Robust remote sensing retrieval of key eutrophication indicators in coastal waters based on explainable machine learning
ISPRS Journal of Photogrammetry and Remote Sensing(2024)
摘要
Excessive discharges of nitrogen and phosphorus nutrients lead to eutrophication in coastal waters. Optical remote sensing retrieval of the key eutrophication indicators, namely dissolved inorganic nitrogen concentration (DIN), soluble reactive phosphate concentration (SRP), and chemical oxygen demand (COD), remains challenging due to lack of distinct spectral features. Although machine learning (ML) has shown the potential, the retrieval accuracy is limited, and the interpretability is insufficient in terms of the black-box characteristics. To address these limitations, based on robust and explainable ML algorithms, we constructed models for retrieving DIN, SRP, and COD over coastal waters of Northern South China Sea (NSCS), which is experiencing prominent eutrophication. Retrieval models based on classification and regression trees (CART) ML algorithms were developed using 4038 groups of in situ observations and quasi-synchronous satellite images. A comparison of CART algorithms, including Random Forest, Gradient Boosting Decision Tree, and eXtreme Gradient Boosting (XGBoost), indicated the highest retrieval accuracy of XGBoost for DIN (R2 = 0.88, MRE = 24.39 %), SRP (R2 = 0.92, MRE = 33.27 %), and COD (R2 = 0.75, MRE = 18.58 %) for validation dataset. On the basis of spectral remote sensing reflectance, further inputs of ocean physio-chemical properties, spatio-temporal information, and inherent optical properties may reduce retrieval errors by 30.16 %, 19.85 %, and 3.95 %, respectively, and their combined use reduced errors by 54.71 %. Besides, explainable ML analysis characterized the contribution of input features and enhanced the transparency of ML black-box models. Based on the proposed models, 27,278 satellite images and spatio-temporal reconstruction method, 1-km resolution gap-free daily DIN, SRP, and COD products were constructed from 2002 to 2022 for the coastal waters of NSCS. Under the influence of urbanization and river discharge, nitrogen and phosphorus concentrations in this area were found to have increased by 6.09 % and 11.04 %, respectively, over the past 21 years, with the fastest rise in the Pearl River Estuary, where the eutrophic water area had shown an increase rate of approximately 112.66 km2/yr. The proposed robust and explainable ML retrieval models may support ocean environment management and water quality monitoring by providing key eutrophication indicators products over coastal waters.
更多查看译文
关键词
Explainable machine learning,Water quality,Remote sensing,Eutrophication,Coastal waters
AI 理解论文
溯源树
样例
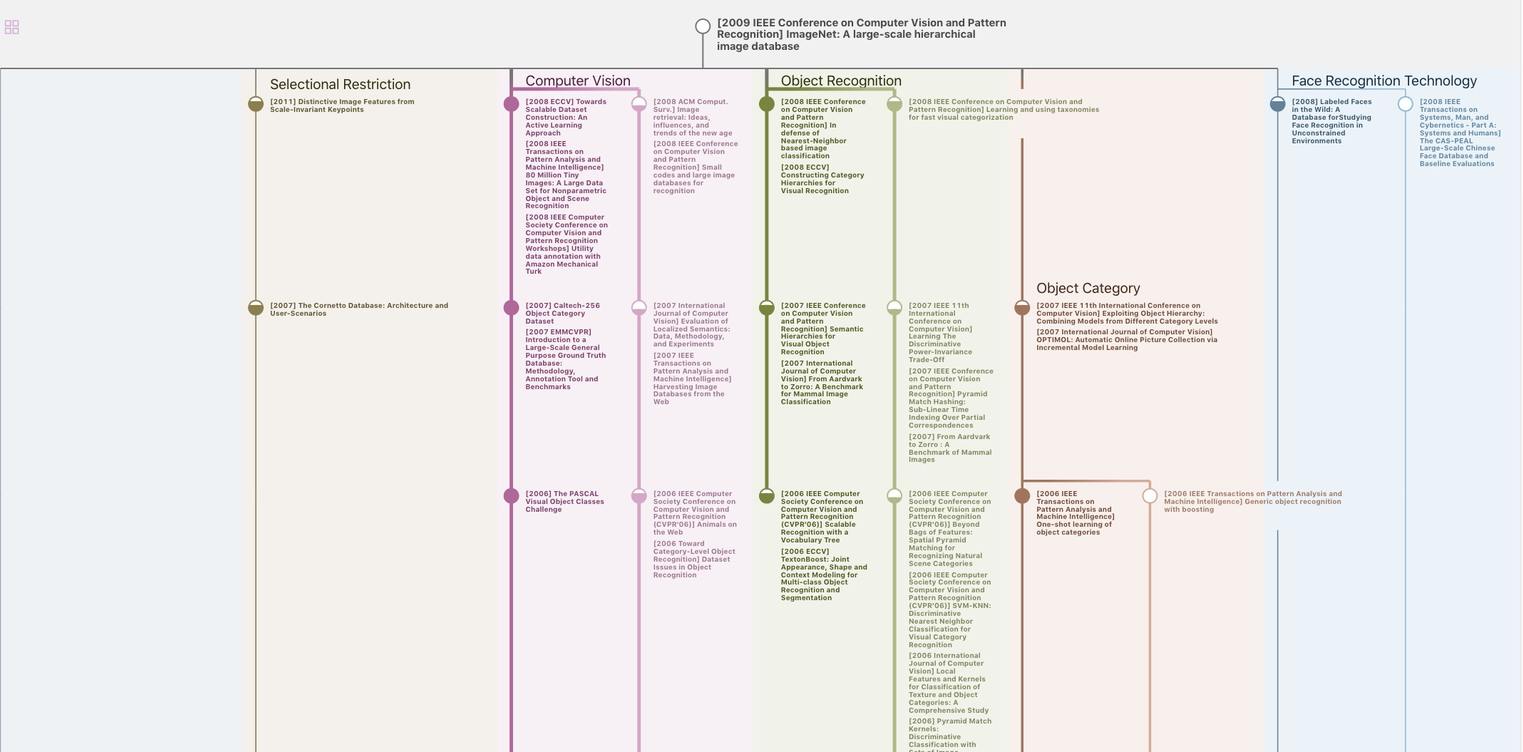
生成溯源树,研究论文发展脉络
Chat Paper
正在生成论文摘要