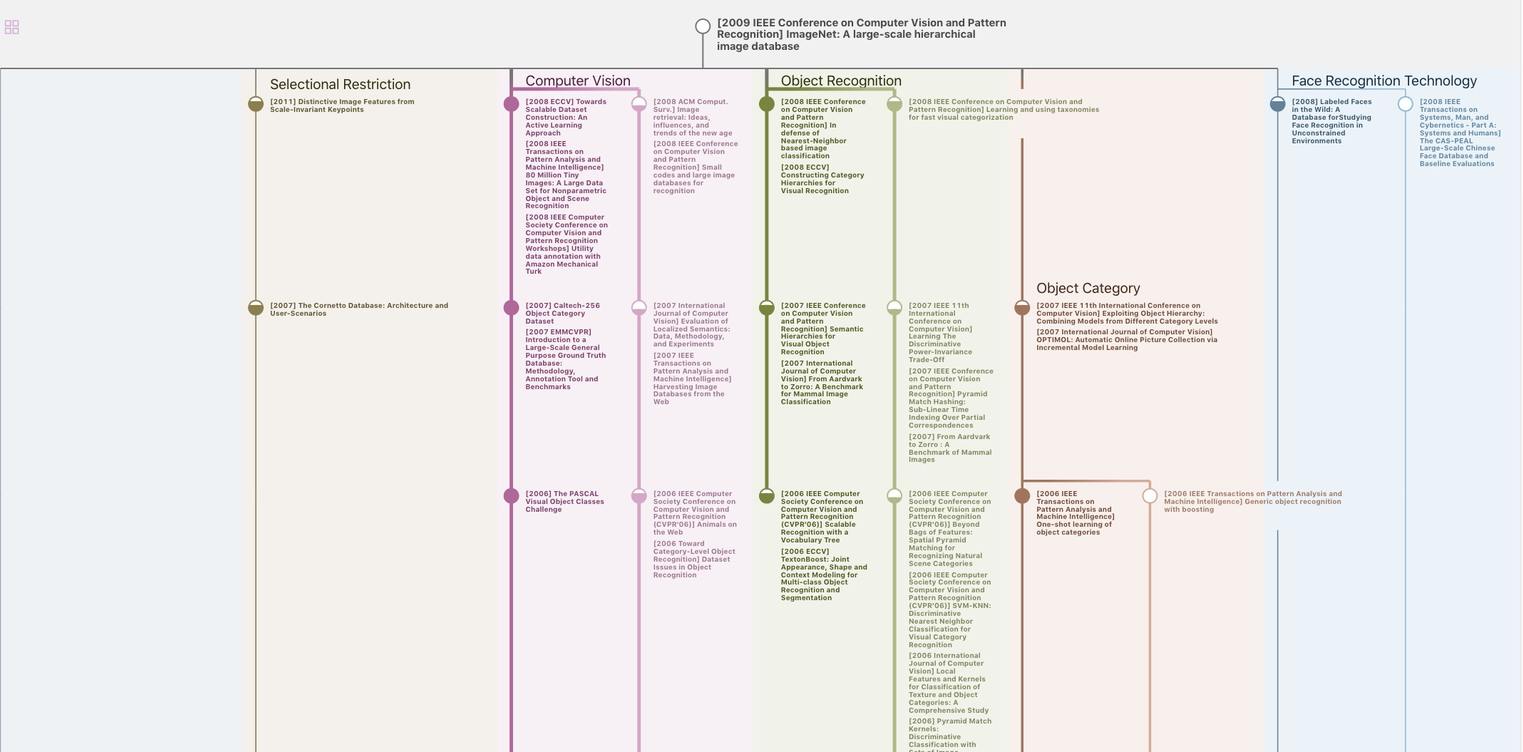
生成溯源树,研究论文发展脉络
Chat Paper
正在生成论文摘要