The combination of deep learning and pseudo-MS image improves the applicability of metabolomics to congenital heart defect prenatal screening
Talanta(2024)
摘要
To investigate the metabolic alterations in maternal individuals with fetal congenital heart disease (FCHD), establish the FCHD diagnostic models, and assess the performance of these models, we recruited two batches of pregnant women. By metabolomics analysis using Ultra High-performance Liquid Chromatography-Mass/Mass (UPLC-MS/MS), a total of 36 significantly altered metabolites (VIP >1.0) were identified between FCHD and non-FCHD groups. Two logistic regression models and four support vector machine (SVM) models exhibited strong performance and clinical utility in the training set (area under the curve (AUC) =1.00). The convolutional neural network (CNN) model also demonstrated commendable performance and clinical utility (AUC=0.89 in the training set). Notably, in the validation set, the performance of the CNN model (AUC=0.66, precision = 0.714) exhibited better robustness than the six models above (AUC≤0.50). In conclusion, the CNN model based on pseudo-MS images holds promise for real-world and clinical applications due to its better repeatability.
更多查看译文
关键词
Congenital heart disease,Convolutional neural networks,Diagnostic model,Metabolomics
AI 理解论文
溯源树
样例
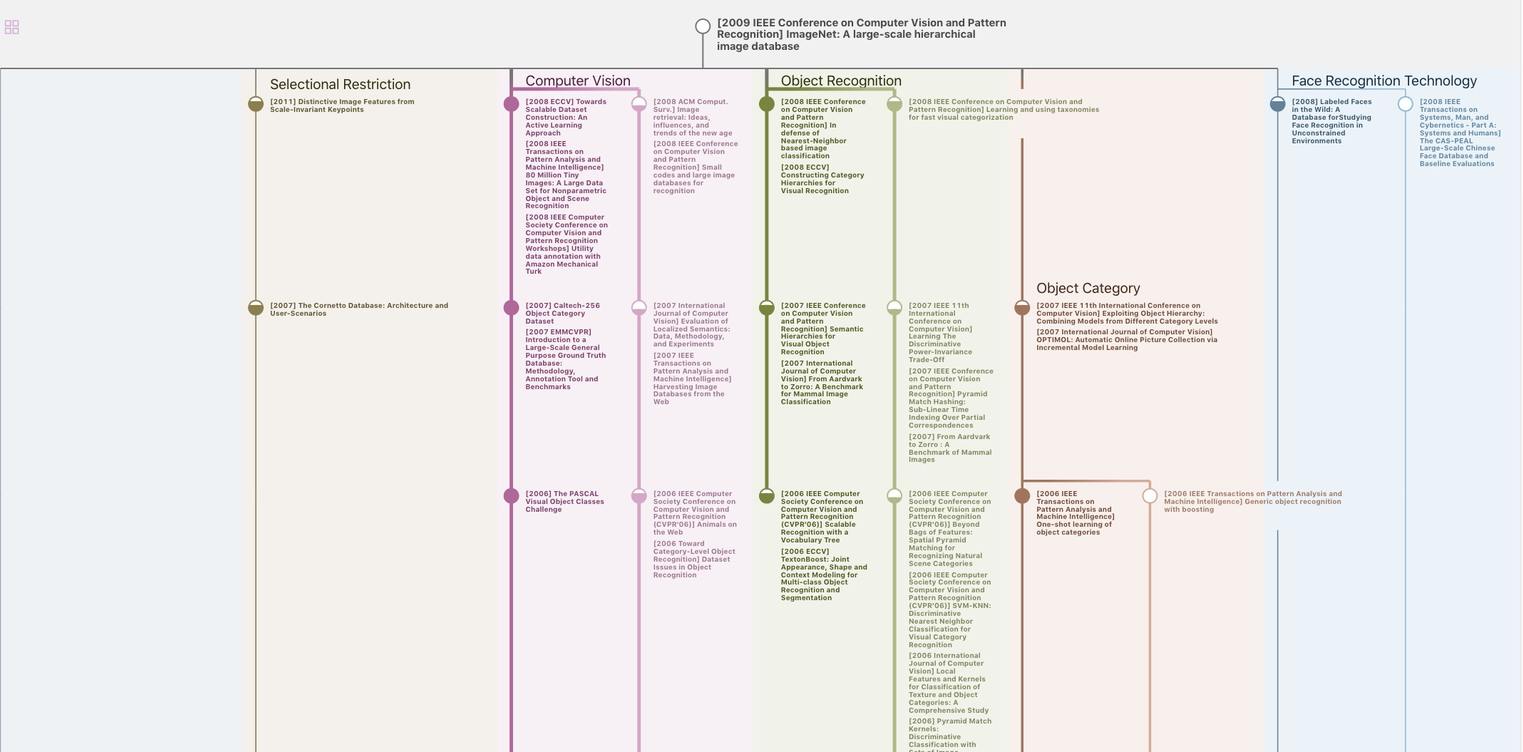
生成溯源树,研究论文发展脉络
Chat Paper
正在生成论文摘要