A new evolutionary strategy for reinforcement learning
Multimedia Tools and Applications(2024)
摘要
The detection of traffic signs in the natural scene requires a great deal of information due to the variations it undergoes over time for the output of the detection model to be effective and relevant. The amount of annotated data can range from a few hundred to thousands or even millions of examples, the availability of labeled data is often a critical bottleneck in the development and deployment of deep learning models, and acquiring high-quality annotations can be time-consuming and costly. Unlabeled data is easy to acquire but expensive to annotate. Several works focus on the use of active learning as an approach to get rid of the problem of costly annotation and computation time. To address these difficulties, we propose a Semi-Automatic Deep Image Annotation system using a new Evolutionary Strategy for Reinforcement Learning (SADIA-ESRL). Our experiments demonstrate remarkable efficiency on Natural Scene Traffic Sign and panel guide Arabic-Latin Text Dataset (NaSTSArLaT). The annotation approach studied has allowed for annotating only 1/4 of the images without compromising the model’s efficiency. This reduction in annotation effort is accompanied by significant time savings, with the labeling process now taking as little as 1/5 of the initial time. Furthermore, this strategy grants us the capability to selectively annotate instances, ensuring optimal performance in the used detection model.
更多查看译文
关键词
Annotation,Deep Reinforcement Learning,Traffic Sign,Text Detection,Object Detection,Genetic Algorithm
AI 理解论文
溯源树
样例
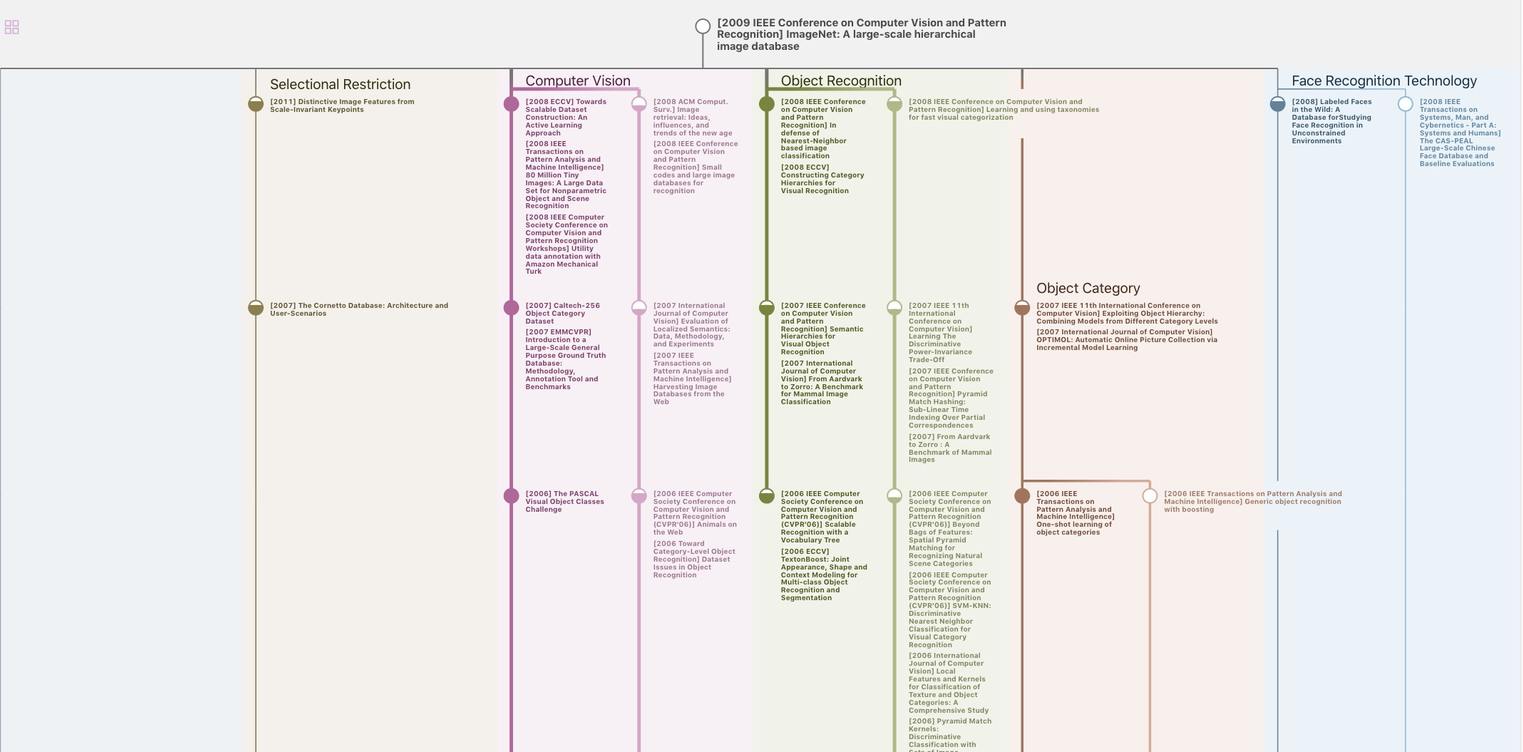
生成溯源树,研究论文发展脉络
Chat Paper
正在生成论文摘要