Modelling Stochastic and Quasi-Periodic Behaviour in Stellar Time-Series: Gaussian Process Regression Versus Power-Spectrum Fitting
MONTHLY NOTICES OF THE ROYAL ASTRONOMICAL SOCIETY(2024)
摘要
As the hunt for an Earth-like exoplanets has intensified in recent years, sohas the effort to characterise and model the stellar signals that can hide ormimic small planetary signals. Stellar variability arises from a number ofsources, including granulation, supergranulation, oscillations and activity,all of which result in quasi-periodic or stochastic behaviour in photometricand/or radial velocity observations. Traditionally, the characterisation ofthese signals has mostly been done in the frequency domain. However, the recentdevelopment of scalable Gaussian process regression methods makes directtime-domain modelling of stochastic processes a feasible and arguablypreferable alternative, obviating the need to estimate the power spectraldensity of the data before modelling it. In this paper, we compare the twoapproaches using a series of experiments on simulated data. We show thatfrequency domain modelling can lead to inaccurate results, especially when thetime sampling is irregular. By contrast, Gaussian process regression resultsare often more precise, and systematically more accurate, in both the regularand irregular time sampling regimes. While this work was motivated by theanalysis of radial velocity and photometry observations of main sequence starsin the context of planet searches, we note that our results may also haveapplications for the study of other types of astrophysical variability such asquasi-periodic oscillations in X-ray binaries and active galactic nucleivariability.
更多查看译文
关键词
asteroseismology,methods: data analysis,techniques: radial velocities,Sun: granulation
AI 理解论文
溯源树
样例
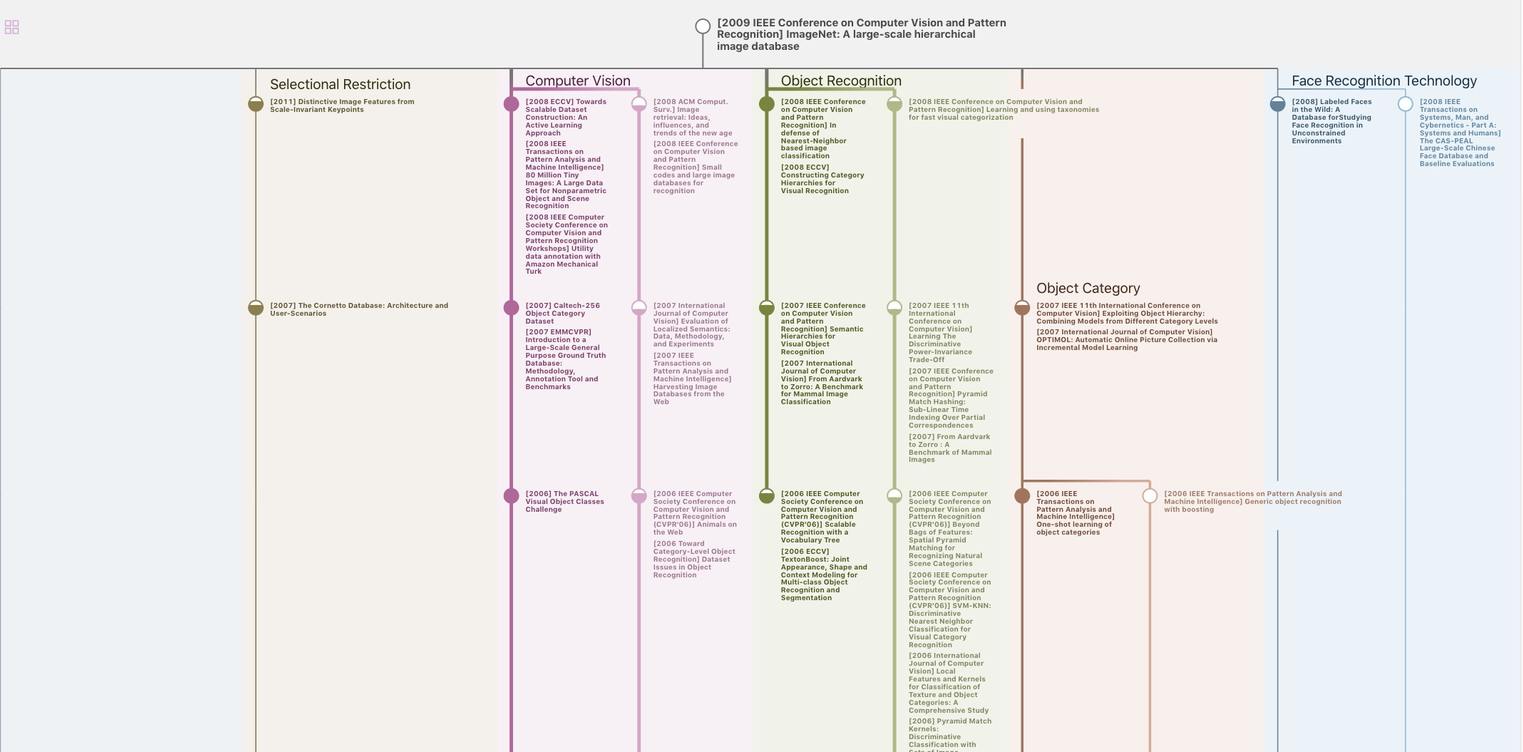
生成溯源树,研究论文发展脉络
Chat Paper
正在生成论文摘要