Machine learning for predicting Chagas disease infection in rural areas of Brazil
PLOS NEGLECTED TROPICAL DISEASES(2024)
摘要
Introduction Chagas disease is a severe parasitic illness that is prevalent in Latin America and often goes unaddressed. Early detection and treatment are critical in preventing the progression of the illness and its associated life-threatening complications. In recent years, machine learning algorithms have emerged as powerful tools for disease prediction and diagnosis.Methods In this study, we developed machine learning algorithms to predict the risk of Chagas disease based on five general factors: age, gender, history of living in a mud or wooden house, history of being bitten by a triatomine bug, and family history of Chagas disease. We analyzed data from the Retrovirus Epidemiology Donor Study (REDS) to train five popular machine learning algorithms. The sample comprised 2,006 patients, divided into 75% for training and 25% for testing algorithm performance. We evaluated the model performance using precision, recall, and AUC-ROC metrics.Results The Adaboost algorithm yielded an AUC-ROC of 0.772, a precision of 0.199, and a recall of 0.612. We simulated the decision boundary using various thresholds and observed that in this dataset a threshold of 0.45 resulted in a 100% recall. This finding suggests that employing such a threshold could potentially save 22.5% of the cost associated with mass testing of Chagas disease.Conclusion Our findings highlight the potential of applying machine learning to improve the sensitivity and effectiveness of Chagas disease diagnosis and prevention. Furthermore, we emphasize the importance of integrating socio-demographic and environmental factors into neglected disease prediction models to enhance their performance. Chagas disease, a severe parasitic illness prevalent in Latin America, poses significant challenges due to delayed detection and treatment. Machine learning algorithms, advanced computer programs, have emerged as valuable tools for disease prediction and diagnosis. In our study, we utilized these algorithms to forecast Chagas disease risk based on factors such as age, gender, and living conditions. Drawing on data from the Retrovirus Epidemiology Donor Study (REDS), we trained five algorithms, with one showing promising results, achieving an impressive score of 0.772 out of 1. By establishing a specific threshold, we could potentially reduce testing costs while maintaining high detection rates. This research highlights the potential of machine learning in improving Chagas disease diagnosis and prevention by incorporating socio-demographic and environmental factors. Integrating these elements into predictive models has the potential to enhance their effectiveness and sensitivity, thereby improving disease management outcomes and ultimately reducing the burden of Chagas disease in affected regions.
更多查看译文
AI 理解论文
溯源树
样例
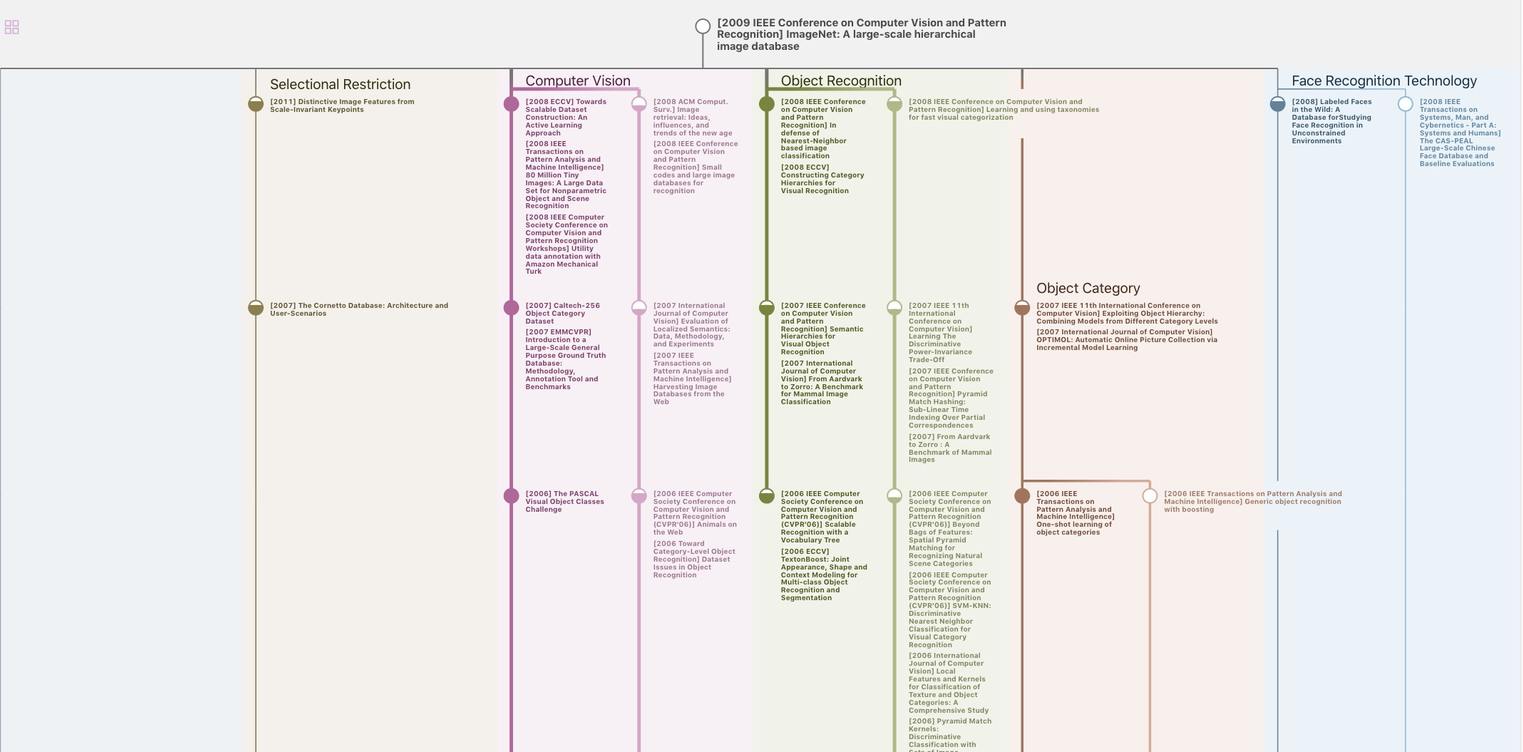
生成溯源树,研究论文发展脉络
Chat Paper
正在生成论文摘要