An End-to-End Predict-Then-Optimize Clustering Method for Stochastic Assignment Problems
IEEE Transactions on Intelligent Transportation Systems(2024)
摘要
Express pickup and delivery systems play crucial roles in contemporary urban areas. Couriers within these systems retrieve packages from designated Areas of Interest (AOI) that the express company assigns to them during specific time intervals. The express company traditionally employs historical pickup request data for executing AOI assignments (or pickup request assignments) for couriers, and these assignments are conventionally static and do not evolve over time However, future pickup requests display significant temporal variations. Employing historical data for future assignments is, therefore, somewhat impractical. Furthermore, even if we were to predict future pickup requests beforehand and subsequently employ these predictions for assignments, this two-stage approach proves to be both impractical and trivial, potentially harboring drawbacks. For example, the better prediction results may not necessarily guarantee better clustering outcomes. To address these challenges, we introduce an intelligent end-to-end predict-then-optimize clustering method that simultaneously forecasts future pickup requests for AOIs and dynamically allocates AOIs to couriers through clustering. Initially, we propose a deep learning-based prediction model for predicting order quantities within AOIs. Subsequently, we present a differential constrained
K
-means clustering method for AOI clustering based on the prediction results. Finally, we introduce a one-stage end-to-end predict-then-optimize clustering approach for the rational, dynamic, and intelligent allocation of AOIs to couriers. Our results demonstrate that this one-stage predict-then-optimize method significantly enhances optimization outcomes, namely the quality of clustering results. This study offers valuable insights that are relevant to predict-then-optimize-related tasks, particularly when addressing stochastic assignment problems within all types of express systems.
更多查看译文
关键词
Predict then optimize,order prediction,areas of interest clustering,express pickup,delivery systems
AI 理解论文
溯源树
样例
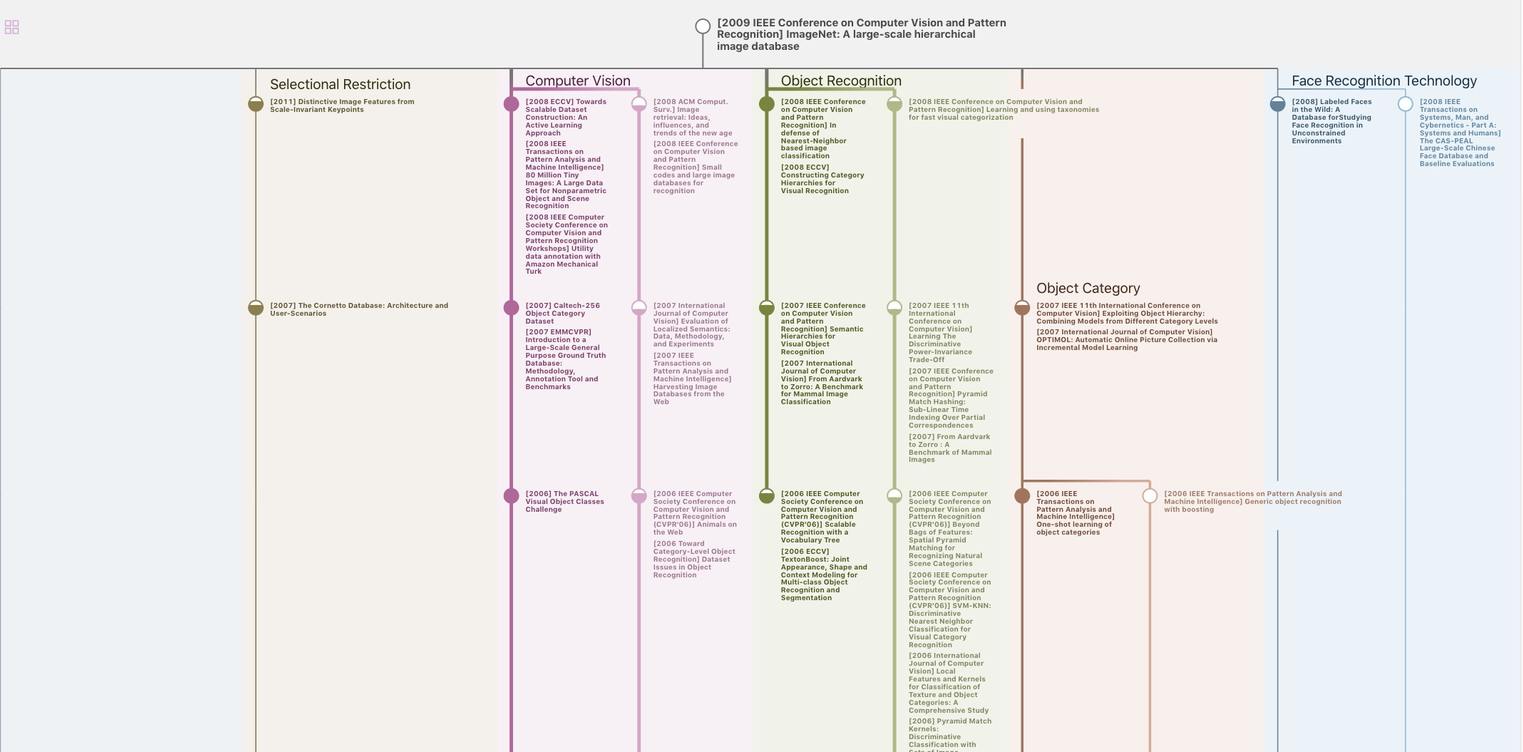
生成溯源树,研究论文发展脉络
Chat Paper
正在生成论文摘要