Deep neural network uncertainty estimation for early oral cancer diagnosis
JOURNAL OF ORAL PATHOLOGY & MEDICINE(2024)
摘要
BackgroundEarly diagnosis in oral cancer is essential to reduce both morbidity and mortality. This study explores the use of uncertainty estimation in deep learning for early oral cancer diagnosis.MethodsWe develop a Bayesian deep learning model termed 'Probabilistic HRNet', which utilizes the ensemble MC dropout method on HRNet. Additionally, two oral lesion datasets with distinct distributions are created. We conduct a retrospective study to assess the predictive performance and uncertainty of Probabilistic HRNet across these datasets.ResultsProbabilistic HRNet performs optimally on the In-domain test set, achieving an F1 score of 95.3% and an AUC of 96.9% by excluding the top 30% high-uncertainty samples. For evaluations on the Domain-shift test set, the results show an F1 score of 64.9% and an AUC of 80.3%. After excluding 30% of the high-uncertainty samples, these metrics improve to an F1 score of 74.4% and an AUC of 85.6%.ConclusionRedirecting samples with high uncertainty to experts for subsequent diagnosis significantly decreases the rates of misdiagnosis, which highlights that uncertainty estimation is vital to ensure safe decision making for computer-aided early oral cancer diagnosis.
更多查看译文
关键词
deep neural network,disease prediction,oral cancer,oral potentially malignant disorders,uncertainty estimation
AI 理解论文
溯源树
样例
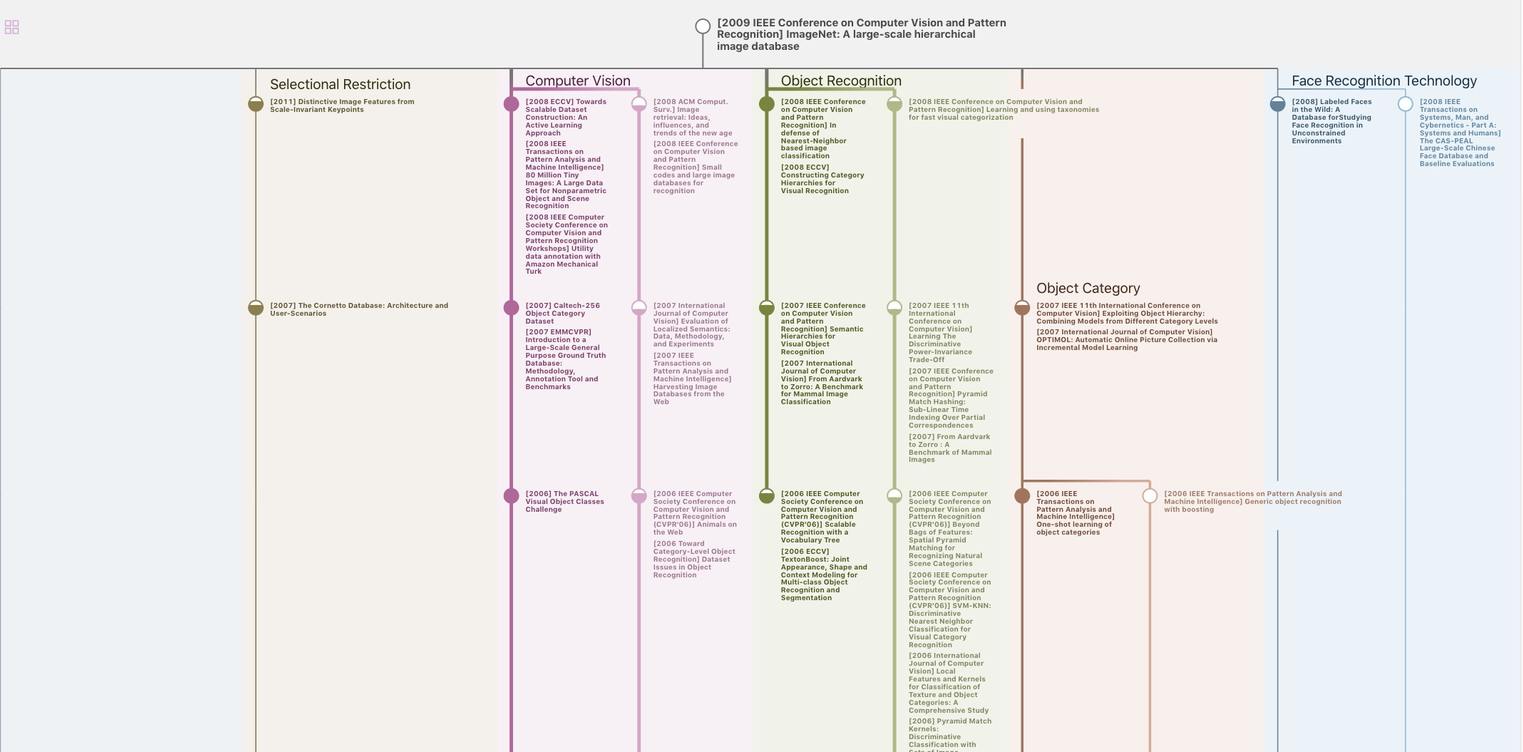
生成溯源树,研究论文发展脉络
Chat Paper
正在生成论文摘要