VARX Granger Analysis: Modeling, Inference, and Applications
arxiv(2024)
摘要
Vector Autoregressive models with exogenous input (VARX) provide a powerful
framework for modeling complex dynamical systems like brains, markets, or
societies. Their simplicity allows us to uncover linear effects between
endogenous and exogenous variables. The Granger formalism is naturally suited
for VARX models, but the connection between the two is not widely understood.
We aim to bridge this gap by providing both the basic equations and easy-to-use
code. We first explain how the Granger formalism can be combined with a VARX
model using deviance as a test statistic. We also present a bias correction for
the deviance in the case of L2 regularization, a technique used to reduce model
complexity. To address the challenge of modeling long responses, we propose the
use of basis functions, which further reduce parameter complexity. We
demonstrate that p-values are correctly estimated, even for short signals where
regularization influences the results. Additionally, we analyze the model's
performance under various scenarios where model assumptions are violated, such
as missing variables or indirect observations of the underlying dynamics.
Finally, we showcase the practical utility of our approach by applying it to
real-world data from neuroscience, physiology, and sociology. To facilitate its
adoption, we make Matlab, Python, and R code available here:
https://github.com/lcparra/varx
更多查看译文
AI 理解论文
溯源树
样例
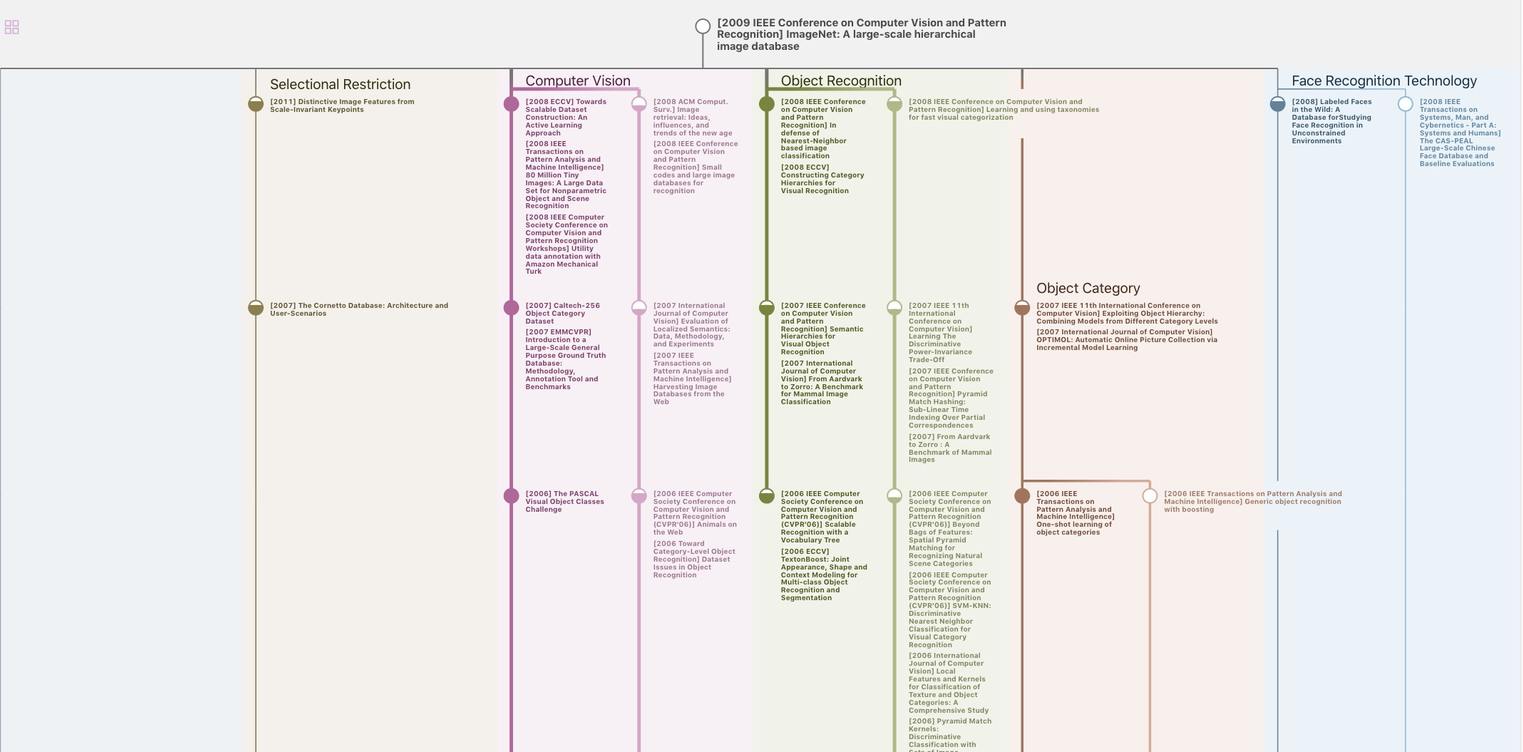
生成溯源树,研究论文发展脉络
Chat Paper
正在生成论文摘要