Runtime Analysis of Evolutionary Diversity Optimization on the Multi-objective (LeadingOnes, TrailingZeros) Problem
arxiv(2024)
摘要
The diversity optimization is the class of optimization problems, in which we
aim at finding a diverse set of good solutions. One of the frequently used
approaches to solve such problems is to use evolutionary algorithms which
evolve a desired diverse population. This approach is called evolutionary
diversity optimization (EDO).
In this paper, we analyse EDO on a 3-objective function LOTZ_k, which is a
modification of the 2-objective benchmark function (LeadingOnes,
TrailingZeros). We prove that the GSEMO computes a set of all Pareto-optimal
solutions in O(kn^3) expected iterations. We also analyze the runtime of the
GSEMO_D (a modification of the GSEMO for diversity optimization) until it
finds a population with the best possible diversity for two different diversity
measures, the total imbalance and the sorted imbalances vector. For the first
measure we show that the GSEMO_D optimizes it asymptotically faster than it
finds a Pareto-optimal population, in O(kn^2log(n)) expected iterations, and
for the second measure we show an upper bound of O(k^2n^3log(n)) expected
iterations. We complement our theoretical analysis with an empirical study,
which shows a very similar behavior for both diversity measures that is close
to the theory predictions.
更多查看译文
AI 理解论文
溯源树
样例
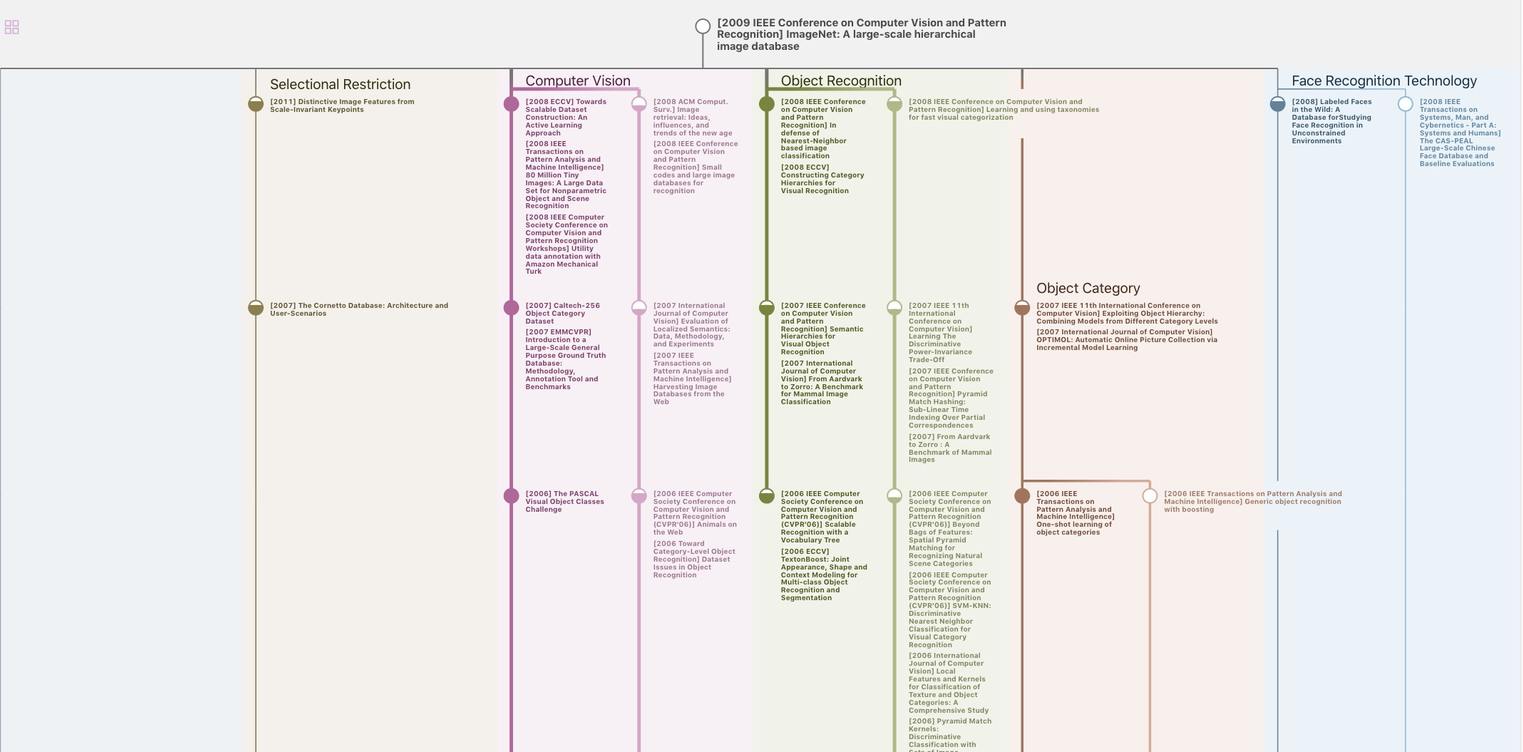
生成溯源树,研究论文发展脉络
Chat Paper
正在生成论文摘要