DeepLIR: Attention-Based Approach for Mask-Based Lensless Image Reconstruction.
IEEE/CVF Winter Conference on Applications of Computer Vision(2024)
摘要
Lensless imaging has emerged as a promising solution to overcome the need for expensive and bulky lenses used in traditional cameras. This technique leverages a mask to op-tically encode the scene, thus generating a sensor pattern. The image is subsequently reconstructed using a computational algorithm. Traditional model-based reconstruction methods often suffer from prolonged convergence time and subpar perceptual image quality. To mitigate these issues, data-driven deep neural networks can potentially offer enhanced reconstruction quality alongside reduced inference time. However, deep learning methods fall short in providing improved results and tend to produce artifacts, primarily because they do not incorporate any prior knowledge about the imaging model. In this work, we propose a DeepLIR, a hybrid approach that combines the physical system model with a deep learning model. This is achieved by unrolling a conventional model-based optimization al-gorithm and incorporating an attention-based deep learning model to denoise the image, thereby enhancing the reconstruction quality. Our empirical analysis confirms that DeepLIR surpasses existing lensless image reconstruction techniques in terms of image quality and computational ef-ficiency. Specifically, DeepLIR achieves a remarkable 1.35 × improvement in perceptual quality over the nearest com-petitor, reflecting its robustness and superiority. Further-more, it demonstrates superior generalization capabilities when applied to real-world imaging. Code available at: https://github.com/arpanpoudel/lenslessimaging.
更多查看译文
关键词
Image Reconstruction,Lensless Imaging,Image Reconstruction Approach,Neural Network,Image Quality,Deep Neural Network,Perception Of Quality,Inference Time,Reconstruction Quality,Real-world Images,Traditional Cameras,Imaging System,Unsupervised Learning,Attention Mechanism,Image Pairs,Trainable Parameters,Real-world Scenarios,Inverse Problem,Image Formation,Forward Model,Peak Signal-to-noise Ratio,Noisy Images,Sensor Measurements,Discrete Cosine Transform,Vision Transformer,Residual Connection,Up-sampling Operation,Reconstruction Artifacts,Self-attention Mechanism,Visual Quality
AI 理解论文
溯源树
样例
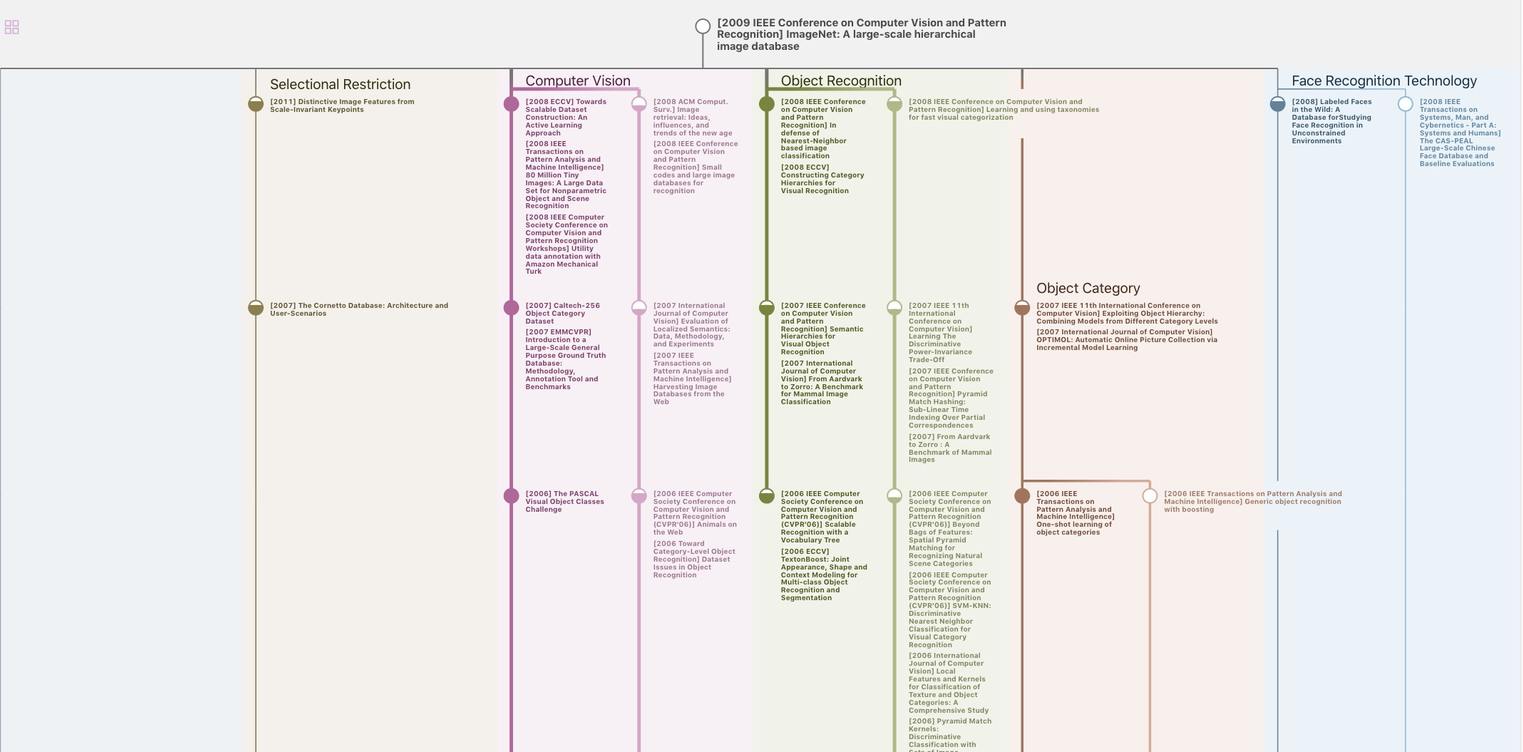
生成溯源树,研究论文发展脉络
Chat Paper
正在生成论文摘要