A Crystal-Plasticity-informed Gaussian Process Regression Model to Capture Anisotropy in Single Crystal Shape Memory Alloys
Computational materials science(2024)
摘要
This work presents a machine learning (ML) framework that model the anisotropic actuation responses in a shape memory alloy. A Gaussian Process Regression (GPR) based ML model is trained on a set of different crystal orientations subjected to different actuation conditions. The training employed thermo-mechanical responses from a crystal-plasticity model that captures phase-transformation, stress-induced plasticity, and transformation-induced plasticity. On training the GPR-ML model at fixed stress level for different orientations, it captured the thermo-mechanical responses accounting for the anisotropy, and predicted responses for new orientations with good accuracy. The GPR-ML model is able to capture the transformation temperature variations even when trained using multiple stress levels, and the transformation strain showed significant deviations. The developed GPR-ML model gave reasonable predictions for an unexplored sample set of orientations and loading conditions.
更多查看译文
关键词
HTSMA,Ni-Ti-Hf,Reduced order functions,Gaussian Process Regression,Phase-transformation
AI 理解论文
溯源树
样例
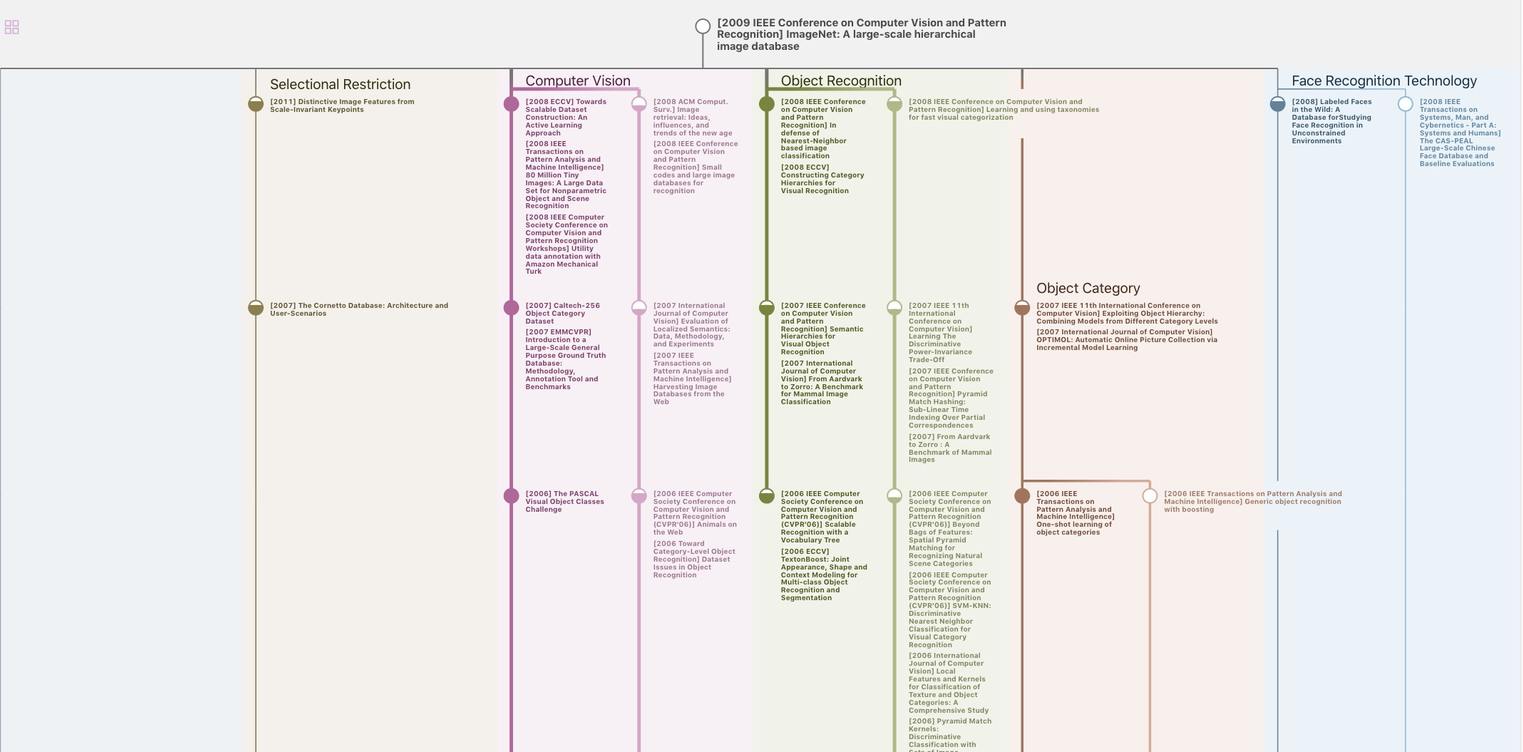
生成溯源树,研究论文发展脉络
Chat Paper
正在生成论文摘要