Developing ALERT, a Machine Learning Model to Identify Six-Month Mortality in Patients with Advanced Cancer
Journal of Pain and Symptom Management(2024)
摘要
Outcomes
1. Participants will be able to explain benefits and challenges of implementing machine learning predictive mortality tools.2. Participants will be able to suggest at least one recommendation for optimizing future acceptance and integration of the ALERT tool into practice.
Key Message
Machine learning driven predictive models have the potential to forecast mortality and guide care decisions for patients with solid cancers at the end of life. The identified potential benefits of the predictive machine learning tool included the ability to enhance quality of life at the end of life through removing uncertainty and allowing for opportunities to introduce goals of care.
Introduction
Machine learning driven predictive models have the potential to forecast mortality and guide care decisions for patients with solid cancers at the end of life.
Objectives
The purpose of this study was to seek input from patients and providers in order to develop and implement the ‘ALERT’ predictive model for patients with advanced solid cancers at high risk of death within the last 6-months of life.
Methods
We conducted one-on-one interviews with oncology physicians, social workers, nurses and patients with advanced cancer (n=24). Thematic analysis was done by a team of investigators to identify benefits, challenges, and recommendations.
Results
Benefits, challenges, and recommendations to guide the future implementation of ALERT were identified. Potential benefits of the tool included: 1) maximize quality of life at the end of life; 2) ease providers’ prognostication concerns in times of uncertainty. Challenges all revolved around potential for poor communication of the ALERT findings including: 1) method of provider communication of findings: need to balance standardization with flexibility to accommodate varying disease teams preferences; 2) inconsistency and/or insensitivity of communication to the patient and family; 3) anxiety and isolation that may result as a consequence of poor communication; 4) interdisciplinary power dynamics. Key recommendations included: 1) education to providers and patients regarding the science behind the algorithm; 2) create intentional, protected space and time to communicate prognostic findings; 3) ensure machine learning prognostication is synergistic with provider expertise.
Conclusions
Interdisciplinary participants support the future implementation of ALERT. Despite explicit benefits and support, ongoing evaluation is needed to ensure ALERT's benefits are maximized and challenges are overcome. As one participation stated, we need to ensure we “minimize the toxicity of the ALERT signal while ensuring therapeutic benefit”.
Keywords
Prognostication / Innovative Technologies
更多查看译文
AI 理解论文
溯源树
样例
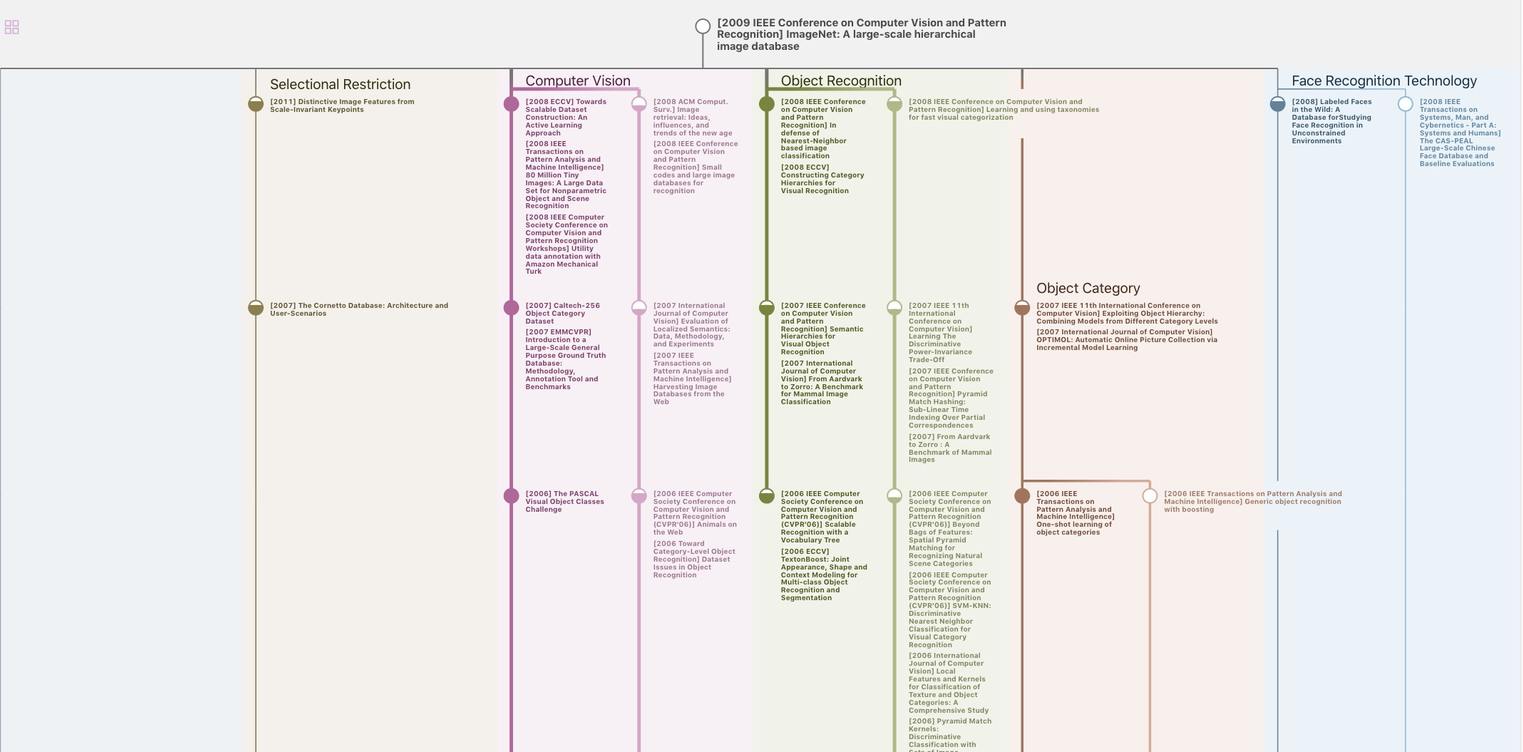
生成溯源树,研究论文发展脉络
Chat Paper
正在生成论文摘要