Tensor-Networks-based Learning of Probabilistic Cellular Automata Dynamics
arxiv(2024)
摘要
Algorithms developed to solve many-body quantum problems, like tensor
networks, can turn into powerful quantum-inspired tools to tackle problems in
the classical domain. In this work, we focus on matrix product operators, a
prominent numerical technique to study many-body quantum systems, especially in
one dimension. It has been previously shown that such a tool can be used for
classification, learning of deterministic sequence-to-sequence processes and of
generic quantum processes. We further develop a matrix product operator
algorithm to learn probabilistic sequence-to-sequence processes and apply this
algorithm to probabilistic cellular automata. This new approach can accurately
learn probabilistic cellular automata processes in different conditions, even
when the process is a probabilistic mixture of different chaotic rules. In
addition, we find that the ability to learn these dynamics is a function of the
bit-wise difference between the rules and whether one is much more likely than
the other.
更多查看译文
AI 理解论文
溯源树
样例
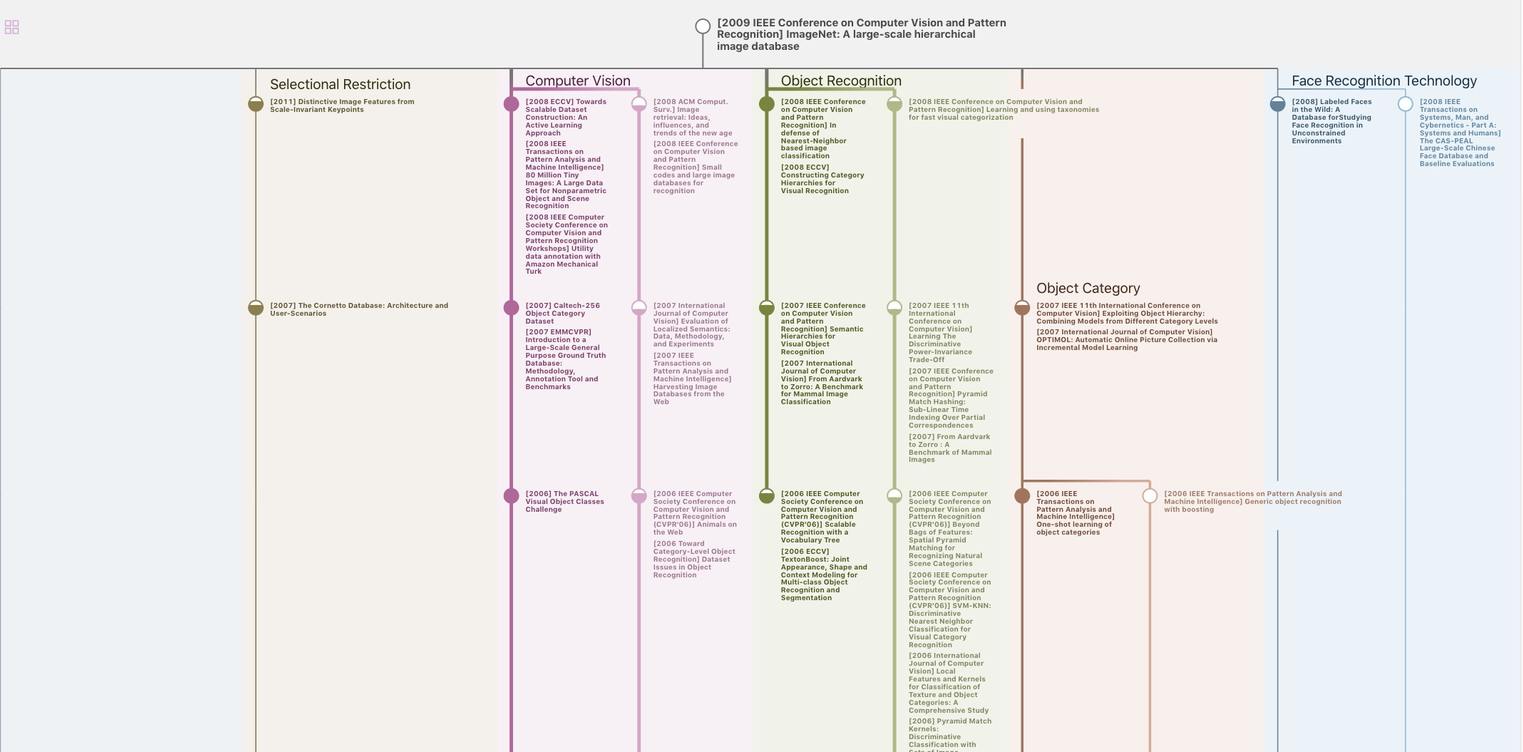
生成溯源树,研究论文发展脉络
Chat Paper
正在生成论文摘要