A novel, reduced-order optimization method for nonlinear model correction of turboshaft engines
Journal of Mechanical Science and Technology(2024)
摘要
Turboshaft engines are different from each other due to manufacturing and installation tolerances. Hence, it is difficult to draw out the physical model from the average component maps and design points to represent the performance of the individual engine. The available test-bed data is usually less than the number of correction coefficients to update the maps, and it is the underdetermined state optimization issue. In this paper, we propose a novel reduced-order optimization, namely PSO-EKF algorithm, combined with prior state estimation for non-linear model correction of the turboshaft engine. This method combines the advantages of PSO and extended Kalman filter (EKF). PSO-EKF method converts partial parameters optimized by PSO into parameters directly solved by EKF. The order of optimization space is reduced. Using the correction coefficient function, a stability improvement strategy is designed to ensure the stability of the optimization process. Compared with the GA and PSO algorithms, experimental verification shows that the method has a faster convergence speed and smaller model error than the general PSO. After the performance map is updated by this method, the error of outputs of the individual model is within 1.4
更多查看译文
关键词
Turboshaft engine,Component-level model,PSO algorithm,EKF,Model correction
AI 理解论文
溯源树
样例
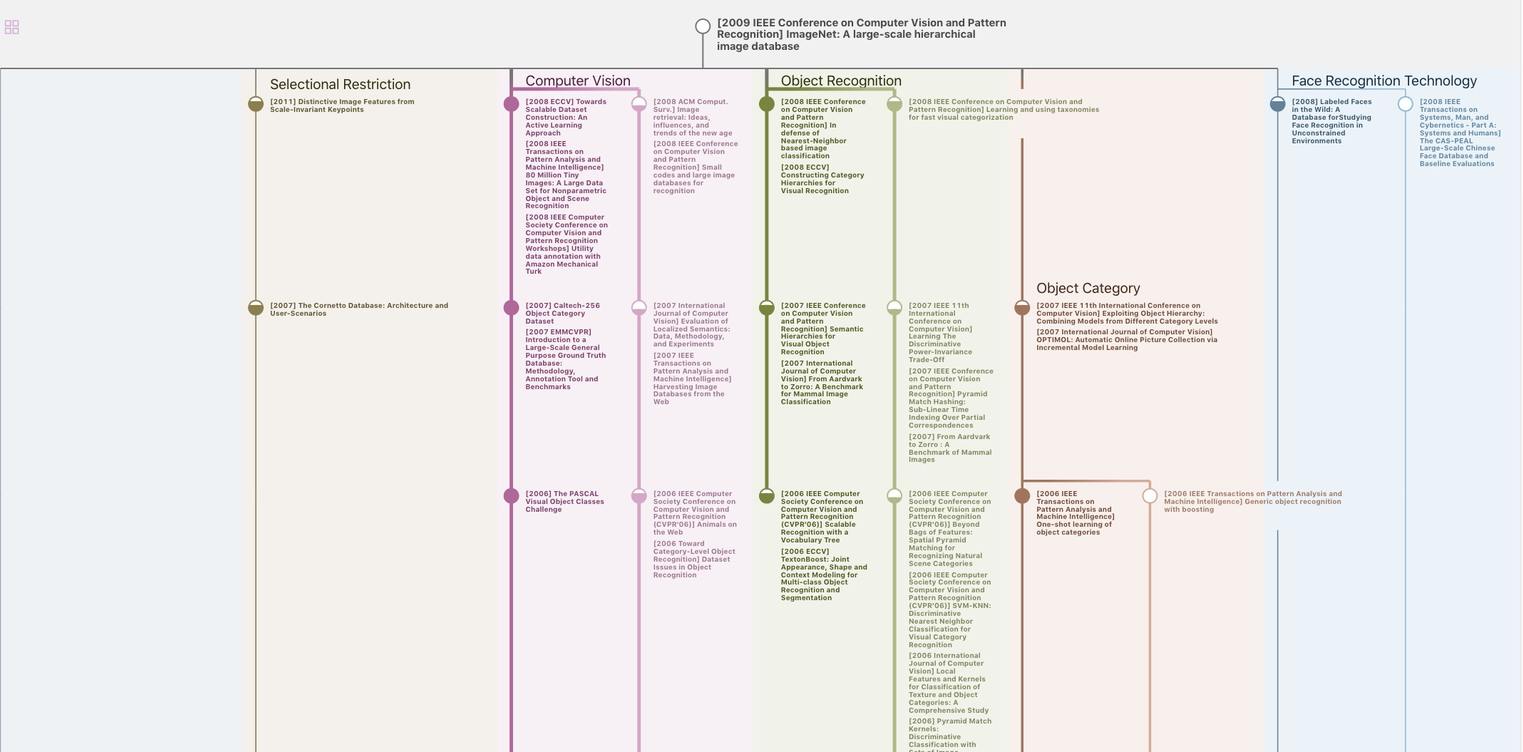
生成溯源树,研究论文发展脉络
Chat Paper
正在生成论文摘要