DEs-Inspired Accelerated Unfolded Linearized ADMM Networks for Inverse Problems
IEEE transactions on neural networks and learning systems(2024)
摘要
Many research works have shown that the traditional alternating direction multiplier methods (ADMMs) can be better understood by continuous-time differential equations (DEs). On the other hand, many unfolded algorithms directly inherit the traditional iterations to build deep networks. Although they achieve superior practical performance and a faster convergence rate than traditional counterparts, there is a lack of clear insight into unfolded network structures. Thus, we attempt to explore the unfolded linearized ADMM (LADMM) from the perspective of DEs, and design more efficient unfolded networks. First, by proposing an unfolded Euler LADMM scheme and inspired by the trapezoid discretization, we design a new more accurate Trapezoid LADMM scheme. For the convenience of implementation, we provide its explicit version via a prediction-correction strategy. Then, to expand the representation space of unfolded networks, we design an accelerated variant of our Euler LADMM scheme, which can be interpreted as second-order DEs with stronger representation capabilities. To fully explore this representation space, we designed an accelerated Trapezoid LADMM scheme. To the best of our knowledge, this is the first work to explore a comprehensive connection with theoretical guarantees between unfolded ADMMs and first-(second-) order DEs. Finally, we instantiate our schemes as (A-)ELADMM and (A-)TLADMM with the proximal operators, and (A-)ELADMM-Net and (A-)TLADMM-Net with convolutional neural networks (CNNs). Extensive inverse problem experiments show that our Trapezoid LADMM schemes perform better than well-known methods.
更多查看译文
关键词
Differential equations,Convex functions,Optimization,Inverse problems,Convergence,Space exploration,Extrapolation,Acceleration,differential equations (DEs),inverse problems,network structure design,unfolded LADMM networks
AI 理解论文
溯源树
样例
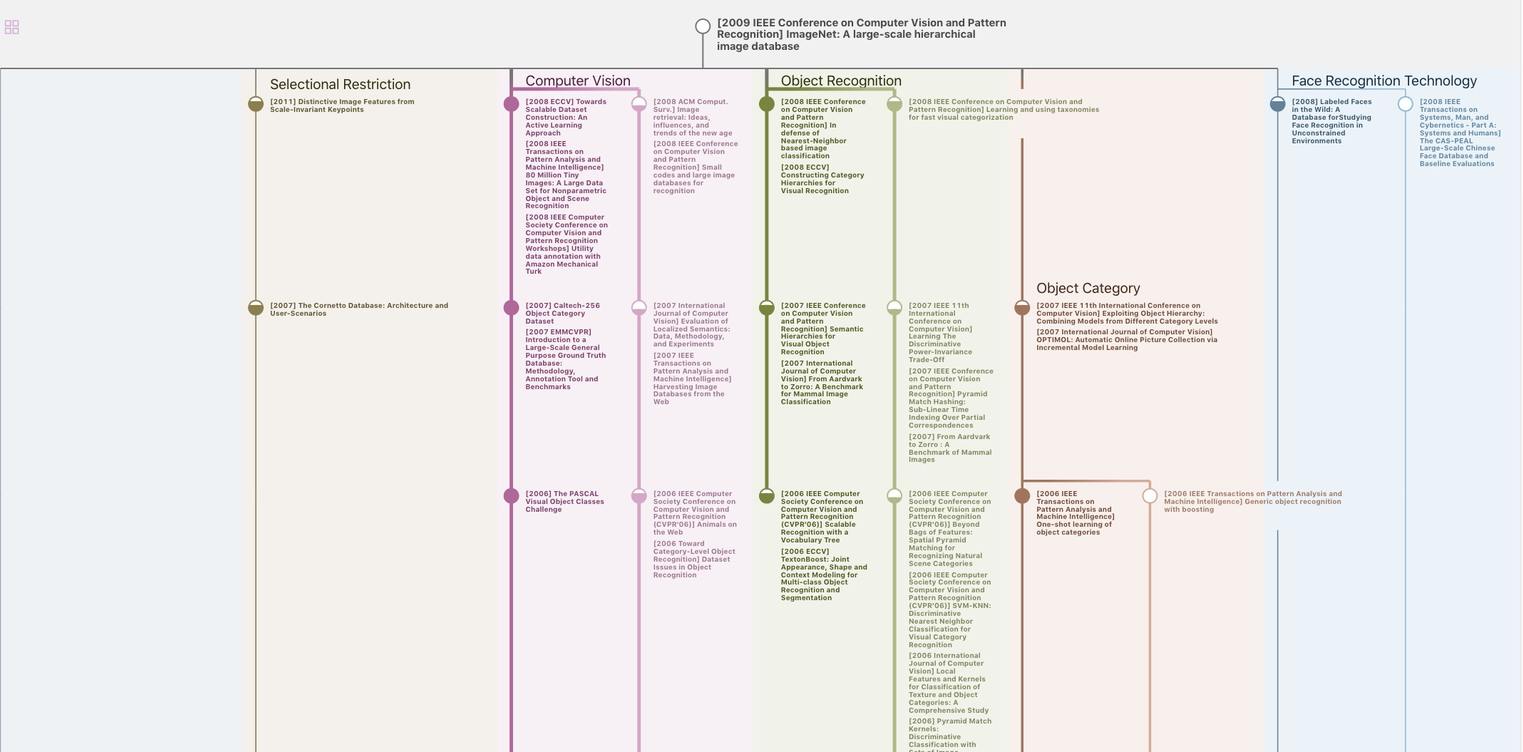
生成溯源树,研究论文发展脉络
Chat Paper
正在生成论文摘要