Residential load forecasting based on symplectic geometry mode decomposition and GRU neural network with attention mechanism
APPLIED STOCHASTIC MODELS IN BUSINESS AND INDUSTRY(2024)
摘要
Short-term residential load forecasting plays an increasingly important role in modern smart grids, with its main challenge being the high volatility and uncertainty of load curves. This article proposes a hybrid Symplectic Geometry Mode Decomposition-Gated Recurrent Unit with Attention Mechanism (SGMD-GRUAM) model for hourly residential load forecasting. First, SGMD is used to decompose the residential load and obtain a series of stable subsequences. Then, the Pearson correlation coefficient is used to select features related to each subsequence, such as weather factors. Next, a GRUAM prediction model is constructed for each subsequence. Finally, the final load prediction value is obtained by superimposing the previous component sequences and eliminating the noise sequence. The experiment uses the public dataset from UMass for a case study and compares it with benchmark models such as ARIMA and EEDM-GRUAM. The experimental results show that the proposed SGMD-GRUAM model has significant advantages in terms of prediction performance.
更多查看译文
关键词
attention mechanism,gated recurrent unit (GRU),residential load forecasting,short-term load forecasting (STLF),symplectic geometry mode decomposition (SGMD)
AI 理解论文
溯源树
样例
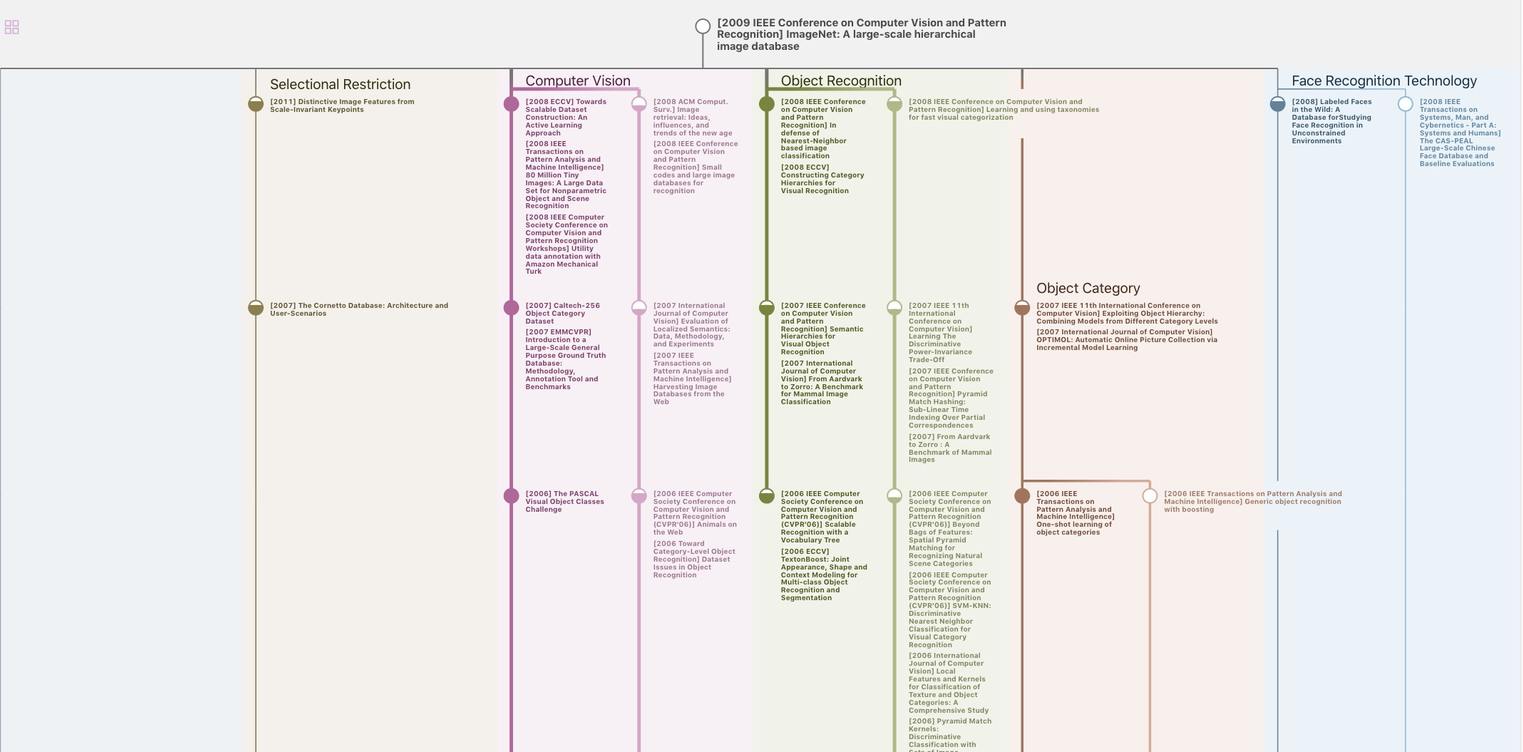
生成溯源树,研究论文发展脉络
Chat Paper
正在生成论文摘要