Efficient Text Detection Via Re-parameterization and Tversky Coefficientefficient and Flexible Text Detection
Electronic Information Technology and Computer Engineering(2023)
摘要
Currently, scene text detection models commonly suffer from a high precision but low recall issue. In other words, the false negative rate is much higher than the false positive rate, which limits the overall performance improvement of the models. This situation may lead to a series of issues such as missing important information. We propose an efficient and flexible text detection model to address the diversity of challenges in various usage scenarios. First of all, in order to meet the ever-increasing real-time needs while keeping its performance as unaffected as possible, we introduced the idea of structural re-parameterization, using parameter conversion to decouple training and inference, and realized multi-branch training and single-branch inference. In order to fuse multi-scale features efficiently and losslessly, we design a lightweight sub-pixel convolutional upsampling unit (LSUN) and an attention feature fusion module (AFM). LSUN will gradually adjust according to the feedback of the loss, and can learn the upsampling mode and feature expression suitable for specific tasks to produce more accurate and detailed upsampling results. AFM enables the network to selectively emphasize important features and suppress unimportant ones. Focal-TverskyLoss controls the punishment of the model for false positive samples(FPs) and false negative samples(FNs) through hyperparameters, and adjusts the penalty to drive the self-improvement of the model, balancing precision and recall.
更多查看译文
AI 理解论文
溯源树
样例
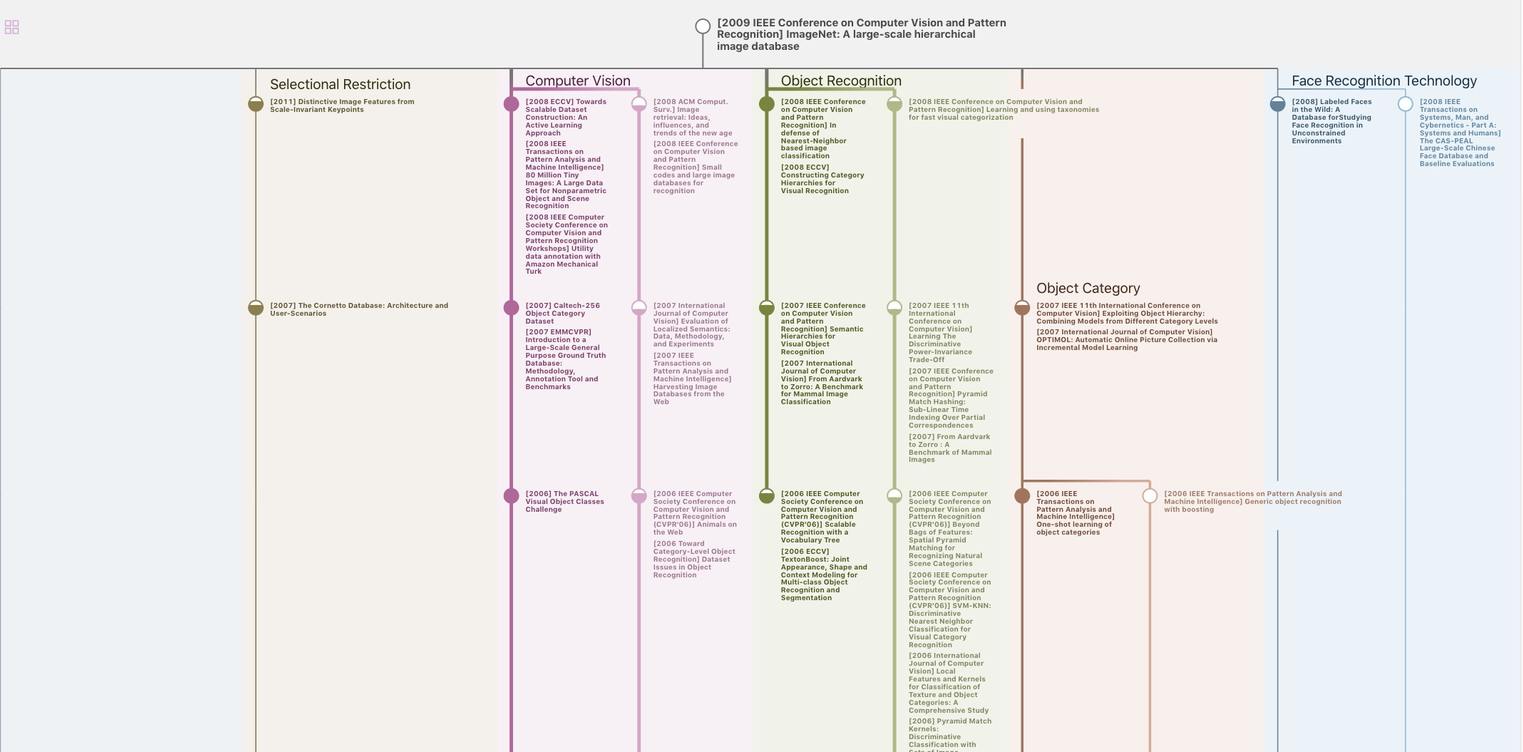
生成溯源树,研究论文发展脉络
Chat Paper
正在生成论文摘要