SampleHST-X: A Point and Collective Anomaly-Aware Trace Sampling Pipeline with Approximate Half Space Trees
Journal of Network and Systems Management(2024)
摘要
The storage requirement for distributed tracing can be reduced significantly by sampling only the anomalous or interesting traces that occur rarely at runtime. In this paper, we introduce an unsupervised sampling pipeline for distributed tracing that ensures high sampling accuracy while reducing the storage requirement. The proposed method, SampleHST-X, extends our recent work SampleHST. It operates based on a budget which limits the percentage of traces to be sampled while adjusting the storage quota of normal and anomalous traces depending on the size of this budget. The sampling process relies on accurately defining clusters of normal and anomalous traces by leveraging the distribution of mass scores, which characterize the probability of observing different traces, obtained from a forest of Half Space Trees (HST). In our experiments, using traces from a cloud data center, SampleHST yields 2.3 × to 9.5 × better sampling performance. SampleHST-X further extends the SampleHST approach by incorporating a novel class of Half Space Trees, namely Approximate HST, that uses approximate counters to update the mass scores. These counters significantly reduces the space requirement for HST while the sampling performance remains similar. In addition to this extension, SampleHST-X includes a Family of Graph Spectral Distances (FGSD) based trace characterization component, which, in addition to point anomalies, enables it to sample traces with collective anomalies. For such traces, we observe that the SampleHST-X approach can yield 1.2 × to 19 × better sampling performance.
更多查看译文
关键词
Distributed tracing,Microservices,Anomaly detection,Sampling
AI 理解论文
溯源树
样例
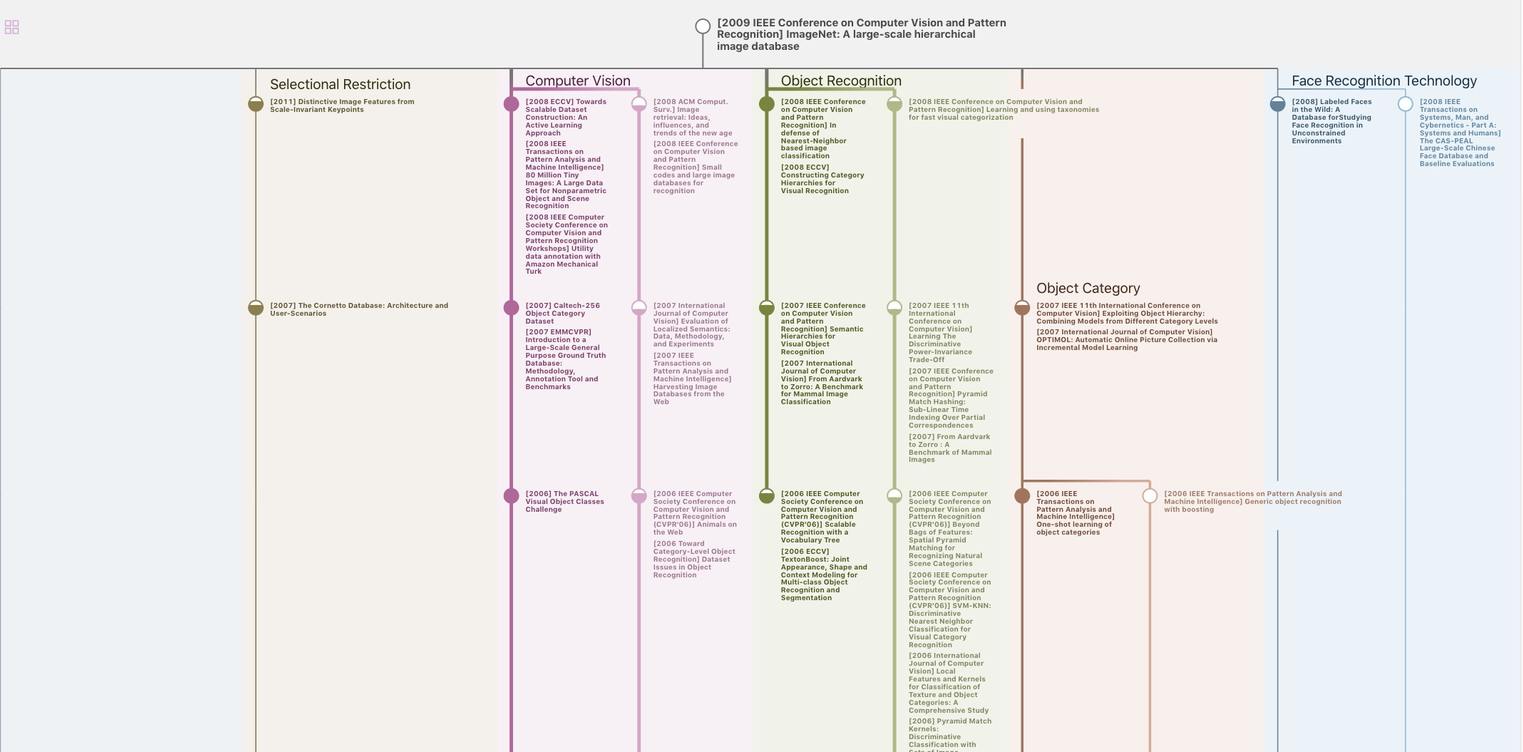
生成溯源树,研究论文发展脉络
Chat Paper
正在生成论文摘要