Ensemble Learning Approach for Prediction of Early Complications after Radiotherapy for Head and Neck Cancer using CT and MRI Radiomic Features
crossref(2024)
摘要
Abstract There are different side effects in radiotherapy of head and neck cancer (HNC) including xerostomia. The present study utilizes the addition of Magnetic Resonance (MR) radiomic image features to typical Computed Tomography (CT)-based features and radiation dose-based characteristics and incorporates the evaluation and validation of individual and ensemble classifiers for prediction of early-onset xerostomia in radiotherapy of HNC. A total of 80 patients diagnosed with HNC were evaluated prospectively. MR and CT imaging, dosimetric, and demographic features of patients were used as model input data. Bilateral parotid radiomic features were extracted from CT, T 1 weighted, and T 2 weighted MR images. Pearson statistical tests were used for selection of features and Random Tree (RT), Neural Network (NN), Linear Support Vector Machine (LSVM) and Bayesian Network (BN) classifiers were evaluated. The results suggest the extracted features from T 1 weighted images have superior prediction ability compared to T 2 weighted acquisitions. The RT and BN models based on T 1 weighted images show better performance than those obtained with T 2 weighted images. T 1 weighted image-based analysis shows area under the curve (AUC) values for The RT and BN models of 0.90 and 0.84, respectively, while corresponding values obtained from T 2 weighted images are 0.79 and 0.78 for RT and BN models respectively. Combined T 1 weighted image-based models RT-BN, RT-LSVM-BN and RT-NN-LSVM-BN also show good performance having AUC values 0.97, 0.92, and 0.90, respectively. These results show that radiomic features from MR images obtained before radiotherapy can be used in addition to other metrics as personalized and unique biomarkers for prediction of early-onset xerostomia. Ensemble classifiers are more efficient than individual classifiers in prediction of early xerostomia.
更多查看译文
AI 理解论文
溯源树
样例
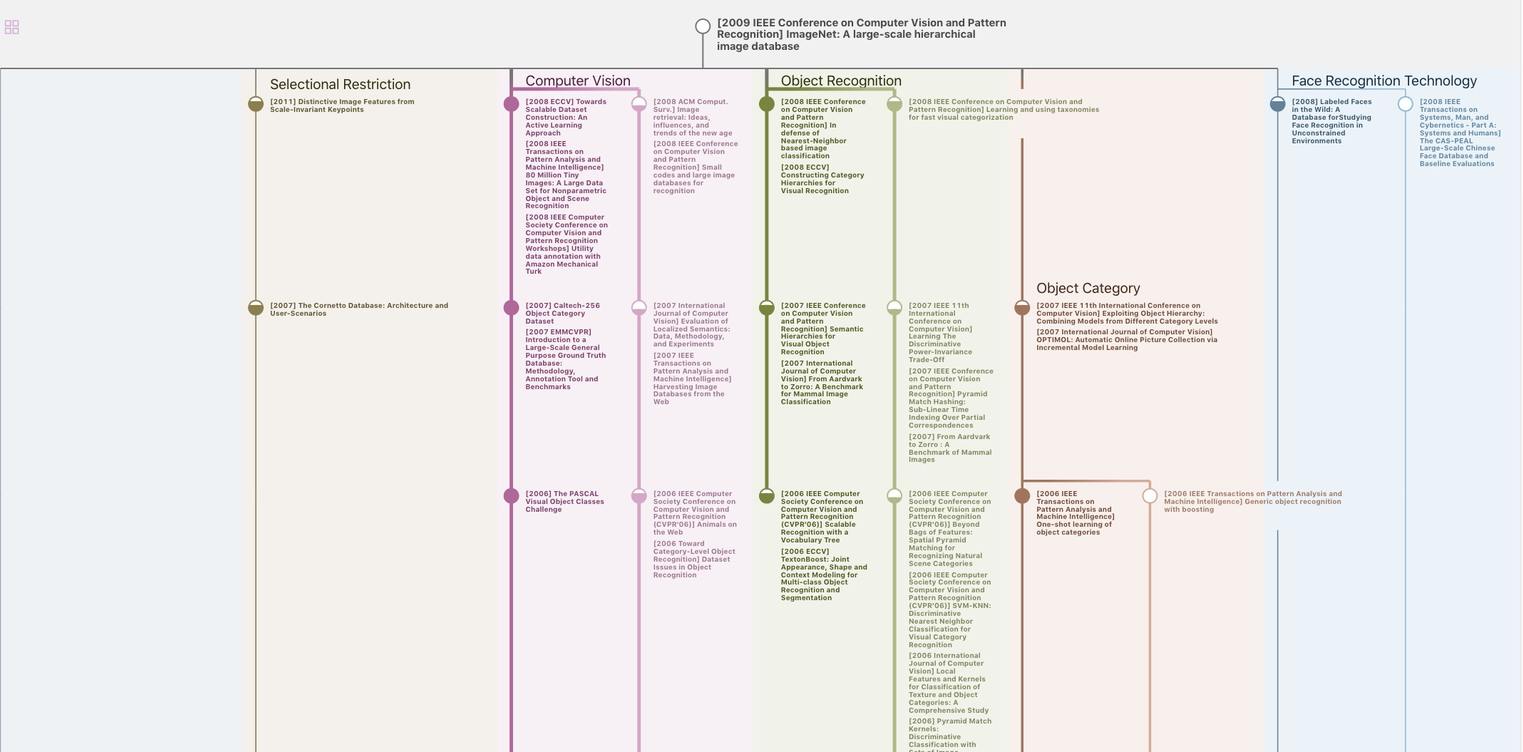
生成溯源树,研究论文发展脉络
Chat Paper
正在生成论文摘要