Cross Model Validation for a Diversified Cropping System
European journal of agronomy(2024)
摘要
Crop diversification is gaining traction due to the positive benefits in the delivery of ecosystem services (ESS) and the promotion of biodiversity. Agroecosystem simulation models can contribute to the design of diversified cropping systems but require calibration and validation before they can be applied. However, data availability is still very limited, particularly for diversified cropping systems. Therefore, the main goal of this study was to evaluate the suitability of the Nelder-Mead optimization method and the leave-one-out (LOO) validation method to calibrate and validate a diversified cropping system with a limited dataset, by using either a fixed year combination for calibration and validation for all crops or using a flexible year combination for every crop. Crop phenology was manually calibrated for all year combinations and the best parameter set based on the LOO-validation was selected for the subsequent step. Next, a four-parameter set related to crop growth and biomass dynamics was chosen for parameter optimization in the calibration step. To measure model performance during both steps, the root mean square error (RMSE) in days was used for phenology and a weighed relative RMSE (RRMSE) was used for crop growth, with the intermediate and final biomass contributing to 50% of the error and the other 50% corresponding to grain yield. Data for model comparison was collected at the patchCROP landscape experiment in Brandenburg, Germany. Observed data included daily weather, soil information, crop phenology, intermediate and final above ground biomass and grain yield for summer seasons 2020, 2021, and 2022 and winter seasons 2020/2021 and 2021/2022 (referred as 2021 and 2022, respectively). Summer crops included maize, soybean, lupine and sunflower, while winter crops were wheat, barley, rye and rapeseed. Results showed that the Nelder-Mead method was successful in reducing the error between observed and simulated data. As for the LOO-validation, the method showed that different year combinations led to a similar RMSE for phenology. However, for crop growth, optimum year combination was critical, as it differed for all summer crops but not for winter crops. For the summer crops, the lowest errors in the LOO-validation were observed in lupine, maize and soybean, with <20.6% RRMSE, while sunflower resulted in a reasonable LOO-validated value with 31.2% RRMSE, but a poor performance in the calibration step with 68.7% RRMSE. For the winter crops, the 2022 calibration year and the 2021 validation year combination resulted in the lowest RRMSE for wheat, barley and rapeseed. However, for rye, both year combinations led to a large error, with the lowest error when using the 2021 season for calibration (65.9% RRMSE) and 2022 season for validation (33.0% RRMSE). The flexible LOO-validation method was useful to make optimal use of the limited dataset as it allowed a more through model testing and pointed to differences among summer and winter crops. The newly validated model has the potential to be used for the design of diversified cropping systems.
更多查看译文
关键词
Nelder-Mead method,LOO-validation,Process-based crop model,Crop model,Model calibration
AI 理解论文
溯源树
样例
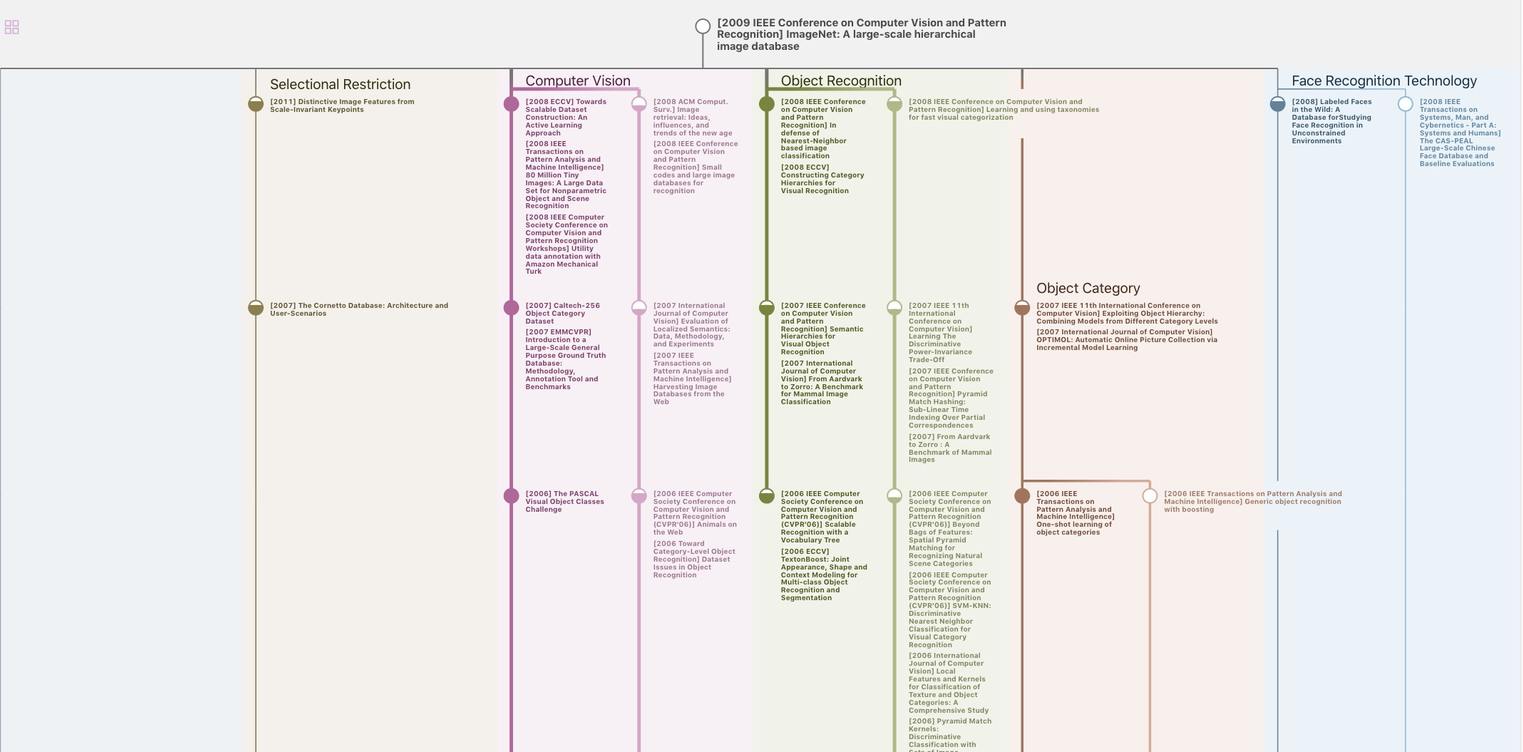
生成溯源树,研究论文发展脉络
Chat Paper
正在生成论文摘要