IMIL: Interactive Medical Image Learning Framework
arxiv(2024)
摘要
Data augmentations are widely used in training medical image deep learning
models to increase the diversity and size of sparse datasets. However, commonly
used augmentation techniques can result in loss of clinically relevant
information from medical images, leading to incorrect predictions at inference
time. We propose the Interactive Medical Image Learning (IMIL) framework, a
novel approach for improving the training of medical image analysis algorithms
that enables clinician-guided intermediate training data augmentations on
misprediction outliers, focusing the algorithm on relevant visual information.
To prevent the model from using irrelevant features during training, IMIL will
'blackout' clinician-designated irrelevant regions and replace the original
images with the augmented samples. This ensures that for originally
mispredicted samples, the algorithm subsequently attends only to relevant
regions and correctly correlates them with the respective diagnosis. We
validate the efficacy of IMIL using radiology residents and compare its
performance to state-of-the-art data augmentations. A 4.2
accuracy over ResNet-50 was observed when using IMIL on only 4
set. Our study demonstrates the utility of clinician-guided interactive
training to achieve meaningful data augmentations for medical image analysis
algorithms.
更多查看译文
AI 理解论文
溯源树
样例
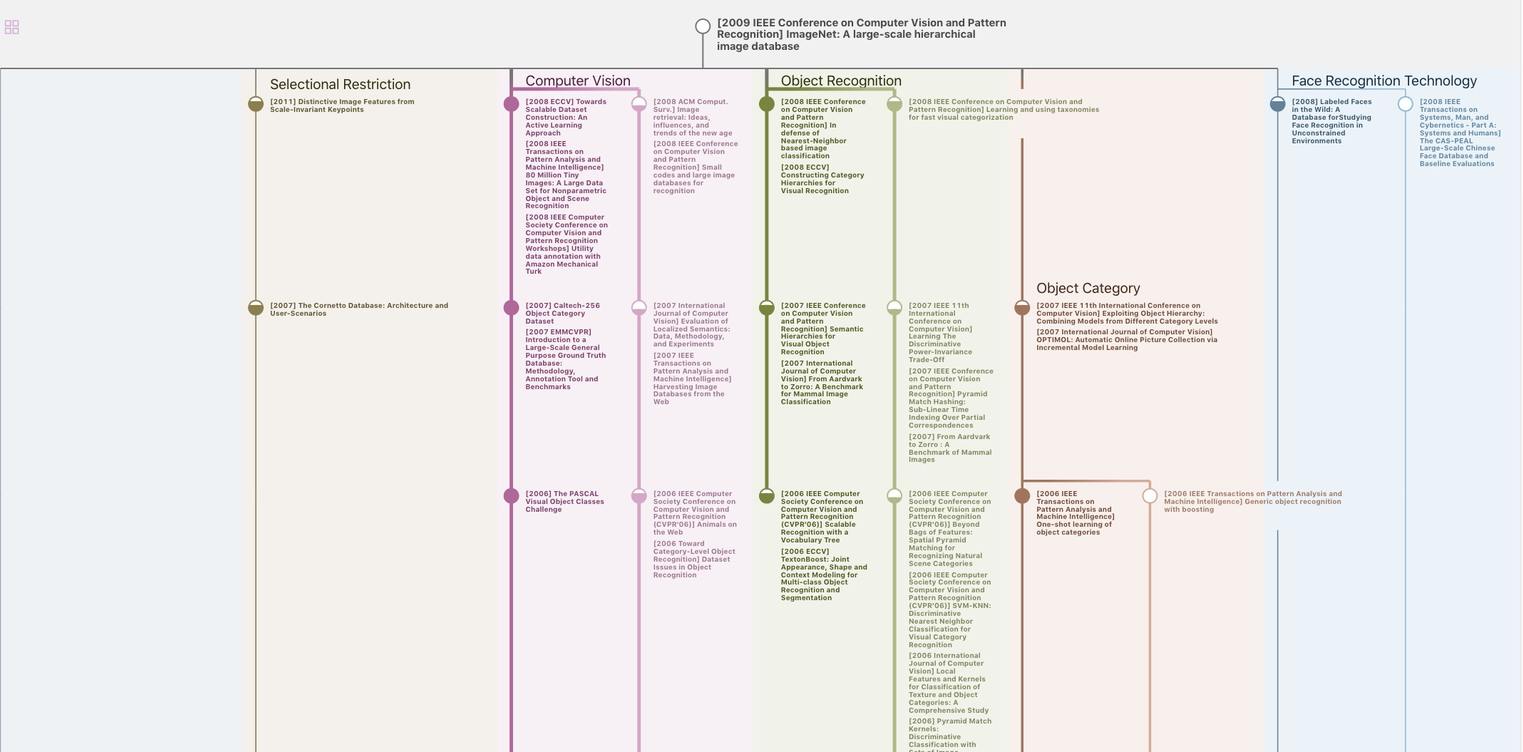
生成溯源树,研究论文发展脉络
Chat Paper
正在生成论文摘要