Multi-Agent Relative Investment Games in a Jump Diffusion Market with Deep Reinforcement Learning Algorithm
arxiv(2024)
摘要
This paper focuses on multi-agent stochastic differential games for
jump-diffusion systems. On one hand, we study the multi-agent game for optimal
investment in a jump-diffusion market. We derive constant Nash equilibria and
provide sufficient conditions for their existence and uniqueness for
exponential, power, and logarithmic utilities, respectively. On the other hand,
we introduce a computational framework based on the actor-critic method in deep
reinforcement learning to solve the stochastic control problem with jumps. We
extend this algorithm to address the multi-agent game with jumps and utilize
parallel computing to enhance computational efficiency. We present numerical
examples of the Merton problem with jumps, linear quadratic regulators, and the
optimal investment game under various settings to demonstrate the accuracy,
efficiency, and robustness of the proposed method. In particular, neural
network solutions numerically converge to the derived constant Nash equilibrium
for the multi-agent game.
更多查看译文
AI 理解论文
溯源树
样例
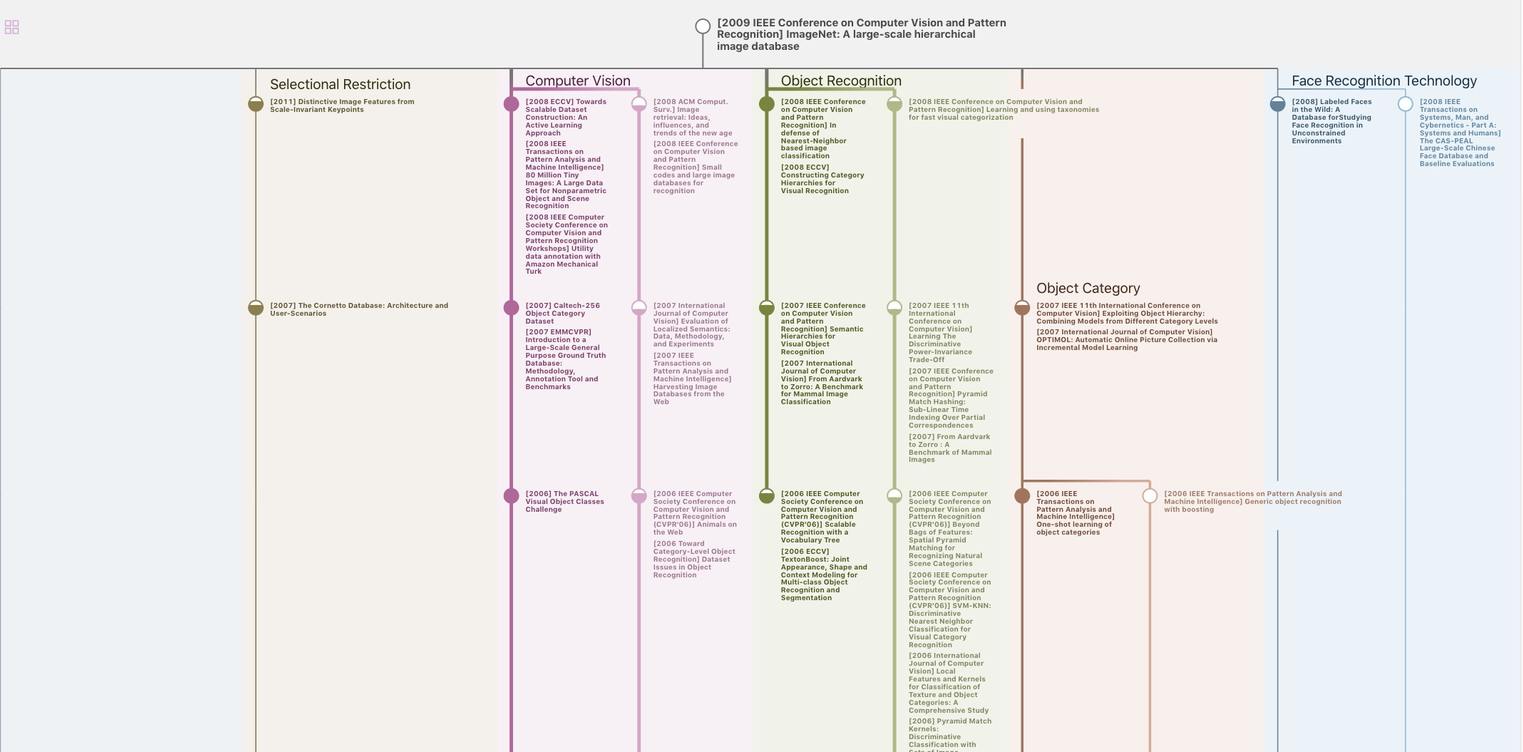
生成溯源树,研究论文发展脉络
Chat Paper
正在生成论文摘要