Deep-PK: Deep Learning for Small Molecule Pharmacokinetic and Toxicity Prediction.
Nucleic acids research(2024)
摘要
Evaluating pharmacokinetic properties of small molecules is considered a key feature in most drug development and high-throughput screening processes. Generally, pharmacokinetics, which represent the fate of drugs in the human body, are described from four perspectives: absorption, distribution, metabolism and excretion-all of which are closely related to a fifth perspective, toxicity (ADMET). Since obtaining ADMET data from in vitro, in vivo or pre-clinical stages is time consuming and expensive, many efforts have been made to predict ADMET properties via computational approaches. However, the majority of available methods are limited in their ability to provide pharmacokinetics and toxicity for diverse targets, ensure good overall accuracy, and offer ease of use, interpretability and extensibility for further optimizations. Here, we introduce Deep-PK, a deep learning-based pharmacokinetic and toxicity prediction, analysis and optimization platform. We applied graph neural networks and graph-based signatures as a graph-level feature to yield the best predictive performance across 73 endpoints, including 64 ADMET and 9 general properties. With these powerful models, Deep-PK supports molecular optimization and interpretation, aiding users in optimizing and understanding pharmacokinetics and toxicity for given input molecules. The Deep-PK is freely available at https://biosig.lab.uq.edu.au/deeppk/. Graphical Abstract
更多查看译文
关键词
Pharmacokinetics,Network Pharmacology,Drug Target Identification,Molecular Docking,Polypharmacology
AI 理解论文
溯源树
样例
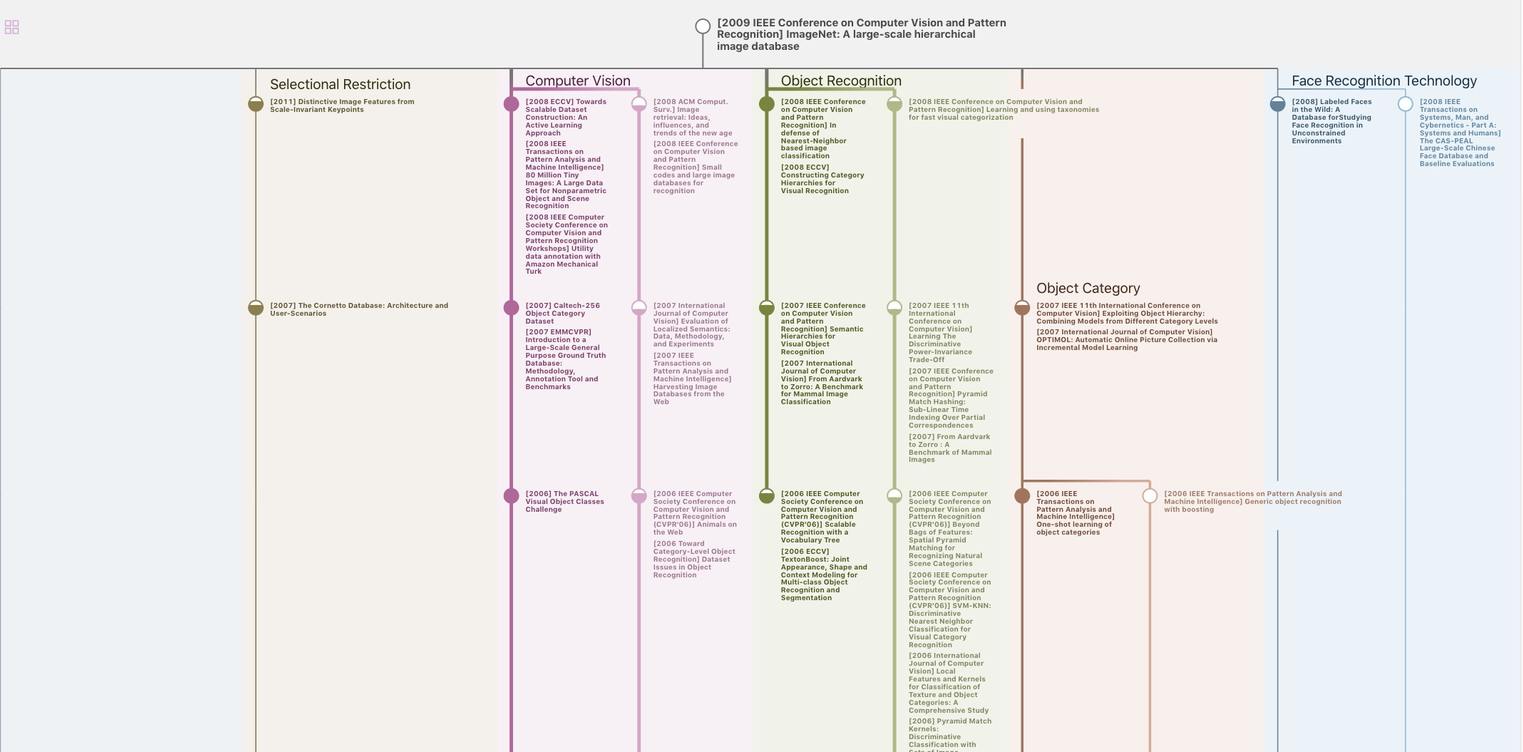
生成溯源树,研究论文发展脉络
Chat Paper
正在生成论文摘要