Machine Learning in Cardiac Stress Test Interpretation: A Systematic Review
European Heart Journal - Digital Health(2024)
摘要
Abstract Background Coronary artery disease (CAD) is a leading health challenge worldwide. Exercise stress testing is a foundational non-invasive diagnostic tool. Nonetheless, its variable accuracy prompts the exploration of more reliable methods. Recent advances in machine learning (ML), including deep learning (DL) and natural language processing (NLP), have shown potential in refining the interpretation of stress testing data. Methods Adhering to PRISMA guidelines, we conducted a systematic review of ML applications in stress electrocardiogram (ECG) and stress echocardiography for CAD prognosis. MEDLINE, Web of Science, and the Cochrane Library were used as databases. We analysed the ML models, outcomes, and performance metrics. Results Overall, seven relevant studies were identified. ML application in stress ECGs resulted in sensitivity and specificity improvements. Some models achieved above 96% in both metrics and reducing false positives by up to 21%. In stress echocardiography, ML models demonstrated an increase in diagnostic precision. Some models achieved specificity and sensitivity rates of up to 92.7% and 84.4%, respectively. NLP applications enabled categorization of stress echocardiography reports, with accuracies nearing 98%. Limitations include a small, retrospective study pool and the exclusion of nuclear stress testing, due to its well-documented status. Conclusion This review indicates AI applications potential in refining CAD stress testing assessment. Further development for real-world use is warranted.
更多查看译文
AI 理解论文
溯源树
样例
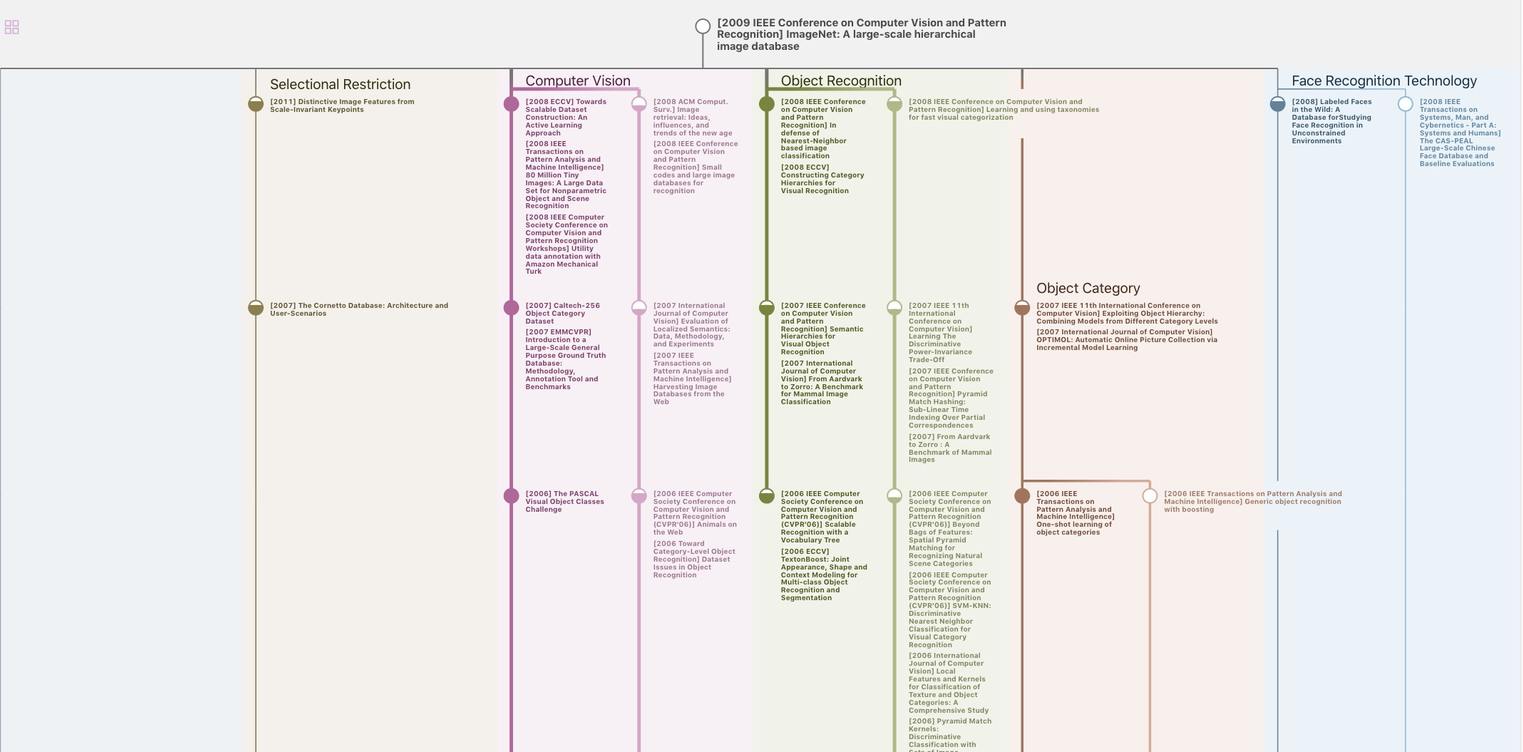
生成溯源树,研究论文发展脉络
Chat Paper
正在生成论文摘要